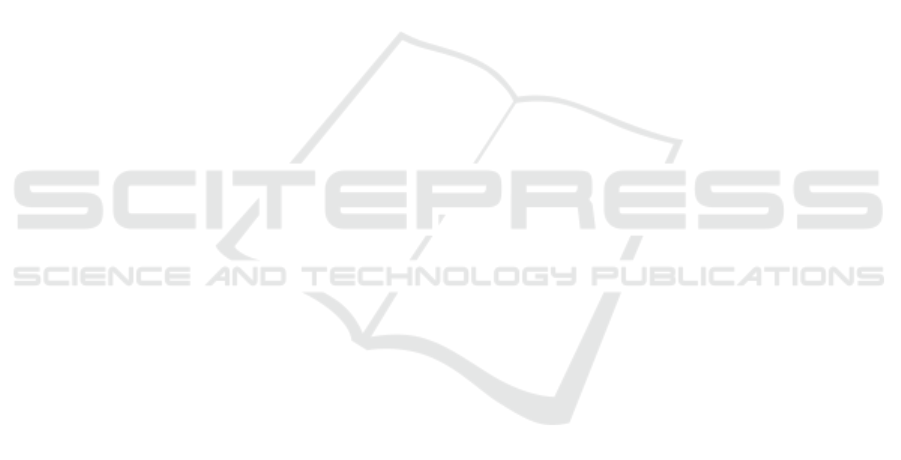
signal-based method for measurement of pain inten-
sity. Frontiers in Neuroscience, 11(MAY):279.
Cordell, W. H., Keene, K. K., Giles, B. K., Jones, J. B.,
Jones, J. H., and Brizendine, E. J. (2002). The high
prevalence of pain in emergency medical care. Amer-
ican Journal of Emergency Medicine, 20(3):165–169.
Fariha, M. A. Z., Ikeura, R., Hayakawa, S., and Tsutsumi, S.
(2020). Analysis of Pan-Tompkins Algorithm Perfor-
mance with Noisy ECG Signals. Journal of Physics:
Conference Series, 1532(1):12022.
Gaskin, D. J. and Richard, P. (2012). The Economic Costs
of Pain in the United States. The Journal of Pain,
13(8):715–724.
Hadjistavropoulos, T. and Craig, K. D. (2002). A theoretical
framework for understanding self-report and observa-
tional measures of pain: A communications model.
Behaviour Research and Therapy, 40(5):551–570.
Hinduja, S., Canavan, S., and Kaur, G. (2020). Multi-
modal Fusion of Physiological Signals and Facial Ac-
tion Units for Pain Recognition. In Struc V., G.-F. F.,
editor, IEEE International Conference on Automatic
Face and Gesture Recognition, pages 577–581. Insti-
tute of Electrical and Electronics Engineers Inc.
K
¨
achele, M., Thiam, P., Amirian, M., Schwenker, F.,
and Palm, G. (2016). Methods for Person-Centered
Continuous Pain Intensity Assessment from Bio-
Physiological Channels. IEEE Journal on Selected
Topics in Signal Processing, 10(5):854–864.
Liu, F., Wei, S., Li, Y., Jiang, X., Zhang, Z., Zhang, L., and
Liu, C. (2017). The accuracy on the common Pan-
Tompkins based QRS detection methods through low-
quality electrocardiogram database. Journal of Medi-
cal Imaging and Health Informatics, 7(5):1039–1043.
Lopez-Martinez, D. and Picard, R. (2017). Multi-task neu-
ral networks for personalized pain recognition from
physiological signals. In International Conference on
Affective Computing and Intelligent Interaction Work-
shops and Demos, pages 181–184, San Antonio. Insti-
tute of Electrical and Electronics Engineers Inc.
Lopez-Martinez, D. and Picard, R. (2018). Continuous Pain
Intensity Estimation from Autonomic Signals with
Recurrent Neural Networks. In International Confer-
ence of the IEEE Engineering in Medicine and Biol-
ogy Society., volume 2018, pages 5624–5627.
