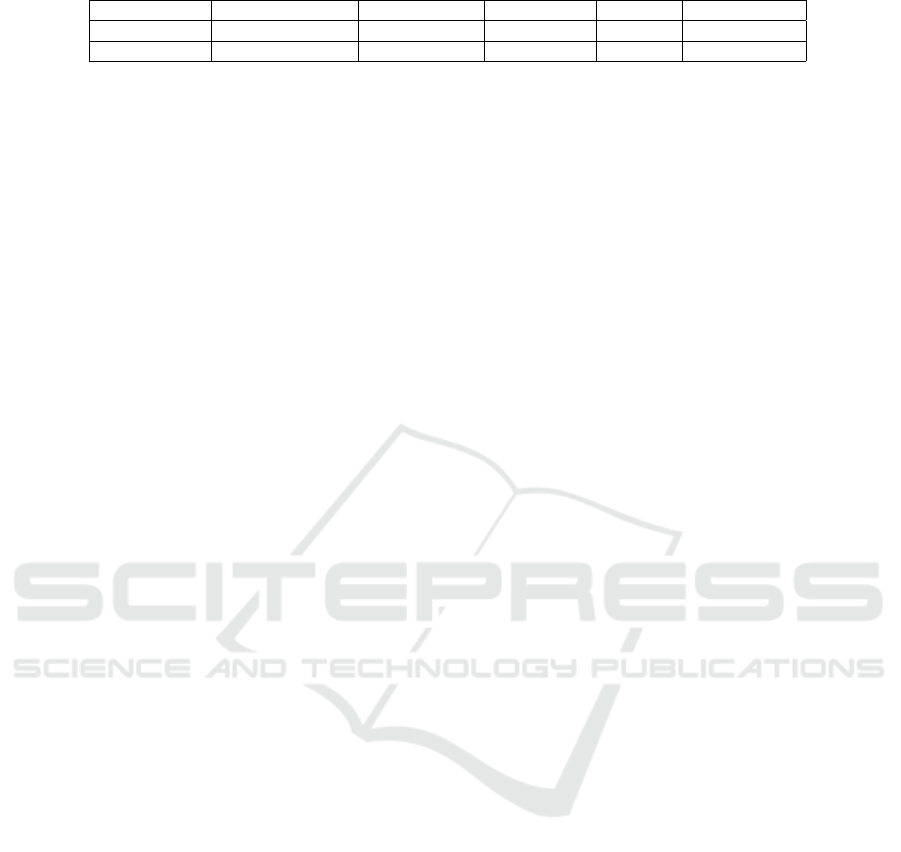
Table 4: Performance comparison.
Models Model parameters Inference time Average FPS mAP@.5 mAP@[.5:.95]
YOLOv5s 7.5 millions 0.007s to 0.01s 121 99.3% 85.9%
Faster R-CNN 105 millions 0.55s 1.8 98.7% 81.38%
terms of inference time and mAP. We also performed
a comparative study with Faster R-CNN. The results
showed that YOLOv5 has an overall better perfor-
mance. As perspectives, it seems interesting to de-
velop real-time ArSL recognition system in mobile
applications based YOLOv5s. Moreover, we need
to further experiments to enhance the performance of
YOLOv5s. It would be also interesting to compare
YOLOv5s against YOLOX-Tiny (Ge et al., 2021).
REFERENCES
Abdel-Fattah, M. A. (2005). Arabic sign language: a per-
spective. Journal of deaf studies and deaf education,
10(2):212–221.
Alani, A. A. and Cosma, G. (2021). Arsl-cnn: a convolu-
tional neural network for arabic sign language gesture
recognition.
Alawwad, R. A., Bchir, O., and Ismail, M. M. B. (2021).
Arabic sign language recognition using faster r-cnn.
Almasre, M. A. and Al-Nuaim, H. (2017). The performance
of individual and ensemble classifiers for an arabic
sign language recognition system. INTERNATIONAL
JOURNAL OF ADVANCED COMPUTER SCIENCE
AND APPLICATIONS, 8(5):307–315.
Alzohairi, R., Alghonaim, R., Alshehri, W., Aloqeely, S.,
Alzaidan, M., and Bchir, O. (2018). Image based ara-
bic sign language recognition system. International
Journal of Advanced Computer Science and Applica-
tions (IJACSA), 9(3).
