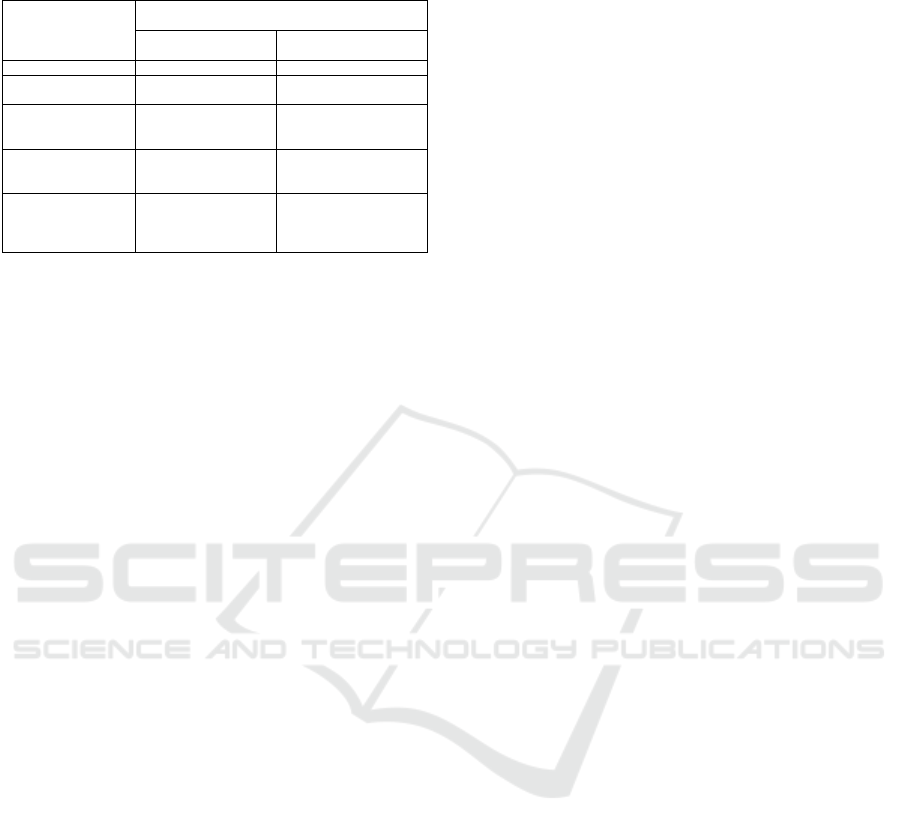
Table 4: Proposed Intelligent Saliency-Motion Driving risk
assessment system.
Method
Intelligent Domain Adapted Risk
Assessment Performance
Low Risk
(Static Salient Objects)
High Risk
(Salient Moving Objects)
Proposed 96.78% 96.66%
Proposed w/o
GRL
91.87% 91.09%
Classic SS-FCN
With Attention
(Rundo et al., 2021)
91.65% 90.90%
Classic SS-FCN
DenseNet
Backbone
89.30% 90.11%
Classic SS-FCN
ResNet-101
backbone
(Min and Corso, 2019)
87.79% 90.01%
Future works aim on embedding such features of
deep LSTM with Attention to further improve the per-
formance of overall pipeline (Rundo, 2019).
ACKNOWLEDGEMENTS
The authors thank the physiologists belonging to the
Department of Biomedical and Biotechnological Sci-
ences (BIOMETEC) of the University of Catania,
who collaborated in this work in the context of the
clinical study Ethical Committee CT1 authorization
n.113 / 2018 / PO. This research was funded by
the National Funded Program 2014-2020 under grant
agreement n. 1733, (ADAS + Project). The reported
information is covered by the following registered
patents: IT Patent Nr. 102017000120714, 24 Octo-
ber 2017. IT Patent Nr. 102019000005868, 16 April
2018; IT Patent Nr. 102019000000133, 07 January
2019.
REFERENCES
Alletto, S., Abati, D., Calderara, S., Cucchiara, R., and
Rigazio, L. (2018). Self-supervised optical flow esti-
mation by projective bootstrap. IEEE Transactions on
Intelligent Transportation Systems, 20(9):3294–3302.
