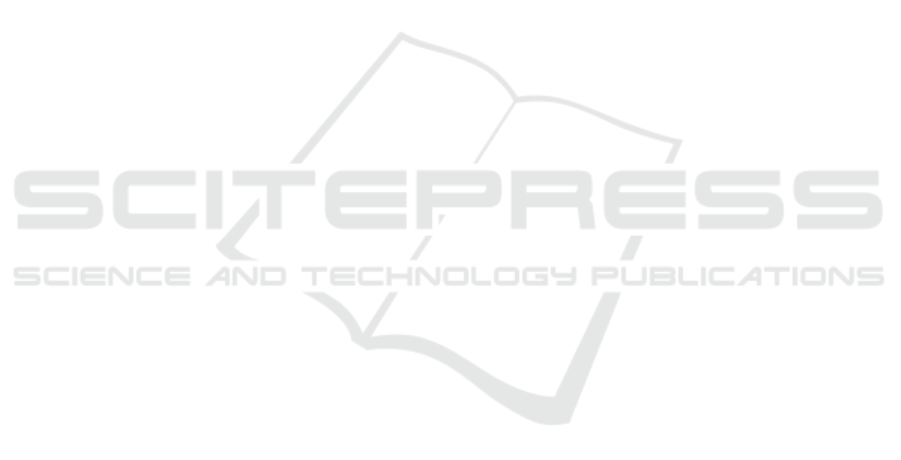
REFERENCES
Aggarwal, C. C. (2015). Data mining: the textbook.
Springer.
Ara, T., M, P., and Bali, M. (2020). Fault prediction in
wireless sensor networks using soft computing. In
2020 International Conference on Smart Technologies
in Computing, Electrical and Electronics (ICSTCEE),
pages 532–538.
Bekkar, M., Djemaa, H. K., and Alitouche, T. A. (2013).
Evaluation measures for models assessment over im-
balanced data sets. J Inf Eng Appl, 3(10).
Bordes, A. and Bottou, L. (2005). The Huller: a simple and
efficient online SVM. In Machine Learning: ECML
2005, Lecture Notes in Artificial Intelligence, LNAI
3720, pages 505–512. Springer Verlag.
Chen, B., Wan, J., Shu, L., et al. (2018). Smart Factory
of Industry 4.0: Key Technologies, Application Case,
and Challenges. IEEE Access, 6:6505–6519.
Chen, T. and Guestrin, C. (2016). XGBoost: A Scalable
Tree Boosting System. In Proceedings of the 22nd
ACM SIGKDD International Conference on Knowl-
edge Discovery and Data Mining, KDD ’16, page
785–794, New York, NY, USA. Association for Com-
puting Machinery.
Choi, M., Park, W.-K., and Lee, I. (2015). Smart office en-
ergy management system using bluetooth low energy
based beacons and a mobile app. In IEEE Interna-
tional Conference on Consumer Electronics (ICCE),
pages 501–502.
Ferri, C., Hern
´
andez-Orallo, J., and Modroiu, R. (2009).
An experimental comparison of performance mea-
sures for classification. Pattern Recognition Letters,
30(1).
Franco da Silva, A. C., Hirmer, P., Schneider, J., et al.
(2020). MBP: Not just an IoT Platform. In IEEE In-
ternational Conference on Pervasive Computing and
Communications Workshops (PerCom Workshops).
Friedman, J. H. (2001). Greedy Function Approximation: A
Gradient Boosting Machine. The Annals of Statistics,
29(5):1189–1232.
Goodfellow, I., Bengio, Y., and Courville, A. (2016). Deep
Learning. MIT Press.
Hastie, T., Tibshirani, R., and Friedman, J. (2009). The el-
ements of statistical learning: data mining, inference,
and prediction. Springer Science & Business Media.
Hossin, M. and M.N, S. (2015). A Review on Evaluation
Metrics for Data Classification Evaluations. Interna-
tional Journal of Data Mining & Knowledge Manage-
ment Process, 5:01–11.
