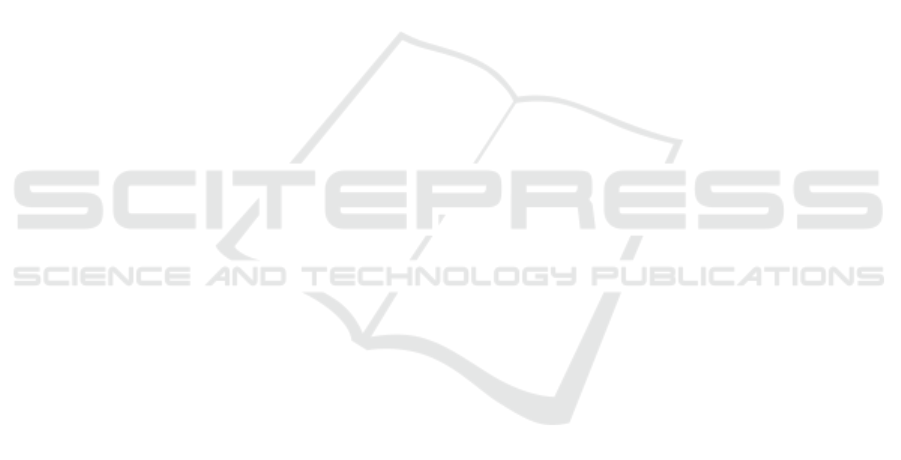
localization. In Proceedings of the IEEE international
conference on computer vision, pages 2938–2946.
Kim, D., Lee, S., Lee, J., and Kim, J. (2020). Leverag-
ing contextual information for monocular depth esti-
mation. IEEE Access, 8:147808–147817.
Kumar, V. R., Hiremath, S. A., Bach, M., Milz, S., Witt,
C., Pinard, C., Yogamani, S., and M
¨
ader, P. (2020).
Fisheyedistancenet: Self-supervised scale-aware dis-
tance estimation using monocular fisheye camera for
autonomous driving. In 2020 IEEE international con-
ference on robotics and automation (ICRA), pages
574–581. IEEE.
Kumar, V. R., Milz, S., Witt, C., Simon, M., Amende,
K., Petzold, J., Yogamani, S., and Pech, T. (2018).
Monocular fisheye camera depth estimation using
sparse lidar supervision. In 2018 21st Interna-
tional Conference on Intelligent Transportation Sys-
tems (ITSC), pages 2853–2858. IEEE.
Li, A., Yuan, Z., Ling, Y., Chi, W., Zhang, C., et al. (2020).
A multi-scale guided cascade hourglass network for
depth completion. In Proceedings of the IEEE/CVF
Winter Conference on Applications of Computer Vi-
sion, pages 32–40.
Lin, J.-T., Dai, D., and Van Gool, L. (2020). Depth estima-
tion from monocular images and sparse radar data. In
2020 IEEE/RSJ International Conference on Intelli-
gent Robots and Systems (IROS), pages 10233–10240.
IEEE.
Liu, F., Shen, C., Lin, G., and Reid, I. (2015). Learning
depth from single monocular images using deep con-
volutional neural fields. IEEE transactions on pat-
tern analysis and machine intelligence, 38(10):2024–
2039.
Ma, F., Cavalheiro, G. V., and Karaman, S. (2019). Self-
supervised sparse-to-dense: Self-supervised depth
completion from lidar and monocular camera. In 2019
International Conference on Robotics and Automation
(ICRA), pages 3288–3295. IEEE.
Park, J., Joo, K., Hu, Z., Liu, C.-K., and So Kweon, I.
(2020). Non-local spatial propagation network for
depth completion. In Computer Vision–ECCV 2020:
16th European Conference, Glasgow, UK, August 23–
28, 2020, Proceedings, Part XIII 16, pages 120–136.
Springer.
Qiu, J., Cui, Z., Zhang, Y., Zhang, X., Liu, S., Zeng, B., and
Pollefeys, M. (2019). Deeplidar: Deep surface normal
guided depth prediction for outdoor scene from sparse
lidar data and single color image. In Proceedings of
the IEEE/CVF Conference on Computer Vision and
Pattern Recognition, pages 3313–3322.
Ronneberger, O., Fischer, P., and Brox, T. (2015). U-net:
Convolutional networks for biomedical image seg-
mentation. In International Conference on Medical
image computing and computer-assisted intervention,
pages 234–241. Springer.
Shivakumar, S. S., Nguyen, T., Miller, I. D., Chen, S. W.,
Kumar, V., and Taylor, C. J. (2019). Dfusenet: Deep
fusion of rgb and sparse depth information for image
guided dense depth completion. In 2019 IEEE In-
telligent Transportation Systems Conference (ITSC),
pages 13–20. IEEE.
Silberman, N., Hoiem, D., Kohli, P., and Fergus, R. (2012).
Indoor segmentation and support inference from rgbd
images. In European conference on computer vision,
pages 746–760. Springer.
Simonyan, K. and Zisserman, A. (2014). Very deep con-
volutional networks for large-scale image recognition.
arXiv preprint arXiv:1409.1556.
Sjafrie, H. (2019). Introduction to Self-Driving Vehicle
Technology. Chapman and Hall/CRC.
Tan, M. and Le, Q. (2019). Efficientnet: Rethinking model
scaling for convolutional neural networks. In Interna-
tional Conference on Machine Learning, pages 6105–
6114. PMLR.
Van Gansbeke, W., Neven, D., De Brabandere, B., and
Van Gool, L. (2019). Sparse and noisy lidar comple-
tion with rgb guidance and uncertainty. In 2019 16th
international conference on machine vision applica-
tions (MVA), pages 1–6. IEEE.
Wang, X., Girshick, R., Gupta, A., and He, K. (2018). Non-
local neural networks. In Proceedings of the IEEE
conference on computer vision and pattern recogni-
tion, pages 7794–7803.
Watson, J., Mac Aodha, O., Prisacariu, V., Brostow, G., and
Firman, M. (2021). The temporal opportunist: Self-
supervised multi-frame monocular depth. In Proceed-
ings of the IEEE/CVF Conference on Computer Vision
and Pattern Recognition, pages 1164–1174.
Wiltschko, T. et al. (2019). Safety first for automated driv-
ing. Technical report, Daimler AG.
Wong, A., Fei, X., Tsuei, S., and Soatto, S. (2020).
Unsupervised depth completion from visual inertial
odometry. IEEE Robotics and Automation Letters,
5(2):1899–1906.
Xiaogang, R., Wenjing, Y., Jing, H., Peiyuan, G., and Wei,
G. (2020). Monocular depth estimation based on deep
learning: A survey. In 2020 Chinese Automation
Congress (CAC), pages 2436–2440. IEEE.
Xu, Y., Zhu, X., Shi, J., Zhang, G., Bao, H., and Li,
H. (2019). Depth completion from sparse lidar data
with depth-normal constraints. In Proceedings of the
IEEE/CVF International Conference on Computer Vi-
sion, pages 2811–2820.
Zhang, Y., Nguyen, T., Miller, I. D., Shivakumar, S. S.,
Chen, S., Taylor, C. J., and Kumar, V. (2019).
Dfinenet: Ego-motion estimation and depth refine-
ment from sparse, noisy depth input with rgb guid-
ance. arXiv preprint arXiv:1903.06397.
Zhao, C., Sun, Q., Zhang, C., Tang, Y., and Qian, F. (2020).
Monocular depth estimation based on deep learning:
An overview. Science China Technological Sciences,
pages 1–16.
Towards Depth Perception from Noisy Camera based Sensors for Autonomous Driving
207