Barjenbruch, M., Kellner, D., Klappstein, J., Dickmann, J.,
and Dietmayer, K. (2015). Joint spatial-and doppler-
based ego-motion estimation for automotive radars. In
2015 IEEE Intelligent Vehicles Symposium (IV), pages
839–844. IEEE.
Carr
´
e, M., Exposito, E., and Ibanez-Guzman, J. (2018).
Challenges for the self-safety in autonomous vehicles.
In 2018 13th Annual Conference on System of Systems
Engineering (SoSE), pages 181–188. IEEE.
Conoci, S., Rundo, F., Fallica, G., Lena, D., Buraioli, I., and
Demarchi, D. (2018). Live demonstration of portable
systems based on silicon sensors for the monitoring
of physiological parameters of driver drowsiness and
pulse wave velocity. In 2018 IEEE Biomedical Cir-
cuits and Systems Conference (BioCAS), pages 1–3.
IEEE.
Ganin, Y. and Lempitsky, V. (2015). Unsupervised do-
main adaptation by backpropagation. In International
conference on machine learning, pages 1180–1189.
PMLR.
Grigorescu, S. M., Trasnea, B., Marina, L., Vasilcoi, A.,
and Cocias, T. (2019). Neurotrajectory: A neuroevo-
lutionary approach to local state trajectory learning for
autonomous vehicles. IEEE Robotics and Automation
Letters, 4(4):3441–3448.
Hee Lee, G., Faundorfer, F., and Pollefeys, M. (2013). Mo-
tion estimation for self-driving cars with a general-
ized camera. In Proceedings of the IEEE Conference
on Computer Vision and Pattern Recognition, pages
2746–2753.
Lee, H., Lee, J., and Shin, M. (2019). Using wearable
ecg/ppg sensors for driver drowsiness detection based
on distinguishable pattern of recurrence plots. Elec-
tronics, 8(2):192.
Min, K. and Corso, J. J. (2019). Tased-net: Temporally-
aggregating spatial encoder-decoder network for
video saliency detection. In Proceedings of the
IEEE/CVF International Conference on Computer Vi-
sion, pages 2394–2403.
Ogitsu, T. and Mizoguchi, H. (2015). A study on driver
training on advanced driver assistance systems by us-
ing a driving simulator. In 2015 International Confer-
ence on Connected Vehicles and Expo (ICCVE), pages
352–353. IEEE.
Okuda, R., Kajiwara, Y., and Terashima, K. (2014). A sur-
vey of technical trend of adas and autonomous driv-
ing. In Technical Papers of 2014 International Sym-
posium on VLSI Design, Automation and Test, pages
1–4. IEEE.
Panagiotopoulos, I. and Dimitrakopoulos, G. (2019). Cog-
nitive infotainment systems for intelligent vehicles. In
2019 10th International Conference on Information,
Intelligence, Systems and Applications (IISA), pages
1–8. IEEE.
Rundo, F. (2019). Deep lstm with reinforcement
learning layer for financial trend prediction in fx
high frequency trading systems. Applied Sciences,
9(20):4460.
Rundo, F., Conoci, S., Battiato, S., Trenta, F., and Spamp-
inato, C. (2020a). Innovative saliency based deep driv-
ing scene understanding system for automatic safety
assessment in next-generation cars. In 2020 AEIT In-
ternational Conference of Electrical and Electronic
Technologies for Automotive (AEIT AUTOMOTIVE),
pages 1–6. IEEE.
Rundo, F., Conoci, S., Ortis, A., and Battiato, S.
(2018a). An advanced bio-inspired photoplethysmog-
raphy (ppg) and ecg pattern recognition system for
medical assessment. Sensors, 18(2):405.
Rundo, F., Leotta, R., and Battiato, S. (2021). Real-time
deep neuro-vision embedded processing system for
saliency-based car driving safety monitoring. In 2021
4th International Conference on Circuits, Systems and
Simulation (ICCSS), pages 218–224. IEEE.
Objects Motion Detection in Domain-adapted Assisted Driving
107