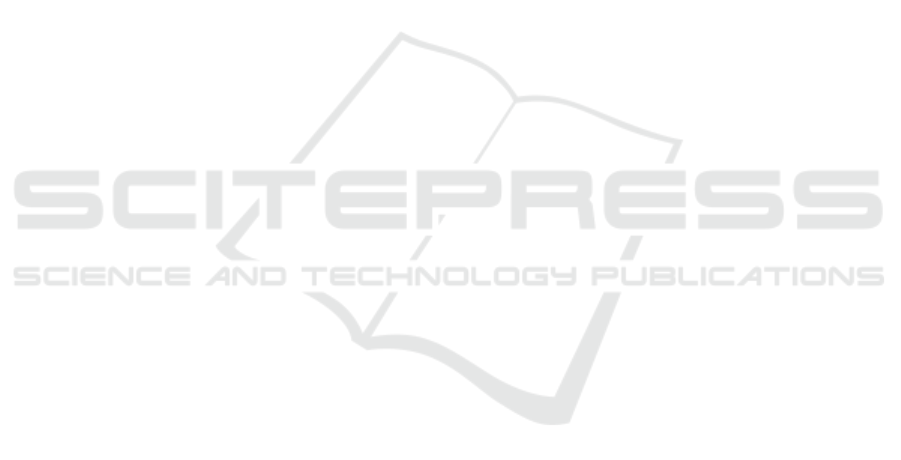
parking systems: Design, implementation and chal-
lenges. Image and Vision Computing, 68:88–101.
Horgan, J., Hughes, C., McDonald, J., and Yogamani, S.
(2015). Vision-based driver assistance systems: Sur-
vey, taxonomy and advances. In 2015 IEEE 18th In-
ternational Conference on Intelligent Transportation
Systems, pages 2032–2039. IEEE.
Loh, Y. P. and Chan, C. S. (2019). Getting to know low-light
images with the exclusively dark dataset. Computer
Vision and Image Understanding, 178:30–42.
Maddern, W., Pascoe, G., Linegar, C., and Newman, P.
(2017). 1 year, 1000 km: The oxford robotcar
dataset. The International Journal of Robotics Re-
search, 36(1):3–15.
Min, K. and Corso, J. J. (2019). Tased-net: Temporally-
aggregating spatial encoder-decoder network for
video saliency detection. In Proceedings of the
IEEE/CVF International Conference on Computer Vi-
sion, pages 2394–2403.
Mizutani, H. (1994). A new learning method for multi-
layered cellular neural networks. In Proceedings of
the Third IEEE International Workshop on Cellular
Neural Networks and their Applications (CNNA-94),
pages 195–200. IEEE.
Pham, L. H., Tran, D. N.-N., and Jeon, J. W. (2020). Low-
light image enhancement for autonomous driving sys-
tems using driveretinex-net. In 2020 IEEE Inter-
national Conference on Consumer Electronics-Asia
(ICCE-Asia), pages 1–5. IEEE.
Qu, Y., Ou, Y., and Xiong, R. (2019). Low illumination
enhancement for object detection in self-driving. In
2019 IEEE International Conference on Robotics and
Biomimetics (ROBIO), pages 1738–1743. IEEE.
Rashed, H., Ramzy, M., Vaquero, V., El Sallab, A., Sistu,
G., and Yogamani, S. (2019). Fusemodnet: Real-time
camera and lidar based moving object detection for ro-
bust low-light autonomous driving. In Proceedings of
the IEEE/CVF International Conference on Computer
Vision Workshops, pages 0–0.
Roska, T. and Chua, L. O. (1992). Cellular neural networks
with non-linear and delay-type template elements and
non-uniform grids. International Journal of Circuit
Theory and Applications, 20(5):469–481.
Rundo, F. (2021). Intelligent real-time deep system for
robust objects tracking in low-light driving scenario.
Computation, 9(11):117.
Rundo, F., Banna, G. L., Prezzavento, L., Trenta, F.,
Conoci, S., and Battiato, S. (2020a). 3d non-
local neural network: A non-invasive biomarker for
immunotherapy treatment outcome prediction. case-
study: Metastatic urothelial carcinoma. Journal of
Imaging, 6(12):133.
