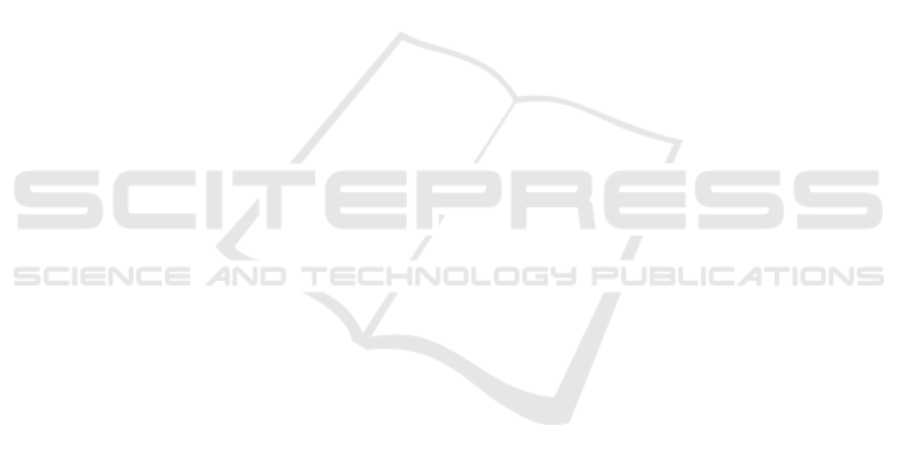
also including faster and more effective contact with
a specialist. Thanks to the validation strategy that was
based on desirability, feasibility, usability and satis-
faction, it allowed us to identify that young Peruvians
are willing to use technological tools in order to be
supported to improve their mental health, as well as
mental health specialists identify a great opportunity
to improve in this sector.
A technological solution to monitor the depres-
sive state of a patient by analyzing social media posts
in order to monitor the signs of depressive symp-
toms that a patient is going through by analyzing their
daily posts on their social media to obtain the evo-
lution of the chronicity of symptoms in each time
range. Evenmore, using Genetic information to seek
for historical data about a patient depression (Arroyo-
Mari
˜
nos et al., 2021) or monitoring symptoms with a
technological solution similar to other disease (Jorge-
L
´
evano et al., 2021).
Preventive model to address suicidal depressive
episodes with the help of a virtual assistant that seeks
to prevent suicidal ideas caused by severe episodes of
depression using strategies that promote positive cop-
ing in people with the help of virtual assistants. Since
it is considered that a depressive episode can occur
at any moment in an individual’s life, it is planned
to develop a virtual assistant that can accompany and
provide mental health support in severe episodes of
depression, and that this allows to recommend or con-
tact directly to a mental health professional after the
level of depression subsides.
REFERENCES
Arroyo-Mari
˜
nos, J. C., Mejia-Valle, K. M., and Ugarte, W.
(2021). Technological model for the protection of ge-
netic information using blockchain technology in the
private health sector. In ICT4AWE.
Cummins, N., Baird, A., and Schuller, B. W. (2018). Speech
analysis for health: Current state-of-the-art and the in-
creasing impact of deep learning. Methods, 151.
Faisol, M., Ramlan, S. A., Hafizah, A., Mozi, A., and Za-
karia, F. F. (2021). Mobile-based speech recognition
for early reading assistant. Journal of Physics: Con-
ference Series, 1962.
Graham, S., Depp, C., Lee, E., Nebeker, C., Tu, X., Kim,
H.-C., and Jeste, D. (2019). Artificial intelligence for
mental health and mental illnesses: an overview. Cur-
rent Psychiatry Reports, 21.
Jorge-L
´
evano, K., Cuya-Chumbile, V., and Ugarte, W.
(2021). Technological solution to optimize the
alzheimer’s disease monitoring process, in metropoli-
tan lima, using the internet of things. In ICT4AWE.
Khanal, S. R., Reis, A., Barroso, J., and Filipe, V. (2018).
Using emotion recognition in intelligent interface de-
sign for elderly care. In WorldCIST, volume 746 of
Advances in Intelligent Systems and Computing.
Khasawneh, T. N., AL-Sahlee, M. H., and Safia, A. A.
(2020). Sql, newsql, and nosql databases: A com-
parative survey. In ICICS.
Li, C., Wei, W., Li, J., and Song, W. (2017). A cloud-based
monitoring system via face recognition using gabor
and CS-LBP features. J. Supercomput., 73(4).
Li, X., Yu, H., Yang, W., Mo, Q., Yang, Z., Wen, S., Zhao,
F., Zhao, W., Tang, Y., Ma, L., Zeng, R., Zou, X., and
Lin, H. (2021). Depression and anxiety among quar-
antined people, community workers, medical staff,
and general population in the early stage of covid-19
epidemic. Frontiers in Psychology, 12.
Macciotta-Felices, B., Moron-Corales, C., Luna-Matos, M.,
Gonzales-Madrid, V., Melgarejo-Moreno, A., Zafra-
Tanaka, J. H., Goicochea-Lugo, S., Martinez-Rivera,
R. N., Nieto-Gutierrez, W., Fiestas-Saldarriaga, F.,
Taype-Rondan, A., Timana-Ruiz, R., and Garavito-
Farro, H. (2020). Clinical practice guideline for the
screening and management of the mild depressive
episode at the first level of care for the peruvian so-
cial security (essalud). ACTA MEDICA PERUANA,
37(4).
Ralston, K., Chen, Y., Isah, H., and Zulkernine, F. H.
(2019). A voice interactive multilingual student sup-
port system using IBM watson. In IEEE ICMLA.
Simcock, G., McLoughlin, L. T., Regt, T. D., Broadhouse,
K. M., Beaudequin, D. A., Lagopoulos, J., and Her-
mens, D. F. (2020). Associations between facial emo-
tion recognition and mental health in early adoles-
cence. International Journal of Environmental Re-
search and Public Health, 17.
Villarreal-Zegarra, D., Cabrera-Alva, M., Carrillo-Larco,
R. M., and Bernabe-Ortiz, A. (2020). Trends in the
prevalence and treatment of depressive symptoms in
peru: a population-based study. BMJ Open, 10(7).
Williamson, J. R., Young, D., Nierenberg, A. A., Niemi,
J., Helfer, B. S., and Quatieri, T. F. (2019). Track-
ing depression severity from audio and video based
on speech articulatory coordination. Comput. Speech
Lang., 55.
Zeghari, R., K
¨
onig, A., Guerchouche, R., Sharma, G.,
Joshi, J., Fabre, R., Robert, P., and Manera, V. (2021).
Correlations between facial expressivity and apathy
in elderly people with neurocognitive disorders: Ex-
ploratory study. JMIR Form Res, 5(3).
Zhang, Y., Yang, Z., Lu, H., Zhou, X., Phillips, P., Liu,
Q., and Wang, S. (2016). Facial emotion recognition
based on biorthogonal wavelet entropy, fuzzy support
vector machine, and stratified cross validation. IEEE
Access, 4.
ICT4AWE 2022 - 8th International Conference on Information and Communication Technologies for Ageing Well and e-Health
36