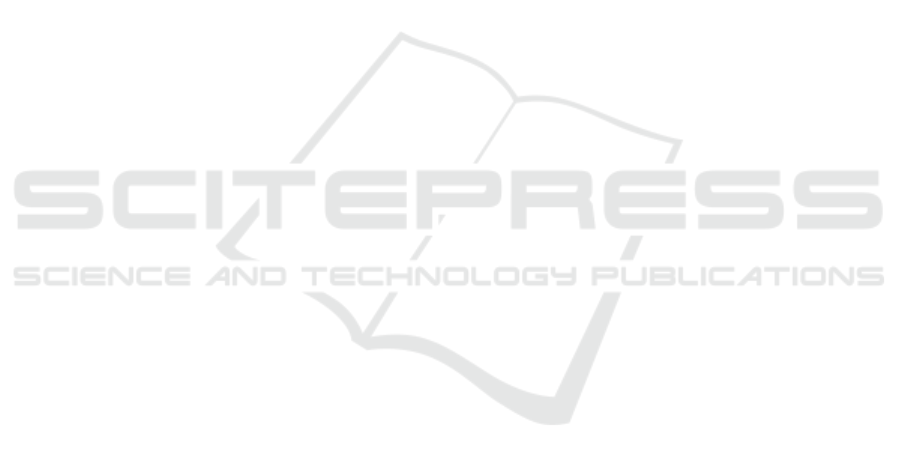
7 ONGOING WORKS
We planned to continue this exploratory study and
(meta-)modeling approach by the following perspec-
tives: i/ considering the low level generation into
the three inter-related parts of the metamodel (do-
main/context/exercise); ii/ taking into account ad-
ditional pedagogical information like feedbacks to
give and post-actions to realize after correct or in-
correct answers; Indeed, gamified learning experi-
ences should have early, frequent, meaningful and
rapid feedback (Faiella and Ricciardi, 2015); iii/ ex-
perimenting the specification of domain models (pro-
cess and tooling) directly by teachers thanks to user-
friendly authoring-tools; iv/ exploring the gaming
facet to generate not only learning exercises but also
gaming activities. Indeed, tailoring gamification is a
current trend in the educational context (Klock et al.,
2020)(Rodrigues et al., 2020).
This last perspective is very important. It will
tackle the need for some new adaptations to learn-
ers’ gaming preferences, based on different gameplay,
game mechanisms, and aesthetics, to identify and de-
sign correctly, in order to better engage and motivate
learners to practice the multiplication exercises.
8 CONCLUSIONS
This article intended to explore how the genera-
tion logic and the underlying elements involved are
expressed from the teachers’ viewpoint in learning
games. First, we conducted an interview-based ex-
ploratory study about the training of times tables. We
related its preparation and analysis. This work led us
to collect many information about a didactic-centered
viewpoint of adaptations to take into account although
no explicit generation rules has been identified. Nev-
ertheless, these information can be used to capture the
didactic facet of the generation.
We then proposed, as a second contribution, a
metamodel specifying all these information. The
metamodel has generic concepts, properties and rela-
tions that can be relevant for other didactic contexts
about declarative knowledge centered. The meta-
model also embeds context-related information about
the times table context. This metamodeling approach
allows to capture invariant informations as well as
generation variants whose semantics can be taken into
account in the generic generation logic by considering
the input models given to the generator.
REFERENCES
B
¨
ockle, M., Novak, J., and Bick, M. (2017). Towards
adaptive gamification: A synthesis of current devel-
opments.
Diwan, C., Srinivasa, S., and Ram, P. (2019). Automatic
Generation of Coherent Learning Pathways for Open
Educational Resources. In Transforming Learning
with Meaningful Technologies, volume 11722, pages
321–334. Springer International Publishing.
Faiella, F. and Ricciardi, M. (2015). Gamification and learn-
ing: a review of issues and research. 11(3):10.
Kim, J. W., Ritter, F. E., and Koubek, R. J. (2013). An in-
tegrated theory for improved skill acquisition and re-
tention in the three stages of learning. 14(1):22–37.
Klock, A. C. T., Gasparini, I., Pimenta, M. S., and Hamari,
J. (2020). Tailored gamification: A review of litera-
ture. 144:102495.
Laforcade, P. (2020). Generation of adapted learning sce-
narios in a serious game: Lessons learnt. In Games
and Learning Alliance, volume 12517, pages 13–23.
Springer International Publishing. Series Title: Lec-
ture Notes in Computer Science.
Laforcade, P. and Laghouaouta, Y. (2019). Generation of
adapted learning game scenarios: A model-driven en-
gineering approach. In Computer Supported Educa-
tion, volume 1022, pages 95–116. Springer Interna-
tional Publishing. Series Title: Communications in
Computer and Information Science.
Mendes, F., Brocardo, J., and Oliveira, H. (2021). Build-
ing Opportunities for Learning Multiplication, pages
241–264. Springer International Publishing.
Rodrigues, L., Toda, A. M., Palomino, P. T., Oliveira, W.,
and Isotani, S. (2020). Personalized gamification:
A literature review of outcomes, experiments, and
approaches. In Eighth International Conference on
Technological Ecosystems for Enhancing Multicultur-
ality, pages 699–706. ACM.
Sehaba, K. and Hussaan, A. M. (2013). GOALS: generator
of adaptive learning scenarios. 8(3):224.
Steinberg, D., Budinsky, F., Paternostro, M., and Merks,
E. (2009). EMF: Eclipse Modeling Framework 2.0.
Addison-Wesley Professional, 2nd edition.
Streicher, A. and Smeddinck, J. D. (2016). Personalized and
Adaptive Serious Games. In D
¨
orner, R., G
¨
obel, S.,
Kickmeier-Rust, M., Masuch, M., and Zweig, K., ed-
itors, Entertainment Computing and Serious Games,
volume 9970. Springer International Publishing. Se-
ries Title: Lecture Notes in Computer Science.
Vandewaetere, M., Desmet, P., and Clarebout, G. (2011).
The contribution of learner characteristics in the de-
velopment of computer-based adaptive learning envi-
ronments. Computers in Human Behavior, 27(1):118–
130.
Wilson, C. and Scott, B. (2017). Adaptive systems in educa-
tion: a review and conceptual unification. The Inter-
national Journal of Information and Learning Tech-
nology, 34(1):2–19.
Expressing Adaptations to Take into Account in Generator-based Exercisers: An Exploratory Study about Multiplication Facts
249