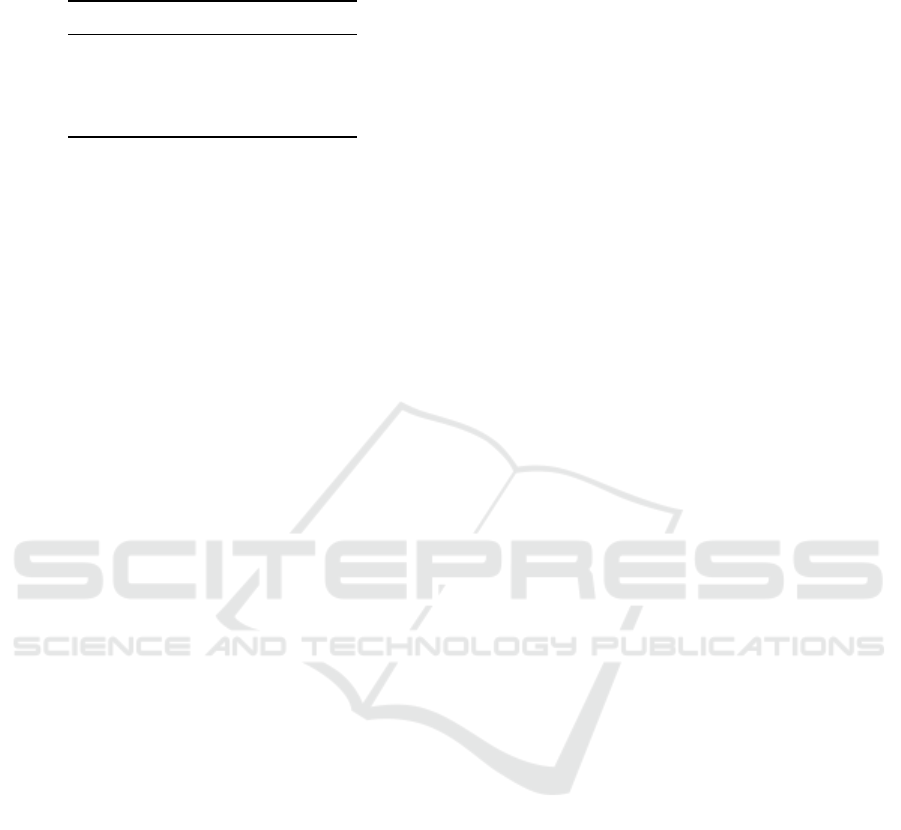
Table 2: Performance comparison among similar NN-based
works that utilized the same Anger dataset.
Reference Accuracy
(Huang et al., 2020) 93.6%
Proposed 86.0%
(Qin et al., 2018) 83.3%
(Jin et al., 2020) 79.7%
score using a two-stream NN-based model, where
each sub-stream has 60 hidden neurons followed by
a single fully-connected final prediction layer. It is
worth noticing that we used a simpler feedforward
single stream architecture with only twelve hidden
units, which is at least ten times less than what they
used. We aimed to achieve reasonable accuracy using
minimum computational burden (hidden units). We
fulfill our aim by accomplishing a reasonably good
performance (86%) with a simple structure after tun-
ing the hyperparameter and hidden layer compres-
sion.
5 CONCLUSION AND FUTURE
WORK
Anger is one of the many powerful emotions of hu-
mans, and finding its authenticity is essential in emo-
tion recognition and human-centered computing ar-
eas. We have developed a simple NN to classify real
and posed anger based on observers’ pupillary re-
sponses. Our research indicates that the anger classi-
fication is achievable by employing this simple struc-
tured NN. We have optimized the NN in two ways,
namely backpropagation and EA. With some rea-
sonable adjustment during testing the network, we
have developed a model that provided as high as
86% accuracy. This high accuracy proved that per-
ceivers’ pupillary response patterns could reflect the
anger they saw as genuine or posed even though the
perceivers are unconscious. Furthermore, our study
shows that three neurons can be removed without sig-
nificant performance degradation through NN prun-
ing.
To ensure the superiority of our proposed ap-
proach, future works will include alternative mod-
els to benchmark and various statistical tests, for ex-
ample Augmented Dickey-Fuller test, Kolmogorov-
Smirnova test, and Shapiro-Wilk test (Fan et al.,
2021) to validate. To build a relationship between
emotion and speech, authors in (Han et al., 2014) have
developed an efficient single-hidden-layer NN (called
extreme learning machine) for emotion recognition
based on utterance level speech features. Therefore,
for future work, we shall design and optimize a net-
work that uses people’s speech emotion to predict
whether their facial emotion is genuine or not, which
could be interesting. Moreover, we can also imple-
ment advanced network pruning techniques, such as
using thresholds and structured filter level pruning
(Luo et al., 2017).
REFERENCES
Chen, L., Gedeon, T., Hossain, M. Z., and Caldwell, S.
(2017). Are you really angry? detecting emotion ve-
racity as a proposed tool for interaction. In Proceed-
ings of the 29th Australian Conference on Computer-
Human Interaction, OZCHI ’17, page 412–416, New
York, NY, USA. Association for Computing Machin-
ery.
Fan, G.-F., Yu, M., Dong, S.-Q., Yeh, Y.-H., and Hong, W.-
C. (2021). Forecasting short-term electricity load us-
ing hybrid support vector regression with grey catas-
trophe and random forest modeling. Utilities Policy,
73:101294.
Gedeon, T. and Harris, D. (1992). Progressive image
compression. In [Proceedings 1992] IJCNN Inter-
national Joint Conference on Neural Networks, vol-
ume 4, pages 403–407.
Han, K., Yu, D., and Tashev, I. (2014). Speech emotion
recognition using deep neural network and extreme
learning machine. In Fifteenth annual conference of
the international speech communication association
(INTERSPEECH), pages 223–227.
Hossain, M. Z. and Gedeon, T. (2017). Classifying posed
and real smiles from observers’ peripheral physiol-
ogy. In Proceedings of the 11th EAI International
Conference on Pervasive Computing Technologies for
Healthcare, PervasiveHealth ’17, page 460–463, New
York, NY, USA. Association for Computing Machin-
ery.
Hossain, M. Z. and Gedeon, T. (2019). Observers’ physi-
ological measures in response to videos can be used
to detect genuine smiles. International Journal of
Human-Computer Studies, 122:232–241.
Huang, C., Zhu, X., and Gedeon, T. (2020). A genetic
feature selection based two-stream neural network for
anger veracity recognition. In Yang, H., Pasupa, K.,
Leung, A. C.-S., Kwok, J. T., Chan, J. H., and King,
I., editors, Neural Information Processing, pages 3–
11, Cham. Springer International Publishing.
Irani, R., Nasrollahi, K., Dhall, A., Moeslund, T. B., and
Gedeon, T. (2016). Thermal super-pixels for bimodal
stress recognition. In 2016 Sixth International Con-
ference on Image Processing Theory, Tools and Appli-
cations (IPTA), pages 1–6.
Jin, R., Zhu, X., and Fu, Y.-S. (2020). Identifying anger ve-
racity using neural network and long-short term mem-
ory with bimodal distribution removal. In Yang, H.,
Pasupa, K., Leung, A. C.-S., Kwok, J. T., Chan, J. H.,
and King, I., editors, Neural Information Processing,
ICAART 2022 - 14th International Conference on Agents and Artificial Intelligence
660