Lynch, M. E. (2011). The need for a Canadian pain strategy.
Pain Research and Management, 16(2):77–80.
Mamontov, D., Polonskaia, I., Skorokhod, A., Semenkin,
E., Kessler, V., and Schwenker, F. (2019). Evo-
lutionary algorithms for the design of neural net-
work classifiers for the classification of pain intensity.
Multimodal Pattern Recognition of Social Signals in
Human-Computer-Interaction, 11377 LNAI:84–100.
Merskey, H., Albe-Fessard, D. G., Bonica, J. J., Carmen, A.,
Dubner, R., Kerr, F. W. L., and Pagni, C. A. (1979).
Editorial: The need of a taxonomy. Pain, 6(3):247–
252.
Pan, J. and Tompkins, W. J. (1985). A Real-Time QRS De-
tection Algorithm. IEEE Transactions on Biomedical
Engineering, BME-32(3):230–236.
Rohling, M. L., Binder, L. M., and Langhinrichsen-
Rohling, J. (1995). Money Matters: A Meta-
Analytic Review of the Association Between Finan-
cial Compensation and the Experience and Treatment
of Chronic Pain. Health Psychology, 14(6):537–547.
Stewart, G. and Panickar, A. (2013). Role of the sympa-
thetic nervous system in pain.
Subramaniam, S. D. and Dass, B. (2021). Automated Noci-
ceptive Pain Assessment Using Physiological Signals
and a Hybrid Deep Learning Network. IEEE Sensors
Journal, 21(3):3335–3343.
Thiam, P., Bellmann, P., Kestler, H. A., and Schwenker, F.
(2019). Exploring deep physiological models for no-
ciceptive pain recognition. Sensors, 19(20):4503.
Toomey, M. (2008). Gender differences in pain: does X =
Y? AANA journal, 76(5):355–359.
Walter, S., Gruss, S., Ehleiter, H., Tan, J., Traue, H. C.,
Crawcour, S., Werner, P., Al-Hamadi, A., Andrade,
A. O., and Da Silva, G. M. (2013). The biovid heat
pain database: Data for the advancement and system-
atic validation of an automated pain recognition. In
2013 IEEE International Conference on Cybernetics,
pages 128–131.
Wang, J., Wei, M., Zhang, L., Huang, G., Liang, Z., Li,
L., and Zhang, Z. (2020). An Autoencoder-based
Approach to Predict Subjective Pain Perception from
High-density Evoked EEG Potentials. In International
Conference of the IEEE Engineering in Medicine Bi-
ology Society, pages 1507–1511. Institute of Electrical
and Electronics Engineers Inc.
Werner, P., Al-Hamadi, A., Niese, R., Walter, S., Gruss,
S., and Traue, H. C. (2014). Automatic pain recog-
nition from video and biomedical signals. In Inter-
national Conference on Pattern Recognition, pages
4582–4587. Institute of Electrical and Electronics En-
gineers Inc.
Werner, P., Lopez-Martinez, D., Walter, S., Al-Hamadi, A.,
Gruss, S., and Picard, R. (2019). Automatic Recogni-
tion Methods Supporting Pain Assessment: A Survey.
IEEE Transactions on Affective Computing.
Yu, M., Sun, Y., Zhu, B., Zhu, L., Lin, Y., Tang, X., Guo,
Y., Sun, G., and Dong, M. (2020). Diverse frequency
band-based convolutional neural networks for tonic
cold pain assessment using EEG. Neurocomputing,
378:270–282.
Zhao, K., Li, Y., Wang, G., Pu, Y., and Lian, Y. (2021).
A robust QRS detection and accurate R-peak identifi-
cation algorithm for wearable ECG sensors. Science
China Information Sciences, 64(8):182401.
ICT4AWE 2022 - 8th International Conference on Information and Communication Technologies for Ageing Well and e-Health
162