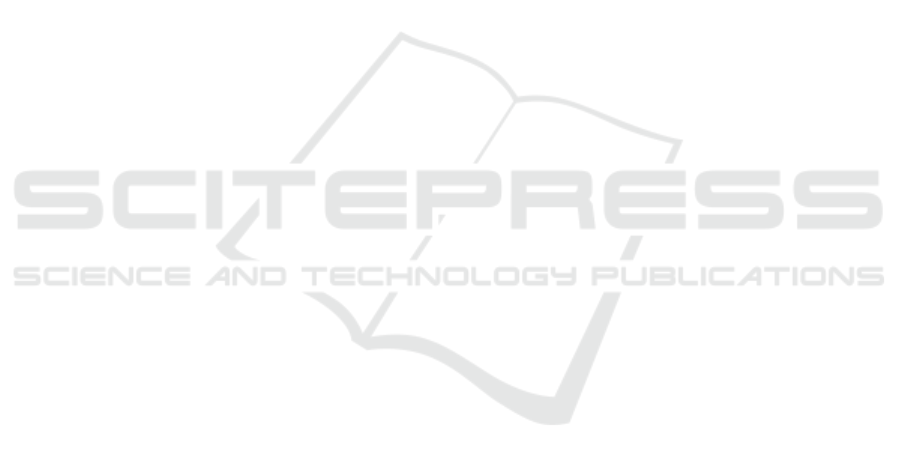
several statistical improvements (Q2). It started by
giving a formal problem description of digital device
and its definition related to mathematics, some re-
lated literature was discussed in which the more re-
cent studies of the impact of instructional computer
use in school settings has found mixed results and
vary across countries (Eickelmann et al., 2012; Hu
et al., 2018; Carstens et al., 2021), while further stud-
ies on the Internet connection and a Personal com-
puter at home shown positive effects on school per-
formance (Wittwer and Senkbeil, 2008). It is not sim-
ply having access to an ICT at home or in school that
can influence in positive terms school learning (which
instead would provide negative outcomes) but rather
the relative ability to use and control it. From the
review of the international literature, about the rela-
tionship between digital learning and learning in the
classroom, the students have greater difficulty using
the computer equipment effectively when teachers do
not work in the classroom to develop shared prac-
tices in the use of technology (Kozma, 2003; Balan-
skat et al., 2006; Burns, 2013; Drijvers, 2015; Bray
and Tangney, 2017; Viberg et al., 2020). According
to the research question Q1, confirmed from the ma-
chine learning models, the most important predictors
for mathematics education at the classroom level are
the ESCS, both at individual and classroom level, the
Personal Computer ownership at home, the language
is spoken at home (Italian), the belonging to a class-
room of Southern Italy (maybe in a pejorative con-
notation), the math score at grade 7, both at individ-
ual and class level. Here, Information and Commu-
nications Technology plays a part in the value-added
approach, specifically in terms of Personal Computer
ownership at home or belonging to a class with high
Personal Computer ownership at home. However,
the role of Information and Communication Tech-
nologies is a minor part compared to the ascribed
characteristics. Indeed, it can occasionally discour-
age students’ trouble and logical thinking if educa-
tion systems don’t borrow technology to meet their
tutoring needs. Therefore, it is essential to develop
a more articulated model in the Italian context, pos-
sibly taking cues from international efforts (Fraillon
et al., 2014), which discusses self-efficacy and the
use of Information and Communication Technology
also in the classroom setting. In addition, the value-
added score analysis suggests that technology-based
professional development by teachers is not so es-
sential. However, for a future where technologies
are crucial, school principals cannot ignore their im-
portance (Karakose et al., 2021). The capability to
break fine problems with group conditioning in the
school class tends to induce a disadvantage in the per-
formance of the individual test. The calculation test
score gap between first-generation immigrants, na-
tives and second-generation emigrants has verified the
OECD framework (Pe
˜
na-L
´
opez et al., 2017)) that’s
the language walls the key to explaining differences in
performance between these two groups of students, as
well as a major disadvantage in terms of implicit per-
ceptivity with access from home to a particular com-
puter. It remains to be explored how peer collabora-
tion activities are structured and how they interact in
the use of new information technologies. Recent stud-
ies show that the effectiveness of peer group activities
is strongly correlated with the strategies introduced
by the teacher to make it work, such as role assign-
ment, group contracts, anonymous peer assessments,
etc. (Chang and Brickman, 2018). Finally, the multi-
level hierarchical model is fully superimposable with
machine learning models that are recommended for
ranking added value. It should therefore be noted that
if a hierarchical model is not applicable, and there are
many other applications, the Extreme Gradient Boost-
ing models and the Random Forest could prove com-
plementary to hierarchical analysis to discover and
test complex relationships between variables in the
educative field also exploiting their predictive poten-
tial. However, it must be pointed out that it is still
challenging, especially for concepts such as value-
added scores in classes, where the goal is still to in-
terpret and know in depth what are the factors that
influence the effectiveness of one class rather than an-
other, to use machine learning models without a con-
solidated conceptual scheme.
REFERENCES
Balanskat, A., Blamire, R., and Kefala, S. (2006). The ict
impact report. European Schoolnet, 1:1–71.
Bray, A. and Tangney, B. (2017). Technology usage in
mathematics education research–a systematic review
of recent trends. Computers & Education, 114:255–
273.
Breiman, L., Friedman, J., Olshen, R., and Stone, C. (1984).
Cart. Classification and Regression Trees; Wadsworth
and Brooks/Cole: Monterey, CA, USA.
Burns, M. (2013). Success, failure or no significant dif-
ference: Charting a course for successful educa-
tional technology integration. International Journal
of Emerging Technologies in Learning, 8(1).
Carstens, K. J., Mallon, J. M., Bataineh, M., and Al-
Bataineh, A. (2021). Effects of technology on stu-
dent learning. Turkish Online Journal of Educational
Technology-TOJET, 20(1):105–113.
Chang, Y. and Brickman, P. (2018). When group work
doesn’t work: Insights from students. CBE—Life Sci-
ences Education, 17(3):ar52.
Digital Device and Mathematics: Multilevel vs Machine Learning Models for Value-added Ranking in Italy
177