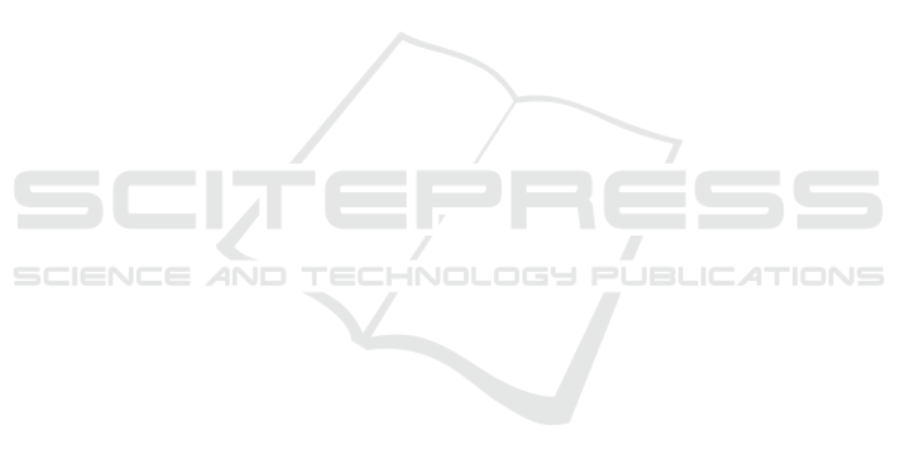
Do
ˇ
silovi
´
c, F. K., Br
ˇ
ci
´
c, M., and Hlupi
´
c, N. (2018). Ex-
plainable artificial intelligence: A survey. In 2018 41st
International convention on information and commu-
nication technology, electronics and microelectronics
(MIPRO), pages 0210–0215. IEEE.
Erickson, N., Mueller, J., Shirkov, A., Zhang, H., Larroy,
P., Li, M., and Smola, A. (2020). Autogluon-tabular:
Robust and accurate automl for structured data. arXiv
preprint arXiv:2003.06505.
Ferreira, L., Pilastri, A., Martins, C., Santos, P., and Cortez,
P. (2020). An automated and distributed machine
learning framework for telecommunications risk man-
agement. In ICAART (2), pages 99–107.
Feurer, M., Klein, A., Eggensperger, K., Springenberg, J.,
Blum, M., and Hutter, F. (2015a). Efficient and robust
automated machine learning. In Advances in neural
information processing systems, pages 2962–2970.
Feurer, M., Klein, A., Eggensperger, K., Springenberg,
J. T., Blum, M., and Hutter, F. (2015b). Supplemen-
tary material for efficient and robust automated ma-
chine learning. Advances in Neural Information Pro-
cessing Systems, pages 1–13.
Gijsbers, P., LeDell, E., Thomas, J., Poirier, S., Bischl, B.,
and Vanschoren, J. (2019). An open source automl
benchmark. arXiv preprint: 1907.00909.
Gilpin, L. H., Bau, D., Yuan, B. Z., Bajwa, A., Specter,
M., and Kagal, L. (2018). Explaining explanations:
An overview of interpretability of machine learning.
In 2018 IEEE 5th International Conference on data
science and advanced analytics (DSAA), pages 80–89.
IEEE.
Goodman, B. and Flaxman, S. (2017). European union reg-
ulations on algorithmic decision-making and a “right
to explanation”. AI magazine, 38(3):50–57.
Guidotti, R., Monreale, A., Ruggieri, S., Turini, F., Gian-
notti, F., and Pedreschi, D. (2018). A survey of meth-
ods for explaining black box models. ACM computing
surveys (CSUR), 51(5):1–42.
Guyon, I., Chaabane, I., Escalante, H. J., Escalera, S.,
Jajetic, D., Lloyd, J. R., Maci
`
a, N., Ray, B., Ro-
maszko, L., Sebag, M., et al. (2016). A brief review of
the chalearn automl challenge: any-time any-dataset
learning without human intervention. In Workshop on
Automatic Machine Learning, pages 21–30.
Guyon, I., Sun-Hosoya, L., Boull
´
e, M., Escalante, H. J.,
Escalera, S., Liu, Z., Jajetic, D., Ray, B., Saeed, M.,
Sebag, M., et al. (2019). Analysis of the automl chal-
lenge series. Automated Machine Learning, page 177.
Hanussek, M., Blohm, M., and Kintz, M. (2020). Can
automl outperform humans? an evaluation on popu-
lar openml datasets using automl benchmark. arXiv
preprint arXiv:2009.01564.
He, X., Zhao, K., and Chu, X. (2021). Automl: A sur-
vey of the state-of-the-art. Knowledge-Based Systems,
212:106622.
Hutter, F., Kotthoff, L., and Vanschoren, J. (2019). Au-
tomated machine learning: methods, systems, chal-
lenges. Springer Nature.
LeDell, E. and Poirier, S. (2020). H2o automl: Scalable
automatic machine learning. In 7th ICML workshop
on automated machine learning.
Lipton, Z. C. (2018). The mythos of model interpretability.
Queue, 16(3):31–57.
Lundberg, S. M. and Lee, S.-I. (2017). A unified approach
to interpreting model predictions. In Advances in neu-
ral information processing systems, pages 4765–4774.
Miller, T. (2019). Explanation in artificial intelligence: In-
sights from the social sciences. Artificial Intelligence,
267:1–38.
Molnar, C. (2018). Interpretable Machine Learning - A
Guide for Making Black Box Models Explainable.
Olson, R. and Moore, J. (2019). Automated Machine Learn-
ing, chapter TPOT: A Tree-Based Pipeline Optimiza-
tion Tool for Automating Machine Learning, pages
151–160. Springer.
Olson, R. S., Bartley, N., Urbanowicz, R. J., and Moore,
J. H. (2016). Evaluation of a tree-based pipeline op-
timization tool for automating data science. In Pro-
ceedings of the Genetic and Evolutionary Computa-
tion Conference 2016, GECCO ’16, pages 485–492,
New York, NY, USA. ACM.
Rao, T. R., Mitra, P., Bhatt, R., and Goswami, A. (2019).
The big data system, components, tools, and technolo-
gies: a survey. Knowledge and Information Systems,
pages 1–81.
Ribeiro, M. T., Singh, S., and Guestrin, C. (2016). ” why
should i trust you?” explaining the predictions of any
classifier. In Proceedings of the 22nd ACM SIGKDD
international conference on knowledge discovery and
data mining, pages 1135–1144.
Snoek, J., Larochelle, H., and Adams, R. P. (2012). Prac-
tical Bayesian Optimization of Machine Learning Al-
gorithms. Advances in Neural Information Processing
Systems 25 (NIPS), pages 1–9.
Umayaparvathi, V. and Iyakutti, K. (2016). Attribute se-
lection and customer churn prediction in telecom in-
dustry. In 2016 international conference on data min-
ing and advanced computing (sapience), pages 84–90.
IEEE.
Wang, B., Xu, H., Zhang, J., Chen, C., Fang, X., Kang,
N., Hong, L., Zhang, W., Li, Y., Liu, Z., et al.
(2020). Vega: towards an end-to-end configurable au-
toml pipeline. arXiv preprint arXiv:2011.01507.
Wang, X., Li, B., Zhang, Y., Kailkhura, B., and Nahrst-
edt, K. (2021). Robusta: Robust automl for feature
selection via reinforcement learning. arXiv preprint
arXiv:2101.05950.
eSardine: A General Purpose Platform with Autonomous AI and Explainable Outputs
625