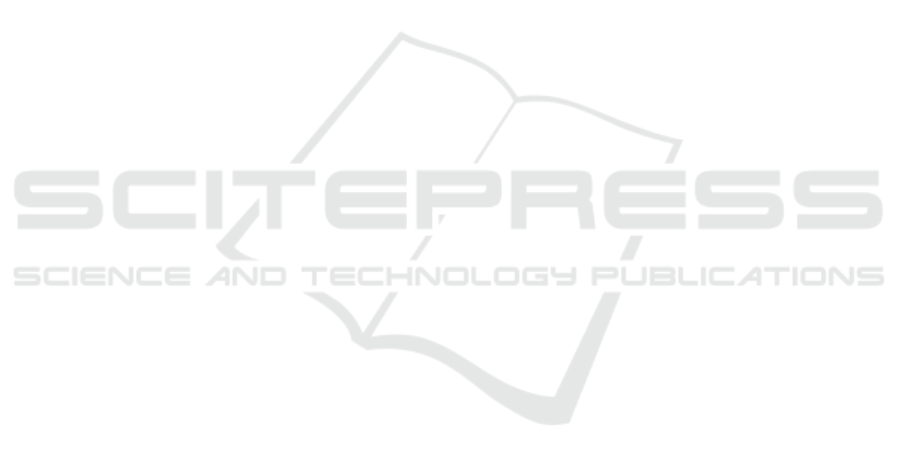
Proceedings of the National Academy of Sciences,
110(22):9100–9105.
Friston, K. (2005). A theory of cortical responses. Philo-
sophical transactions of the Royal Society B: Biologi-
cal sciences, 360(1456):815–836.
Friston, K. (2010). The free-energy principle: a uni-
fied brain theory? Nature reviews neuroscience,
11(2):127–138.
Gharaee, Z. (2018). Action in Mind: A Neural Net-
work Approach to Action Recognition and Seg-
mentation. Lund University: Cognitive Science.
DOI:https://arxiv.org/abs/2104.14870.
Gharaee, Z. (2020a). Hierarchical growing grid
networks for skeleton based action recogni-
tion. Cognitive Systems Research, 63:11–29.
DOI:https://doi.org/10.1016/j.cogsys.2020.05.002.
Gharaee, Z. (2020b). Online recognition of un-
segmented actions with hierarchical som architec-
ture. Cognitive Processing, 22:77–91. DOI:
https://doi.org/10.1007/s10339-020-00986-4.
Gharaee, Z., G
¨
ardenfors, P., and Johnsson, M. (2016).
Action recognition online with hierarchical self-
organizing maps. In Proceedings of Interna-
tional Conference on Signal Image Technology
and Internet Based Systems(SITIS), pages 538–544.
DOI:10.1109/SITIS.2016.91.
Gharaee, Z., G
¨
ardenfors, P., and Johnsson, M. (2017a).
First and second order dynamics in a hier-
archical som system for action recognition.
Applied Soft Computing, 59:574–585. DOI:
https://doi.org/10.1016/j.asoc.2017.06.007.
Gharaee, Z., G
¨
ardenfors, P., and Johnsson, M. (2017b).
Hierarchical self-organizing maps system for ac-
tion classification. In Proceedings of the In-
ternational Conference on Agents and Artificial
Intelligence (ICAART), pages 583–590. DOI:
10.5220/0006199305830590.
Gharaee, Z., G
¨
ardenfors, P., and Johnsson, M. (2017c). On-
line recognition of actions involving objects. Bio-
logically Inspired Cognitive Architectures, 22:10–19.
DOI:10.1016/j.bica.2017.09.007.
Gil, D., Garcia-Rodriguez, J., Cazorla, M., and Johnsson,
M. (2015). Sarasom: a supervised architecture based
on the recurrent associative som. Neural Computing
and Applications, 26(5):1103–1115.
