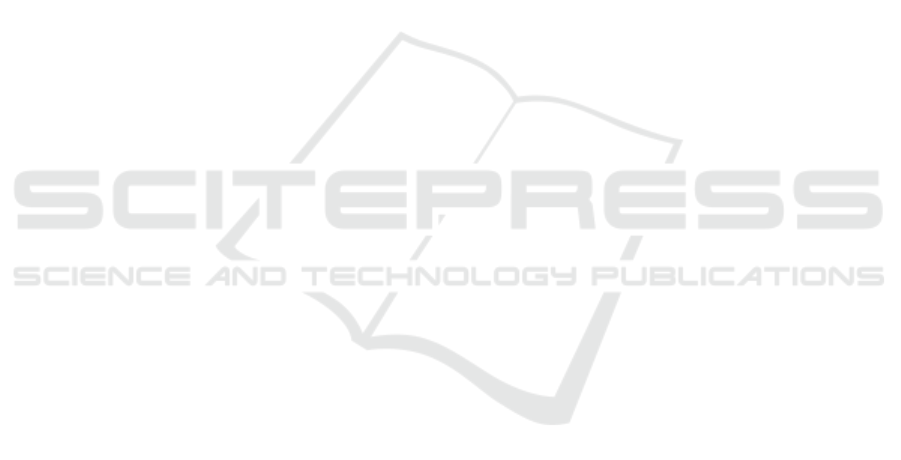
dia data: Social bots, emerging topics, and trends.
JMIR Public Health Surveill, 3(4):e98.
Blei, D. M., Ng, A. Y., and Jordan, M. I. (2003). Latent
dirichlet allocation. the Journal of machine Learning
research, 3:993–1022.
Bolsover, G. and Howard, P. (2019). Chinese computational
propaganda: automation, algorithms and the manipu-
lation of information about chinese politics on twitter
and weibo. Information, Communication & Society,
22(14):2063–2080.
Chan, C.-h. and S
¨
altzer, M. (2020). oolong: An r package
for validating automated content analysis tools. Jour-
nal of Open Source Software, 5:2461.
Coombs, T. and Holladay, J. (2014). How publics react to
crisis communication efforts . Journal of Communi-
cation Management, 18(1):40–57.
Daniel, F. and Millimaggi, A. (2020). On twitter bots behav-
ing badly:a manual and automated analysis of python
code patterns on github. Journal of Web Engineering,
18(8):801–836–801–836.
Davis, C. A., Varol, O., Ferrara, E., Flammini, A., and
Menczer, F. (2016). Botornot: A system to evaluate
social bots. In Proceedings of the 25th International
Conference Companion on World Wide Web, WWW
’16 Companion, page 273–274, Republic and Canton
of Geneva, CHE. International World Wide Web Con-
ferences Steering Committee.
Ferrara, E. (2020). What types of covid-19 conspiracies are
populated by twitter bots? First Monday, 25(6).
Fischer-Preßler, D., Schwemmer, C., and Fischbach, K.
(2019). Collective sense-making in times of crisis:
Connecting terror management theory with twitter
user reactions to the berlin terrorist attack. Comput-
ers in Human Behavior, 100:138–151.
Frandsen, F. and Johansen, W. (2007). Krisekommunika-
tion: N
˚
ar virksomhedens image og omdømme er truet.
Samfundslitteratur.
Frandsen, F. and Johansen, W. (2010). Crisis Communi-
cation, Complexity, and the Cartoon Affair: A Case
Study, chapter 21, pages 425–448. John Wiley &
Sons, Ltd.
Gasc
´
o, M., Bayerl, P. S., Denef, S., and Akhgar, B. (2017).
What do citizens communicate about during crises?
analyzing twitter use during the 2011 uk riots. Gov-
ernment Information Quarterly, 34(4):635–645.
Giglietto, F. and Lee, Y. (2017). A hashtag worth a thousand
words: Discursive strategies around #jenesuispaschar-
lie after the 2015 charlie hebdo shooting. Social Me-
dia + Society, 3(1):2056305116686992.
