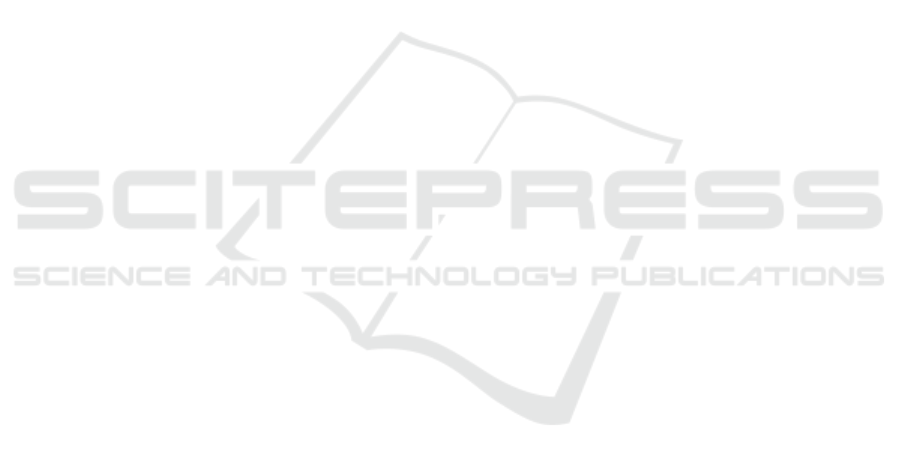
take into account other patient examples with similar
conditions in terms of general symptoms, signs, prog-
noses and progressions (Wiemken et al., 2013).
In this work, we aim to consider in what ways
could a predictive analytical model help to address
inpatient mortality risk problem in CAP cases. To
this end, two aspects should be taken into account
(Pourhomayoun and Shakibi, 2021): (i) the large and
increasing volume of historical patient data, and (ii)
the generation and usage of a model that generalises
beyond the dataset in such a way that it may assist
health professionals to make more assertive decisions
on inpatients treatments. In this scenario, we de-
fine two main research problems that have guided our
work: (i) How to identify elderly inpatients diagnosed
with CAP at risk of death? And, in addition, (ii) how
to provide the probability that such prediction may in-
deed occur?
In this sense, we propose a supervised learning ap-
proach to predict mortality risk with respect to elderly
inpatients with CAP. Based on patients EMR data as
learning features, our approach is able to classify pa-
tients at risk of mortality during hospitalization. In
addition, it can estimate a probability of inpatients
come to death, by means of a range from 50% to 99%
w.r.t. positive classification (patients that do not sur-
vive). The approach uses real world data of elderly
people with CAP from a hospital in Brazil, which
were collected from 2018 to 2021 and prepared for
usage in this work. We evaluate our approach under
two aspects: (i) particularly analysing Receiver Op-
erating Characteristic (ROC) curves, which are used
in medicine to determine diagnostics effectiveness of
classification models, and (ii) by computing ROC’s
Area Under the Curve (AUC), which provides the
overall performance of the most critical classification
in this work (patients classified as at risk of death).
Accomplished results show that the presented ap-
proach outperforms CURB-65 score as baseline both
in terms of AUC and of the obtained probability for
risk of death. Results also bring to attention a time
limit of hospitalization that hugely increased the prob-
ability of death, considering some chronological mea-
surements of inpatients.
Our contributions are summarized as follows: (i) a
relevant dataset built based on different factors corre-
lated to pneumonia, including some features extracted
from medical annotations; (ii) an approach using ma-
chine learning algorithms for analyzing and predict-
ing risk of death in elderly inpatients with CAP; (iii)
a baseline built based on a real medical score (CURB-
65); (iv) a comparative evaluation between the com-
putational version of a baseline and the best achieved
classification model using ROC curves; (v) a statisti-
cal significance test, which confirms that our predic-
tive model outperforms the baseline; and (vi) a data
analysis w.r.t. a patient chronology regarding results
achieved by the best classifier.
This paper is organized as follows: Section 2
provides some theoretical background; Section 3 de-
scribes some related works; Section 4 introduces as-
pects of the research methodology applied in this
work; Section 5 presents the proposed approach with
the experimental evaluation accomplished and results.
Section 6 concludes the paper and points out some fu-
ture work.
2 THEORETICAL BACKGROUND
CAP is a form of intense respiratory infection that af-
fects the lungs. This can lead to symptoms such as
cough and shortness of breath. In severe cases, hospi-
talization is rather recommended (World Health Orga-
nization, 2015)(Long et al., 2017). Particularly, there
are some reasons why CAP can be more severe in
older adults (World Health Organization, 2015): im-
mune system naturally weakens as people age and
older adults are more likely to have chronic health
conditions, such as heart diseases, what can increase
their risk for pneumonia. In order to improve patient
care and management regarding CAP, medical profes-
sionals make use of inpatient risk scores.
A number of pneumonia severity scores have been
described in the literature (Chen et al., 2010)(Long
et al., 2017). Severity scores are important to ascer-
tain, for instance, safety criteria to discharge/admit
patients and time to remain in an Intensive Care Unit
(ICU) (Webb and Gattinoni, 2016). These scores sup-
port clinical decision-making in a variety of scenarios
and can be found in the literature to calculate the prob-
ability of morbidity and mortality among inpatients
with pneumonia. The scores most commonly used are
the CURB-65 and PSI (Long et al., 2017)(Chen et al.,
2010). Both PSI and CURB-65 use data from patient
medical records, such as laboratory results, vital signs
and demographic data, in order to estimate mortality
or even help determining inpatient versus outpatient
treatment. To this end, they provide some categories
of risk, based on the score calculation discussed in the
following (Long et al., 2017)(Chen et al., 2010).
The CURB-65 scores range from 0 to 5 and in-
cludes points for each one of the following criteria,
namely (Webb and Gattinoni, 2016): patient has con-
fusion (defined by a mental test score); blood urea >
20 mg/dL; respiratory rate ≥ 30 breaths/min; blood
pressure (systolic < 90 mm/Hg, or diastolic ≤ 60
mm/Hg) and age ≥ 65 years. Clinical management
Predicting Mortality Risk among Elderly Inpatients with Pneumonia: A Machine Learning Approach
345