Rundo, F., Conoci, S., Banna, G. L., Ortis, A., Stanco, F.,
and Battiato, S. (2018a). Evaluation of levenberg–
marquardt neural networks and stacked autoencoders
clustering for skin lesion analysis, screening and
follow-up. IET Computer Vision, 12(7):957–962.
Rundo, F., Conoci, S., Battiato, S., Trenta, F., and Spamp-
inato, C. (2020b). Innovative saliency based deep
driving scene understanding system for automatic
safety assessment in next-generation cars. In 2020
AEIT International Conference of Electrical and Elec-
tronic Technologies for Automotive (AEIT AUTOMO-
TIVE), pages 1–6. IEEE.
Rundo, F., Conoci, S., Spampinato, C., Leotta, R., Trenta,
F., and Battiato, S. (2021a). Deep neuro-vision em-
bedded architecture for safety assessment in percep-
tive advanced driver assistance systems: the pedes-
trian tracking system use-case. Frontiers in neuroin-
formatics, 15.
Rundo, F., Leotta, R., and Battiato, S. (2021b). Real-time
deep neuro-vision embedded processing system for
saliency-based car driving safety monitoring. In 2021
4th International Conference on Circuits, Systems and
Simulation (ICCSS), pages 218–224. IEEE.
Rundo, F., Petralia, S., Fallica, G., and Conoci, S. (2018b).
A nonlinear pattern recognition pipeline for ppg/ecg
medical assessments. In Convegno Nazionale Sensori,
pages 473–480. Springer.
Rundo, F., Rinella, S., Massimino, S., Coco, M., Fallica, G.,
Parenti, R., Conoci, S., and Perciavalle, V. (2019a).
An innovative deep learning algorithm for drowsiness
detection from eeg signal. Computation, 7(1):13.
Rundo, F., Spampinato, C., Battiato, S., Trenta, F., and
Conoci, S. (2020c). Advanced 1d temporal deep di-
lated convolutional embedded perceptual system for
fast car-driver drowsiness monitoring. In 2020 AEIT
International Conference of Electrical and Electronic
Technologies for Automotive (AEIT AUTOMOTIVE),
pages 1–6. IEEE.
Rundo, F., Spampinato, C., and Conoci, S. (2019b). Ad-hoc
shallow neural network to learn hyper filtered photo-
plethysmographic (ppg) signal for efficient car-driver
drowsiness monitoring. Electronics, 8(8):890.
Rundo, F., Trenta, F., Di Stallo, A. L., and Battiato, S.
(2019c). Advanced markov-based machine learning
framework for making adaptive trading system. Com-
putation, 7(1):4.
Rundo, F., Trenta, F., di Stallo, A. L., and Battiato, S.
(2019d). Grid trading system robot (gtsbot): A novel
mathematical algorithm for trading fx market. Applied
Sciences, 9(9):1796.
STMicroelectronics (2018). STMicroelectronics
ACCORDO 5 Automotive Microcontroller.
https://www.st.com/en/automotive-infotainment-
and-telematics/sta1295.html. (accessed on 01 June
2021).
Szankin, M., Kwa
´
sniewska, A., Ruminski, J., and Nico-
las, R. (2018). Road condition evaluation using fu-
sion of multiple deep models on always-on vision pro-
cessor. In IECON 2018-44th Annual Conference of
the IEEE Industrial Electronics Society, pages 3273–
3279. IEEE.
Trenta, F., Conoci, S., Rundo, F., and Battiato, S. (2019).
Advanced motion-tracking system with multi-layers
deep learning framework for innovative car-driver
drowsiness monitoring. In 2019 14th IEEE Inter-
national Conference on Automatic Face & Gesture
Recognition (FG 2019), pages 1–5. IEEE.
Wang, X., Girshick, R., Gupta, A., and He, K. (2018). Non-
local neural networks. In Proceedings of the IEEE
IMPROVE 2022 - 2nd International Conference on Image Processing and Vision Engineering
116