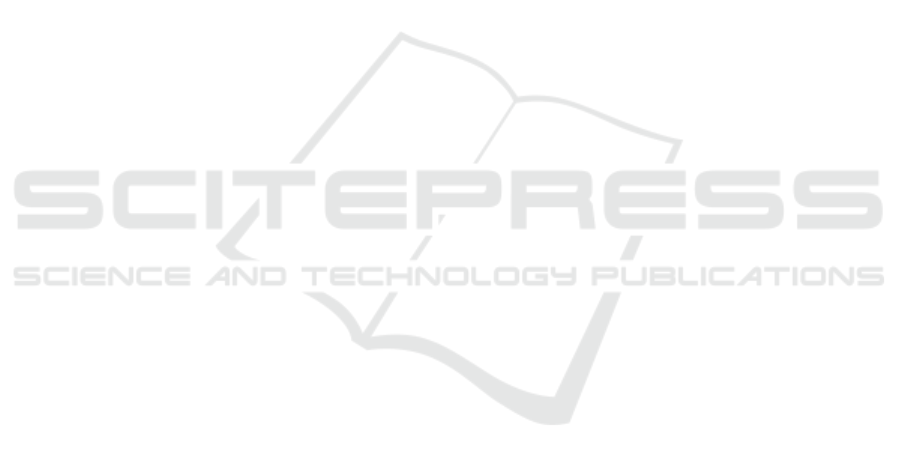
ing PP’s. This may happen because in this process the
acoustic characteristics of a voice are more influential.
Therefore, the Known Speaker approach obtains bet-
ter results because the model trains with all the voices
available in the dataset learning their acoustic charac-
teristics.
As can be seen in the Table 4c, the voice con-
version method improves the performance of models
when detecting mispronunciation. When we trained
the model with only 554 synthetic audios the accuracy
metric increased 2.8%. However, when using the full
set of synthetic audios as in the Table 4, the perfor-
mance increased by 26.4%.
5 CONCLUSIONS AND
PERSPECTIVES
Thanks to the usage of a transfer-learnt AlexNet, we
were able to develop a method to detect mispronunci-
ation in Spanish as well as identify phonological pro-
cesses with 93.4% and 99.1% respectively.
While language-learning apps like Duolingo,
Babbel also employ classification methods to deter-
mine whether a word is partially or fully mispro-
nounced (de la Cal Rioja, 2016). We carry out a sec-
ond classification to determine the type of phonologi-
cal process present in the misspronunciation.
Furthermore, machine and deep learning tech-
niques are being used for various voice recognition
tasks (e.g., query by humming (Alfaro-Paredes et al.,
2021)), that could lead us to improvements in our ap-
proach. Additionally, text formalization (de Rivero
et al., 2021) could be applied for educational purposes
in compliment with our proposal.
For future works, we would like to have the sup-
port of a speech and language therapist who will help
us with the construction of a data set that includes
as many possible mispronunciation scenarios. In this
way, we reduce the probability that the models do not
know how to classify a new audio instance.
REFERENCES
Alfaro-Paredes, E., Alfaro-Carrasco, L., and Ugarte, W.
(2021). Query by humming for song identification us-
ing voice isolation. In IEA/AIE.
Cappellari, V. M. and Cielo, C. A. (2008). Vocal acoustic
characteristics in pre-school aged children. Brazilian
Journal of Otorhinolaryngology, 74(2).
Charney, S. A., Camarata, S. M., and Chern, A. (2021). Po-
tential impact of the covid-19 pandemic on communi-
cation and language skills in children. Otolaryngol-
ogy–Head and Neck Surgery, 165(1).
Coloma, C. J., Pavez, M. M., Maggiolo, M., and Pe
˜
naloza,
C. (2010). Desarrollo fonol
´
ogico en ni
˜
nos de 3 y 4
a
˜
nos seg
´
un la fonolog
´
ıa natural: Incidencia de la edad
y del g
´
enero. Revista signos, 43(72).
de la Cal Rioja, J. (2016). Hound word. software
para la mejora de la pronunciaci
´
on en ingl
´
es.
Universidad de Valladolid - Undergraduate thesis.
https://uvadoc.uva.es/handle/10324/17963.
de Rivero, M., Tirado, C., and Ugarte, W. (2021). For-
malstyler: GPT based model for formal style trans-
fer based on formality and meaning preservation. In
KDIR.
Franciscatto, M. H., Fabro, M. D. D., Lima, J. C. D., Trois,
C., Moro, A., Maran, V., and Soares, M. K. (2021).
Towards a speech therapy support system based on
phonological processes early detection. Comput.
Speech Lang., 65.
Khan, A., Sohail, A., Zahoora, U., and Qureshi, A. S.
(2020). A survey of the recent architectures of deep
convolutional neural networks. Artif. Intell. Rev.,
53(8).
Lu, J., Behbood, V., Hao, P., Zuo, H., Xue, S., and Zhang,
G. (2015). Transfer learning using computational in-
telligence: A survey. Knowl. Based Syst., 80.
Nazir, F., Majeed, M. N., Ghazanfar, M. A., and Maq-
sood, M. (2019). Mispronunciation detection using
deep convolutional neural network features and trans-
fer learning-based model for arabic phonemes. IEEE
Access, 7.
Pavez, M. M. and Coloma, C. J. (2017). Phonological
problems in spanish-speaking children. Advances in
Speech-language Pathology.
Popa, V., Sil
´
en, H., Nurminen, J., and Gabbouj, M. (2012).
Local linear transformation for voice conversion. In
ICASSP. IEEE.
Schuckman, M. (2008). Voice characteristics of preschool
age children. PhD thesis, Miami University.
Shahnawazuddin, S., Adiga, N., Kathania, H. K., and Sai,
B. T. (2020). Creating speaker independent ASR sys-
tem through prosody modification based data augmen-
tation. Pattern Recognit. Lett., 131.
Terbeh, N. and Zrigui, M. (2017). Identification of pro-
nunciation defects in spoken arabic language. In PA-
CLING, volume 781 of Communications in Computer
and Information Science.
Wu, Z. and Li, H. (2014). Voice conversion versus speaker
verification: an overview. APSIPA Transactions on
Signal and Information Processing, 3.
Zhang, L., Wang, S., and Liu, B. (2018). Deep learning for
sentiment analysis: A survey. Wiley Interdiscip. Rev.
Data Min. Knowl. Discov., 8(4).
CSEDU 2022 - 14th International Conference on Computer Supported Education
154