Assaleh, K. and Al-Rousan, M. (2005). Recognition of ara-
bic sign language alphabet using polynomial classi-
fiers. EURASIP Journal on Advances in Signal Pro-
cessing, 2005(13):1–10.
Bantupalli, K. and Xie, Y. (2018). American sign language
recognition using deep learning and computer vision.
In 2018 IEEE International Conference on Big Data
(Big Data), pages 4896–4899. IEEE.
Bheda, V. and Radpour, D. (2017). Using deep convolu-
tional networks for gesture recognition in american
sign language. arXiv preprint arXiv:1710.06836.
Bochkovskiy, A., Wang, C.-Y., and Liao, H.-Y. M. (2020).
Yolov4: Optimal speed and accuracy of object detec-
tion. arXiv preprint arXiv:2004.10934.
Dahmani, D. and Larabi, S. (2014). User-independent
system for sign language finger spelling recognition.
Journal of Visual Communication and Image Repre-
sentation, 25(5):1240–1250.
Dipietro, L., Sabatini, A. M., and Dario, P. (2008). A survey
of glove-based systems and their applications. Ieee
transactions on systems, man, and cybernetics, part c
(applications and reviews), 38(4):461–482.
Dodge, S. and Karam, L. (2016). Understanding how image
quality affects deep neural networks. In 2016 eighth
international conference on quality of multimedia ex-
perience (QoMEX), pages 1–6. IEEE.
ElBadawy, M., Elons, A., Shedeed, H. A., and Tolba, M.
(2017). Arabic sign language recognition with 3d con-
volutional neural networks. In 2017 Eighth Interna-
tional Conference on Intelligent Computing and In-
formation Systems (ICICIS), pages 66–71. IEEE.
Ge, Z., Liu, S., Wang, F., Li, Z., and Sun, J. (2021). Yolox:
Exceeding yolo series in 2021. arXiv e-prints, pages
arXiv–2107.
Goswami, T. and Javaji, S. R. (2021). Cnn model for ameri-
can sign language recognition. In ICCCE 2020, pages
55–61. Springer.
Han, J., Zhang, D., Cheng, G., Guo, L., and Ren, J. (2014).
Object detection in optical remote sensing images
based on weakly supervised learning and high-level
feature learning. IEEE Transactions on Geoscience
and Remote Sensing, 53(6):3325–3337.
Hemayed, E. E. and Hassanien, A. S. (2010). Edge-based
recognizer for arabic sign language alphabet (ars2v-
arabic sign to voice). In 2010 International Computer
Engineering Conference (ICENCO), pages 121–127.
IEEE.
Hu, Q., Wang, P., Shen, C., van den Hengel, A., and Porikli,
F. (2017). Pushing the limits of deep cnns for pedes-
trian detection. IEEE Transactions on Circuits and
Systems for Video Technology, 28(6):1358–1368.
Islam, S., Mousumi, S. S. S., Rabby, A. S. A., Hossain,
S. A., and Abujar, S. (2018). A potent model to recog-
nize bangla sign language digits using convolutional
neural network. Procedia computer science, 143:611–
618.
Jiao, L., Zhang, F., Liu, F., Yang, S., Li, L., Feng, Z., and
Qu, R. (2019). A survey of deep learning-based object
detection. IEEE access, 7:128837–128868.
Krizhevsky, A., Sutskever, I., and Hinton, G. E. (2012). Im-
agenet classification with deep convolutional neural
networks. Advances in neural information processing
systems, 25:1097–1105.
Latif, G., Mohammad, N., Alghazo, J., AlKhalaf, R., and
AlKhalaf, R. (2019). Arasl: Arabic alphabets sign
language dataset. Data in brief, 23:103777.
Lin, T.-Y., Maire, M., and Belongie, S. e. a. (2014). Mi-
crosoft coco: Common objects in context. In Euro-
pean conference on computer vision, pages 740–755.
Springer.
Liu, S., Qi, L., Qin, H., Shi, J., and Jia, J. (2018). Path ag-
gregation network for instance segmentation. In Pro-
ceedings of the IEEE conference on computer vision
and pattern recognition, pages 8759–8768.
Mikołajczyk, A. and Grochowski, M. (2018). Data augmen-
tation for improving deep learning in image classifica-
IMPROVE 2022 - 2nd International Conference on Image Processing and Vision Engineering
24