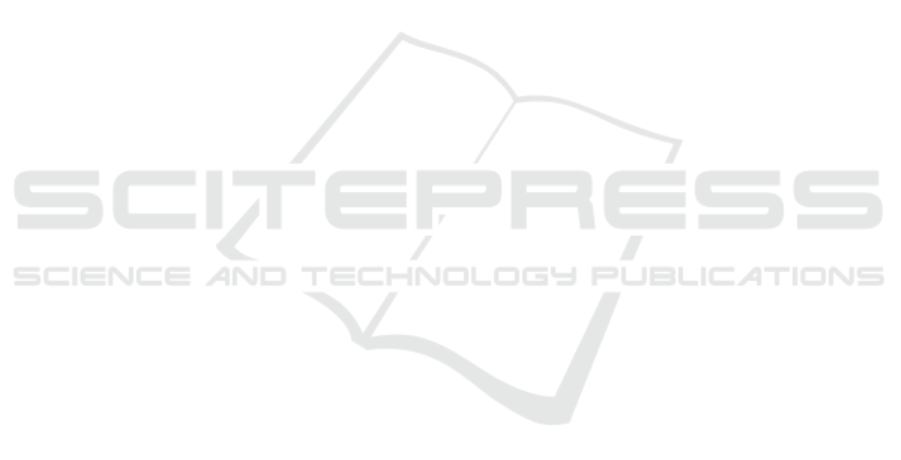
ACKNOWLEDGEMENTS
This paper has been supported by the RUDN Univer-
sity Strategic Academic Leadership Program. The re-
search was carried out using the infrastructure of the
shared research facilities ‘High Performance Comput-
ing and Big Data’ of FRC CSC RAS (CKP ‘Informat-
ics’).
REFERENCES
Azur, M. J., Stuart, E. A., Frangakis, C., and Leaf, P. J.
(2011). Multiple imputation by chained equations:
what is it and how does it work? International journal
of methods in psychiatric research, 20(1):40–49.
Beck, A. T., Steer, R. A., Brown, G. K., et al. (1996). Man-
ual for the beck depression inventory-ii.
Beck, A. T., Steer, R. A., and Carbin, M. G. (1988). Psy-
chometric properties of the beck depression inventory:
Twenty-five years of evaluation. Clinical psychology
review, 8(1):77–100.
Bergstra, J., Bardenet, R., Bengio, Y., and K
´
egl, B. (2011).
Algorithms for hyper-parameter optimization. Ad-
vances in neural information processing systems, 24.
Bergstra, J., Yamins, D., and Cox, D. (2013). Making a sci-
ence of model search: Hyperparameter optimization
in hundreds of dimensions for vision architectures. In
International conference on machine learning, pages
115–123. PMLR.
Bollen, J., Gonc¸alves, B., Ruan, G., and Mao, H. (2011).
Happiness is assortative in online social networks. Ar-
tificial life, 17(3):237–251.
Chen, T. and Guestrin, C. (2016). Xgboost: A scalable
tree boosting system. In Proceedings of the 22nd acm
sigkdd international conference on knowledge discov-
ery and data mining, pages 785–794.
Chiu, C. Y., Lane, H. Y., Koh, J. L., and Chen, A. L. (2021).
Multimodal depression detection on instagram con-
sidering time interval of posts. Journal of Intelligent
Information Systems, 56(1):25–47.
De Choudhury, M., Counts, S., and Horvitz, E. (2013a).
Social media as a measurement tool of depression in
populations. In Proceedings of the 5th annual ACM
web science conference, pages 47–56.
De Choudhury, M., Gamon, M., Counts, S., and Horvitz,
E. (2013b). Predicting depression via social media.
In Seventh international AAAI conference on weblogs
and social media.
Ghosh, S. and Anwar, T. (2021). Depression intensity es-
timation via social media: A deep learning approach.
IEEE Transactions on Computational Social Systems.
Ke, G., Meng, Q., Finley, T., Wang, T., Chen, W., Ma, W.,
Ye, Q., and Liu, T.-Y. (2017). Lightgbm: A highly
efficient gradient boosting decision tree. Advances
in neural information processing systems, 30:3146–
3154.
Koltsova, O. Y., Alexeeva, S., and Kolcov, S. (2016). An
opinion word lexicon and a training dataset for russian
sentiment analysis of social media. Computational
Linguistics and Intellectual Technologies: Materials
of DIALOGUE, 2016:277–287.
Kosinski, M., Stillwell, D., and Graepel, T. (2013). Pri-
vate traits and attributes are predictable from digital
records of human behavior. Proceedings of the na-
tional academy of sciences, 110(15):5802–5805.
Lin, H., Jia, J., Guo, Q., Xue, Y., Li, Q., Huang, J., Cai,
L., and Feng, L. (2014a). User-level psychological
stress detection from social media using deep neural
network. In Proceedings of the 22nd ACM interna-
tional conference on Multimedia, pages 507–516.
Lin, T.-Y., Maire, M., Belongie, S., Hays, J., Perona, P.,
Ramanan, D., Doll
´
ar, P., and Zitnick, C. L. (2014b).
Microsoft coco: Common objects in context. In Euro-
pean conference on computer vision, pages 740–755.
Springer.
Losada, D. E., Crestani, F., and Parapar, J. (2017). erisk
2017: Clef lab on early risk prediction on the inter-
net: experimental foundations. In International Con-
ference of the Cross-Language Evaluation Forum for
European Languages, pages 346–360. Springer.
Losada, D. E., Crestani, F., and Parapar, J. (2018).
Overview of erisk: early risk prediction on the inter-
net. In International conference of the cross-language
evaluation forum for european languages, pages 343–
361. Springer.
Lynn, V., Goodman, A., Niederhoffer, K., Loveys, K.,
Resnik, P., and Schwartz, H. A. (2018). Clpsych 2018
shared task: Predicting current and future psycholog-
ical health from childhood essays. In Proceedings of
the Fifth Workshop on Computational Linguistics and
Clinical Psychology: From Keyboard to Clinic, pages
37–46.
Prokhorenkova, L., Gusev, G., Vorobev, A., Dorogush,
A. V., and Gulin, A. (2017). Catboost: unbiased
boosting with categorical features. arXiv preprint
arXiv:1706.09516.
Reece, A. G. and Danforth, C. M. (2017). Instagram pho-
tos reveal predictive markers of depression. EPJ Data
Science, 6:1–12.
Ren, S., He, K., Girshick, R., and Sun, J. (2016). Faster
r-cnn: towards real-time object detection with region
proposal networks. IEEE transactions on pattern
analysis and machine intelligence, 39(6):1137–1149.
Schwartz, H. A., Sap, M., Kern, M. L., Eichstaedt, J. C.,
Kapelner, A., Agrawal, M., Blanco, E., Dziurzynski,
L., Park, G., Stillwell, D., et al. (2016). Predicting
individual well-being through the language of social
media. In Biocomputing 2016: Proceedings of the Pa-
cific Symposium, pages 516–527. World Scientific.
Shelmanov, A. and Smirnov, I. (2014). Methods for seman-
tic role labeling of russian texts. In Computational
Linguistics and Intellectual Technologies. Proceed-
ings of International Conference Dialog, volume 13,
pages 607–620.
Shen, G., Jia, J., Nie, L., Feng, F., Zhang, C., Hu, T.,
Chua, T.-S., and Zhu, W. (2017). Depression detection
Predicting Depression with Text, Image, and Profile Data from Social Media
759