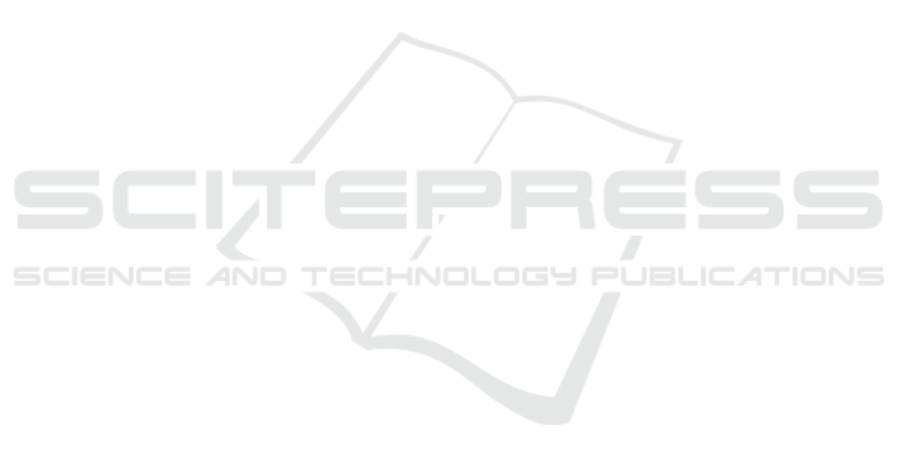
of MovieOcean was perceived as intuitive and
aesthetically pleasing. Only one out of the three
users had a problem finding a movie but also stated
that it could have been because of a typo in the
movie name. The users found it easy to navigate
through their recommendations and find other
movies that were linked as similar movies at the end
of each movie detail view. Moreover, the personality
assessment seemed to reflect what the user thought
about themselves. It was mentioned that the
overview of the personality scores and the short
explanations were received well. Another point is
that the star rating system was a good idea and
brought some life into the recommender system.
5 SUMMARY AND LESSONS
LEARNED
This work explored the inclusion of personality
characteristics as an enhancement of user-user
collaborative filtering algorithms. To this end,
MovieOcean, a personality-based movie
recommender system, was implemented.
MovieOcean uses a questionnaire based on the Big
Five model to generate personality profiles of the
users registered on the platform. With the personality
profiles, personality-based neighborhoods were
created to predict movie ratings and provide
recommendations. Furthermore, to assess how the
consideration of personality traits influences the
recommendations, the RMSE metric was calculated
to analyze the accuracy of the predicted ratings and
the F1-score to evaluate the relevance of the
recommendations for the personality-based and a
standard rating-based approach.
Unfortunately, it was not possible to find
evidence that incorporating personality in
collaborative filtering, in the way it was tested, leads
to better performance. The performance is
significantly worse in terms of the RMSE of the
predicted ratings. However, the F1-scores do not
differ significantly, which makes us think that the
recommendation quality is the same in both
approaches. If this is the case, it is still possible to
suggest using the personality-based approach
because it has several advantages as per our findings:
1. The neighborhoods of personalities can be
precomputed, which leads to a faster
recommendation process.
2. It is possible to use correlations between
personalities and movie genre tastes to overcome
the cold-start problem.
3. Users seem to enjoy the personality-based
approach, as the literature and interviews
conducted for this study showed.
Regarding the research questions defined in
section 1, we conclude that recommender systems
cannot rely more on a personality-based
neighborhood than on a traditional rating-based
neighborhood. However, given the case study results,
one could claim that it is valid to use
personality-based neighborhoods without
deteriorating the quality of the recommendations.
Moreover, only one method to incorporate
personality into recommender systems was explored.
The second question was about the accuracy of
the recommendations. Based on our findings, the
recommendation quality – measured by the F1-score
– does not differ significantly. The significantly
worse RMSE of the personality-based approach
could also influence the recommendations, but this
did not seem to be the case in the conducted
experiments. A slightly better F1-Score was found
for the personality-based approach, when no
additional demographic data was considered.
However, when taking demographic data into
account, the rating-based method had a slightly
higher F1-score. Table 1 shows that in both cases, the
difference was not significant. Suppose the F1-score
is more important than the RMSE of the predicted
ratings. In that case, the authors of this work believe
that it is possible to use a personality-based approach
without worrying about a negative effect on the
recommendation quality. However, based on the
obtained results, there are no improvements either.
In addition, the authors consider that the main
advantage of personality-based recommender
systems is that the neighborhood of similar users can
be precomputed, which results in a faster
recommendation process. In general, personalities
seem to be much more stable than rating vectors, and
we do not think the users would frequently change
the answers to their questionnaire. Even if they
change their answers, they probably only do so a few
times, resulting in a low number of recomputations
compared to the rating neighborhoods, which might
change very often. Moreover, the personality
information can be leveraged to solve the cold-start
recommendation problem by using correlations
between the user personalities and a prototype
personality of users that like movies of a specific
genre. While many users seem to like the
personality-based approach, there are probably also
other users that do not want to fill out the entire
questionnaire before using a recommender system.
In future work, the impact of different kinds of
MovieOcean: Assessment of a Personality-based Recommender System
697