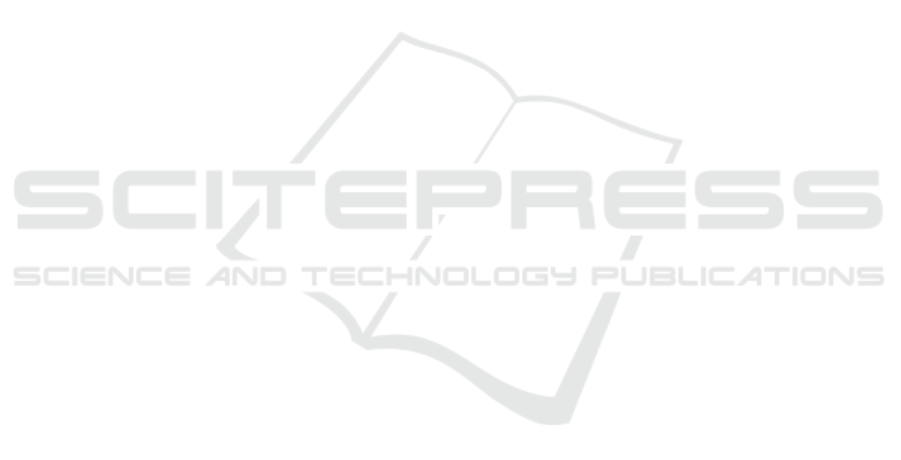
troduce bias, by privileging students who adopt the
most common behavior, for example.
5 CONCLUSIONS AND
PERSPECTIVES
Broadly, the selected classification method (k-means
algorithm) was appropriate to identify groups in
which students share similar behaviors according to
the selected indicators. The different steps described,
from the selection of the dataset to the conception
of personas, allow us to answer the RQ1. In paral-
lel, the unprecedented conception of personas based
on these identified groups is then effective to de-
scribe these learning behaviors semantically and thus
completes the numerical results returned by the algo-
rithm. These personas can then be shared with all
the LA actors who will be able to understand them,
whether they are technophiles or not, and then re-
spond to the RQ2. Altogether, these elements intro-
duce some interesting insights about how to character-
ize LA datasets more completely and understandably.
This new description of corpus, based on learner per-
sonas, seems to be able to become a powerful tool in
the LA field, participating in learning improvement
for the entire student population.
Nevertheless, some aspects were pointed out and
deserve to be studied and evaluated. First of all, we
wonder if the embodiment of the personas, by giving
a name, a gender, and an age to the fictive student, is
relevant in some contexts and does not introduce other
bias in the people who have to use them. Cognitive
bias can appear and affect the way LA actors interpret
the personas and use them. A study focusing on this
aspect seems to be needed to answer this question.
The automation of personas conception can also
be discussed: we ask ourselves if the redaction of the
learning behaviors could be automated with specific
models. It seems to be essential when dealing with
very large datasets, in which the description of hun-
dred of personas implies a large workload. In another
way, having a human intervention can reassure users
and participate in the enhancement of systems’ expli-
cability. These aspects thus deserve an in-depth anal-
ysis to determine the ideal comprise between com-
plete automation and human contribution.
Finally, the presented methodology looks promis-
ing and offers interesting results but was only applied
to a unique dataset in the paper. Now, we must study
how the methodology applies to multiple datasets,
like those shared on the LOLA platform, and from
which different learning indicators can be computed.
Application to a private dataset has started and is ex-
pected to be completed in the near future. This work
contributes to the affirmation of the robustness of our
method and could allow us to impose learner personas
as a privileged tool for LA dataset characterization.
ACKNOWLEDGEMENTS
This work has been done in the framework of the
LOLA project, with the support of the French Min-
istry of Higher Education, Research, and Innovation.
REFERENCES
Arnold, K. E. and Pistilli, M. D. (2012). Course signals at
purdue: Using learning analytics to increase student
success. In Proceedings of the 2nd international con-
ference on learning analytics and knowledge, pages
267–270.
Ben Soussia, A., Roussanaly, A., and Boyer, A. (2021).
An in-depth methodology to predict at-risk learners.
In European Conference on Technology Enhanced
Learning, pages 193–206. Springer.
Boroujeni, M. S., Sharma, K., Kidzi
´
nski, Ł., Lucignano, L.,
and Dillenbourg, P. (2016). How to quantify student’s
regularity? In European conference on technology
enhanced learning, pages 277–291. Springer.
Brooks, C. and Greer, J. (2014). Explaining predictive mod-
els to learning specialists using personas. In Proceed-
ings of the fourth international conference on learning
analytics and knowledge, pages 26–30.
Chi, M. T. and Wylie, R. (2014). The icap framework: Link-
ing cognitive engagement to active learning outcomes.
Educational psychologist, 49(4):219–243.
Davies, D. L. and Bouldin, D. W. (1979). A cluster separa-
tion measure. IEEE transactions on pattern analysis
and machine intelligence, (2):224–227.
Dehaene, S. (2013). Les quatres piliers de l’apprentissage,
ou ce que nous disent les neurosciences.
El-Bishouty, M. M., Aldraiweesh, A., Alturki, U., Tor-
torella, R., Yang, J., Chang, T.-W., Graf, S., et al.
(2019). Use of felder and silverman learning style
model for online course design. Educational Tech-
nology Research and Development, 67(1):161–177.
Felder, R. M., Silverman, L. K., et al. (1988). Learning and
teaching styles in engineering education. Engineering
education, 78(7):674–681.
Hussain, M., Zhu, W., Zhang, W., and Abidi, S. M. R.
(2018). Student engagement predictions in an e-
learning system and their impact on student course as-
sessment scores. Computational intelligence and neu-
roscience, 2018.
Iksal, S. (2012). Ing
´
enierie de l’observation bas
´
ee sur
la prescription en EIAH. PhD thesis, Universit
´
e du
Maine.
A New Way to Characterize Learning Datasets
43