Greenberg, J., Pyszczynski, T., and Solomon, S. (1986).
The Causes and Consequences of a Need for Self-
Esteem: A Terror Management Theory, pages 189–
212. Springer New York, New York, NY.
Grimmer, J. and Stewart, B. M. (2013). Text as data:
The promise and pitfalls of automatic content anal-
ysis methods for political texts. Political Analysis,
21(3):267–297.
Khaund, T., Al-Khateeb, S., Tokdemir, S., and Agarwal,
N. (2018). Analyzing social bots and their coor-
dination during natural disasters. In Thomson, R.,
Dancy, C., Hyder, A., and Bisgin, H., editors, Social,
Cultural, and Behavioral Modeling, pages 207–212,
Cham. Springer International Publishing.
Kiwan, N. (2016). Freedom of thought in the aftermath of
the charlie hebdo attacks. French Cultural Studies,
27(3):233–244.
Ku
ˇ
sen, E. and Strembeck, M. (2018). Why so emotional?
an analysis of emotional bot-generated content on
twitter. In Proceedings of the 3rd International Con-
ference on Complexity, Future Information Systems
and Risk - COMPLEXIS,, pages 13–22. INSTICC,
SciTePress.
Ku
ˇ
sen, E. and Strembeck, M. (2019). Something draws
near, I can feel it: An analysis of human and bot
emotion-exchange motifs on Twitter. Online Social
Networks and Media, 10-11.
Ku
ˇ
sen, E. and Strembeck, M. (2020). You talkin’ to me?
Exploring Human/Bot Communication Patterns dur-
ing Riot Events. Information Processing & Manage-
ment, 57(1).
Ku
ˇ
sen, E. and Strembeck, M. (2021a). Building blocks of
communication networks in times of crises: Emotion-
exchange motifs. Computers in Human Behavior,
123.
Ku
ˇ
sen, E. and Strembeck, M. (2021b). Emotional Com-
munication During Crisis Events: Mining Structural
OSN Patterns. IEEE Internet Computing, 25(02):58–
65.
Nerghes, A. and Lee, J.-S. (2019). Narratives of the refugee
crisis: A comparative study of mainstream-media and
twitter. Media and Communication, 7(2):275–288.
Petrovic, S., Osborne, M., McCreadie, R., Macdonald, C.,
Ounis, I., and Shrimpton, L. (2021). Can twitter re-
place newswire for breaking news? Proceedings of
the International AAAI Conference on Web and Social
Media, 7(1):713–716.
Ramirez, E. H., Brena, R., Magatti, D., and Stella, F.
(2012). Topic model validation. Neurocomputing,
76(1):125–133. Seventh International Symposium on
Neural Networks (ISNN 2010) Advances in Web In-
telligence.
Rauchfleisch, A. and Kaiser, J. (2020). The false positive
problem of automatic bot detection in social science
research. PLOS ONE, 15(10):1–20.
Roberts, M. E., Stewart, B. M., and Tingley, D. (2019). stm:
An r package for structural topic models. Journal of
Statistical Software, 91(2):1–40.
Sasaki, K., Yoshikawa, T., and Furuhashi, T. (2014). Online
topic model for Twitter considering dynamics of user
interests and topic trends. In Proceedings of the 2014
Conference on Empirical Methods in Natural Lan-
guage Processing (EMNLP), pages 1977–1985, Doha,
Qatar. Association for Computational Linguistics.
Schuchard, R., Crooks, A., Stefanidis, A., and Croitoru, A.
(2019). Bots fired: examining social bot evidence in
online mass shooting conversations. Palgrave Com-
munications, 5(1):158.
Seeger, M. and Sellnow, T. L. (2016). Narratives of crisis:
Telling stories of ruin and renewal. Stanford Univer-
sity Press.
COMPLEXIS 2022 - 7th International Conference on Complexity, Future Information Systems and Risk
22