Granada, R. L., Pereira, R. F., Monteiro, J., Ruiz, D. D. A.,
Barros, R. C., and Meneguzzi, F. R. (2017). Hybrid
activity and plan recognition for video streams. In
Proceedings of the 31st. AAAI Conference: Plan, Ac-
tivity and Intent Recognition Workshop, 2017, Estados
Unidos.
Hesslow, G. (2002). Conscious thought as simulation of be-
haviour and perception. Trends in cognitive sciences,
6(6):242–247.
Johnsson, M., Balkenius, C., and Hesslow, G. (2009). Asso-
ciative self-organizing map. In In the proceedings of
the International Joint Conference on Computational
Intelligence (IJCCI).
Joo, J., Li, W., Steen, F. F., and Zhu, S.-C. (2014). Vi-
sual persuasion: Inferring communicative intents of
images. In Proceedings of the IEEE conference on
computer vision and pattern recognition, pages 216–
223.
Karasev, V., Ayvaci, A., Heisele, B., and Soatto, S. (2016).
Intent-aware long-term prediction of pedestrian mo-
tion. In 2016 IEEE International Conference on
Robotics and Automation (ICRA), pages 2543–2549.
IEEE.
Kosslyn, S. M., Ganis, G., and Thompson, W. L. (2001).
Neural foundations of imagery. Nature reviews neuro-
science, 2(9):635–642.
Liu, K., Sibille, J., and Dragoi, G. (2018). Generative pre-
dictive codes by multiplexed hippocampal neuronal
tuplets. Neuron, 99(6):1329–1341.
´
Olafsd
´
ottir, H. F., Barry, C., Saleem, A. B., Hassabis, D.,
and Spiers, H. J. (2015). Hippocampal place cells con-
struct reward related sequences through unexplored
space. Elife, 4:e06063.
Pezzulo, G. and Cisek, P. (2016). Navigating the affordance
landscape: feedback control as a process model of be-
havior and cognition. Trends in cognitive sciences,
20(6):414–424.
Pezzulo, G., Maisto, D., Barca, L., and Van den Bergh, O.
(2019). Symptom perception from a predictive pro-
cessing perspective. Clinical Psychology in Europe,
1(4):1–14.
Pfeiffer, B. E. and Foster, D. J. (2013). Hippocampal
place-cell sequences depict future paths to remem-
bered goals. Nature, 497(7447):74–79.
Sciutti, A., Ansuini, C., Becchio, C., and Sandini, G.
(2015). Investigating the ability to read others’ inten-
tions using humanoid robots. Frontiers in psychology,
6:1362.
Seidenari, L., Varano, V., Berretti, S., Del Bimbo, A., and
Pala, P. (2013). Recognizing actions from depth cam-
eras as weakly aligned multi-part bag-of-poses. In
Proceedings of the IEEE Conference on Computer Vi-
sion and Pattern Recognition Workshops (CVPRW),
pages 479–485. DOI: 10.1109/CVPRW.2013.77.
Stoianov, I., Maisto, D., and Pezzulo, G. (2020). The
hippocampal formation as a hierarchical generative
model supporting generative replay and continual
learning. bioRxiv. DOI:10.1101/2020.01.16.908889.
Vinanzi, S., Goerick, C., and Cangelosi, A. (2019). Min-
dreading for robots: Predicting intentions via dynam-
ical clustering of human postures. In 2019 Joint IEEE
9th International Conference on Development and
Learning and Epigenetic Robotics (ICDL-EpiRob),
pages 272–277. IEEE.
Wan, Y. W. (accessed 2015). Msr action recognition
datasets and codes.
Xie, D., Shu, T., Todorovic, S., and Zhu, S.-C. (2017).
Learning and inferring “dark matter” and predicting
human intents and trajectories in videos. IEEE trans-
actions on pattern analysis and machine intelligence,
40(7):1639–1652.
Predicting the Intended Action using Internal Simulation of Perception
635