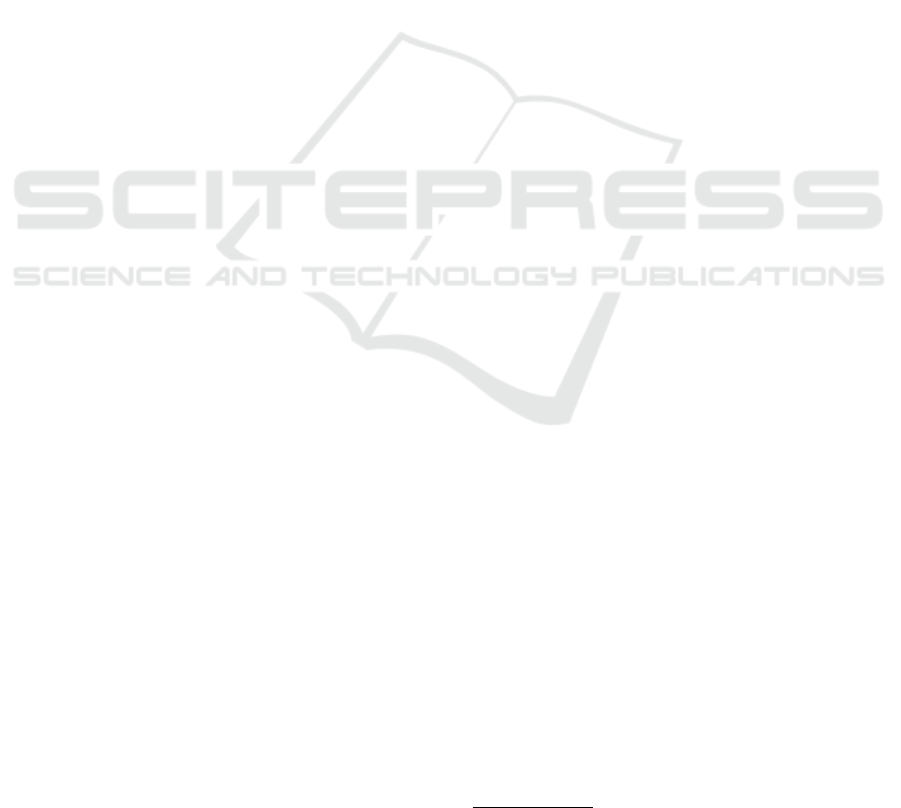
tremely hard to process since the sentence-final fell
is highly surprising and has thus a high information
value, thereby forming an information peak. UID,
therefore, seems to be an essential principle of lan-
guage processing. This study aims to check whether
the UID principle holds when extra-sentential con-
texts for calculating the information content of words
are considered. Formal definitions of UID (given in 6
and 7 below) consider both the variance of informa-
tion in messages, for example, in sentences, and the
change of information from sign to sign in messages,
for instance, from word to word in sentences (Collins,
2014; Jain et al., 2018). Our prediction for the eight
languages in focus is that the variance and informa-
tion change per word will be small on average and
per sentence.
In contrast to the assumption of the cross-
linguistically valid UID principle, we assume that
information derived from dependency structures is
language-dependent. Therefore we exploit corpora
from typologically different languages: the empirical
testing ground for this study is a convenience sam-
ple of the non-European languages Indonesian and
Arabic and some European languages from different
language subfamilies, i.e. Russian (Slavic), Span-
ish, French (Romance), Swedish and German (Ger-
manic). Including more than one language from the
same (sub)family allows us to see whether the Ro-
manic or the Germanic languages, respectively, be-
have similarly.
Shannon defines information as the likelihood of a
sign s (Shannon, 1948). Shannon Information (SI), in
bits, is the log-transformation of the sign’s probability
whereby s represents a sign, given equation 1:
SI(s) = −log
2
(P(s)) (1)
Intuitively, the number of bits corresponds to the
number of ‘yes/no’-questions to determine a possible
state in a probability space, and it is important to clar-
ify that information in Shannon’s theory is different
from the concept of ’information’ in linguistics and
also in everyday language use. Initially, the mean-
ing of messages was not of any interest for Shan-
non, since “[...] semantic aspects of communication
are irrelevant to the engineering problem[. . . ]” (Shan-
non and Weaver, 1949), i.e. for the optimal coding
and transmission of messages. In particular since
the seminal work of (Dretske, 1981), the relation-
ship between Shannon information and natural lan-
guage understanding came into focus (see for instance
(Resnik, 1995; Melamed, 1997; Bennett and Good-
man, 2018)). This is also important for our study,
since the UID deals with principles of language un-
derstanding. In surprisal theory (Hale, 2001), infor-
mation is derived from conditional probabilities, i.e.,
given a context. (Levy, 2008) equals information con-
tent of a sign with its surprisal, which, in turn, is
proportional to the processing effort it causes (Hale,
2001; Levy, 2008): the more surprising a sign s is,
that is to say, the smaller its probability is in a con-
text. The more informative s is, the higher the effort
is to process it. This relationship is given in 2:
di f f iculty ∝ surprisal (2)
This corresponds to Zipf’s law (Zipf, 2013),
which describes the negative correlation between fre-
quency and length of linguistic signs and, in addition,
the principle of least effort (Zipf, 1949): frequently
occurring signs tend to be short, rarely occurring ones
tend to be longer and tend to have higher information
content. (Levy, 2008) points out that in the Surprisal
Model model of language comprehension that em-
ploys information theory, large, extra-sentential con-
texts need to be considered to estimate a word’s infor-
mation content (IC). This is represented in 3 by the
variable CONTEXT:
SI(w
i
) = −log
2
P(w
i
|w
1
...w
i−1
, CONTEXT) (3)
However, (Levy, 2008) gives no clear definition
of what a context is. This makes the notion of extra-
sentential context somewhat challenging to grasp and
might explain that, to our best knowledge, there are
no studies on the calculation of information utilis-
ing large contexts. In this paper, we will explicitly
take up the idea of extra-sentential contexts by us-
ing dependency structures on the highest hierarchy-
level (directly below the verbal root-node) in sen-
tences that precede the target word and in the actual
sentence a target word occurs. Thereby we take up
an idea from (Levshina, 2017), who estimated lexi-
cal information from dependency structures. How-
ever, in contrast to Levshina, we consider complete
syntactic dependency patterns in sentences. We as-
sume that the languages in focus differ in terms of
their dependency structures: differences in the posi-
tion of subjects and objects are to be expected because
our set of languages contains both strongly inflected
and weakly inflected languages, with the former tend-
ing to have greater freedom in word position in the
sentence. Consequently, when deriving lexical in-
formation from language-specific dependency struc-
tures, there should be differences in the distribution
of information between the languages.
The present study uses the existing – partly quite
small - corpora in the Universal Dependency Tree-
banks
1
; in this respect, our study is based on conve-
nience sampling. For some languages like Indone-
sian, larger Dependency treebanks as models need to
1
https://universaldependencies.org
Uniform Density in Linguistic Information Derived from Dependency Structures
497