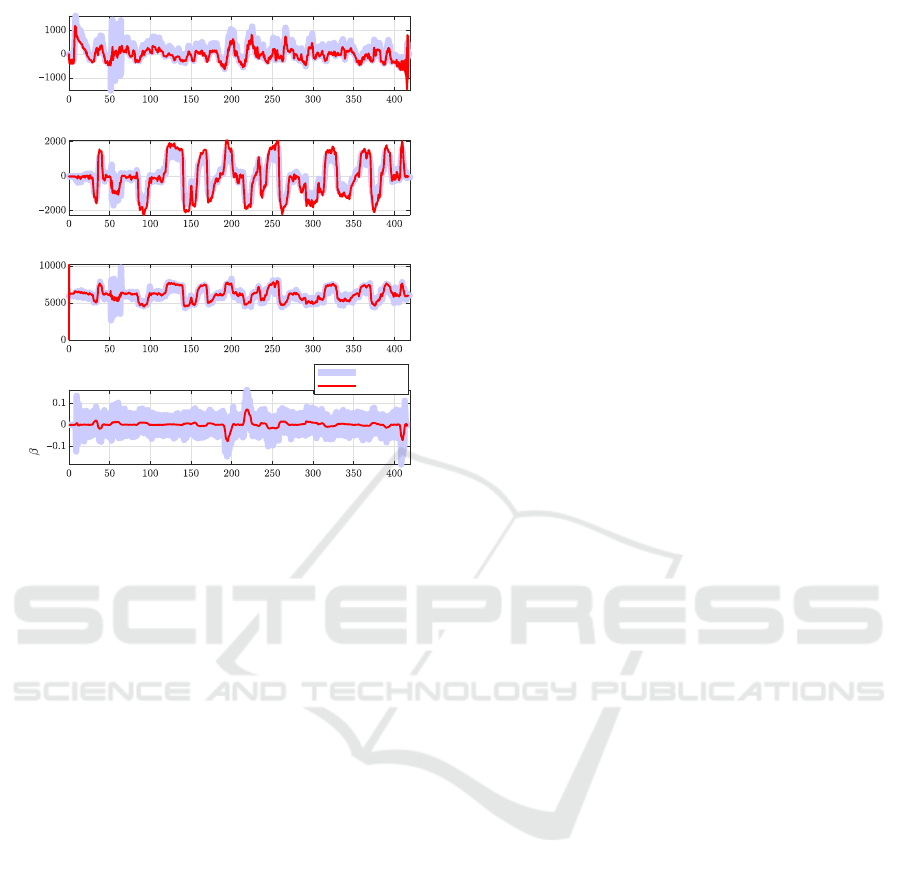
Time [s]
F
x,rl
[N]
Rear Left Longitudinal Tire Force
Time [s]
F
y,rl
[N]
Rear Left Lateral Tire Force
Time [s]
F
z,rl
[N]
Rear Left Vertical Tire Force
Time [s]
COG
[rad]
Vehicle Sideslip Angle
measured
simulated
Figure 9: Estimation results for the rear left wheel forces
and the vehicle sideslip angle.
objective estimators, one can observe that the sensors
used for both tire force and sideslip angle estimation
are mostly identical which is confirmed by the sensor
selection results on figure 6. This approach is able to
combine both quantities of interest in a single estima-
tor and additionally provides improved performance
due to the coupled dynamic nature of the model.
5 CONCLUSIONS
In this work, a novel, multi-objective, automotive
state estimator has been developed featuring a system-
level, non-linear vehicle model. As estimators us-
ing more complex models typically face more issues
towards stability, an extensive observability analysis
was performed. It is shown that unobservable states
can be detected using a Singular Value Decomposi-
tion of the total observability matrix and that dynamic
model coupling greatly determines the required sen-
sors to obtain an observable estimator. Using an ob-
servable projection defined in previous work, this pa-
per proves that it is possible to stabilize the estimator
without GPS measurements if they are independent
from the quantities of interest due to their decoupled
nature. Finally, the estimator has been experimentally
validated on an engineering vehicle case and proved
to be able to accurately track all quantities of interest
with a minimal sensor set.
ACKNOWLEDGEMENTS
This research was partially supported by Flanders
Make, the strategic research centre for the manu-
facturing industry. The Flanders Innovation & En-
trepreneurship Agency within the IMPROVED and
MULTISENSOR project is also gratefully acknowl-
edged for its support. Internal Funds KU Leuven are
gratefully acknowledged for their support.
REFERENCES
Albinsson, A., Bruzelius, F., Jonasson, M., and Jacobson,
B. (2014). Tire force estimation utilizing wheel torque
measurements and validation in simulations and ex-
periments. In 12th International Symposium on Ad-
vanced Vehicle Control, pages 294–299.
Devos, T., Kirchner, M., Croes, J., Desmet, W., and Naets,
F. (2021). Sensor selection and state estimation for
unobservable and non-linear system models. Sensors,
21(22).
Kim, D., Min, K., Kim, H., and Huh, K. (2020). Vehicle
sideslip angle estimation using deep ensemble-based
adaptive kalman filter. Mechanical Systems and Signal
Processing, 144:106862.
Naets, F., van Aalst, S., Boulkroune, B., Ghouti, N. E., and
Desmet, W. (2017). Design and experimental vali-
dation of a stable two-stage estimator for automotive
sideslip angle and tire parameters. IEEE Transactions
on Vehicular Technology, 66(11):9727–9742.
Nakamura, W., Hashimoto, T., and Chen, L.-K. (2020).
State estimation method based on unscented kalman
filter for vehicle nonlinear dynamics. International
Journal of Electrical and Information Engineering,
14(12):435 – 438.
Reif, K., Renner, K., and Saeger, M. (2007). Vehicle
state estimation on the basis of a non-linear two-track
model. ATZ worldwide, 109:33–36.
Svendenius, J. and Wittenmark, B. (2003). Brush tire model
with increased flexibility. In European Control Con-
ference, pages 108–115.
Van Aalst, S., Naets., F., Boulkroune, B., De Nijs, W., and
Desmet, W. (2018). An adaptive vehicle sideslip es-
timator for reliable estimation in low and high excita-
tion driving. In 15th IFAC Symposium on Control in
Transportation Systems, volume 51, pages 243–248.
Vaseur, C., van Aalst, S., and Desmet, W. (2020). Vehicle
state and tire force estimation: Performance analysis
of pre and post sensor additions. In 2020 IEEE Intel-
ligent Vehicles Symposium (IV), pages 1615–1620.
Wang, R. and Wang, J. (2013). Tire–road friction coeffi-
cient and tire cornering stiffness estimation based on
longitudinal tire force difference generation. Control
Engineering Practice, 21(1):65–75.
Yang, C., Blasch, E., and Douville, P. (2010). Design of
schmidt-kalman filter for target tracking with naviga-
tion errors. In IEEE Aerospace Conference Proceed-
ings, pages 1–12.
VEHITS 2022 - 8th International Conference on Vehicle Technology and Intelligent Transport Systems
280