Jiang, F., Sui, Y., and Cao, C. (2013). An incremental de-
cision tree algorithm based on rough sets and its ap-
plication in intrusion detection. Artificial Intelligence
Review, 40(4):517–530.
Ketkar, N. (2017). Stochastic Gradient Descent, pages 113–
132. Apress, Berkeley, CA.
Lian, G., Chen, W., and Huang, S. (2019). Cloud-based on-
line ageing monitoring for iot devices. IEEE Access,
7:135964–135971.
Narasimhan, H., Pan, W., Kar, P., et al. (2016). Optimiz-
ing the Multiclass F-Measure via Biconcave Program-
ming. In IEEE 16th International Conference on Data
Mining (ICDM), pages 1101–1106.
Opitz, J. and Burst, S. (2021). Macro F1 and Macro F1.
Paul, T. and Ueno, K. (2020). Robust Incremental Lo-
gistic Regression for Detection of Anomaly Using
Big Data. In 19th IEEE International Conference on
Machine Learning and Applications (ICMLA), pages
1167–1173.
Rafiuzzaman, M., Gascon-Samson, J., Pattabiraman, K.,
et al. (2019). Failure Prediction in the Internet of
Things Due to Memory Exhaustion. In Proceedings of
the 34th ACM/SIGAPP Symposium on Applied Com-
puting, SAC ’19, page 292–301, New York, USA. As-
sociation for Computing Machinery.
Risteska Stojkoska, B. L. and Trivodaliev, K. V. (2017). A
review of Internet of Things for smart home: Chal-
lenges and solutions. Journal of Cleaner Production,
140:1454–1464.
Saito, T. and Rehmsmeier, M. (2015). The Precision-Recall
Plot Is More Informative than the ROC Plot When
Evaluating Binary Classifiers on Imbalanced Datasets.
PLOS ONE, 10(3):1–21.
Sokolova, M. and Lapalme, G. (2009). A systematic analy-
sis of performance measures for classification tasks.
Information Processing & Management, 45(4):427–
437.
Solanas, A., Patsakis, C., Conti, M., et al. (2014). Smart
health: A context-aware health paradigm within smart
cities. IEEE Communications Magazine, 52(8):74–
81.
Soualhia, M., Fu, C., and Khomh, F. (2019). Infrastructure
Fault Detection and Prediction in Edge Cloud Envi-
ronments. In Proceedings of the 4th ACM/IEEE Sym-
posium on Edge Computing, SEC ’19, page 222–235,
New York, NY, USA. Association for Computing Ma-
chinery.
Su, K., Li, J., and Fu, H. (2011). Smart city and the appli-
cations. In International Conference on Electronics,
Communications and Control (ICECC), pages 1028–
1031.
Suga, N., Yano, K., Webber, J., et al. (2019). Prediction
of QoS Outage Probability for Wireless Communica-
tion in Factory Environments. In International Con-
ference on Internet of Things, Embedded Systems and
Communications (IINTEC), pages 124–129.
Vermesan, O. and Friess, P., editors (2013). Internet of
Things: Converging Technologies for Smart Environ-
ments and Integrated Ecosystems. River Publishers
Series in Communication. River, Aalborg.
Wang, A., Wan, G., Cheng, Z., and Li, S. (2009). An in-
cremental extremely random forest classifier for on-
line learning and tracking. In 16th IEEE International
Conference on Image Processing (ICIP), pages 1449–
1452.
Zhang, T. (2004). Solving Large Scale Linear Prediction
Problems Using Stochastic Gradient Descent Algo-
rithms. In Proceedings of the Twenty-First Interna-
tional Conference on Machine Learning, ICML ’04,
page 116, New York, NY, USA. Association for Com-
puting Machinery.
IoTBDS 2022 - 7th International Conference on Internet of Things, Big Data and Security
148