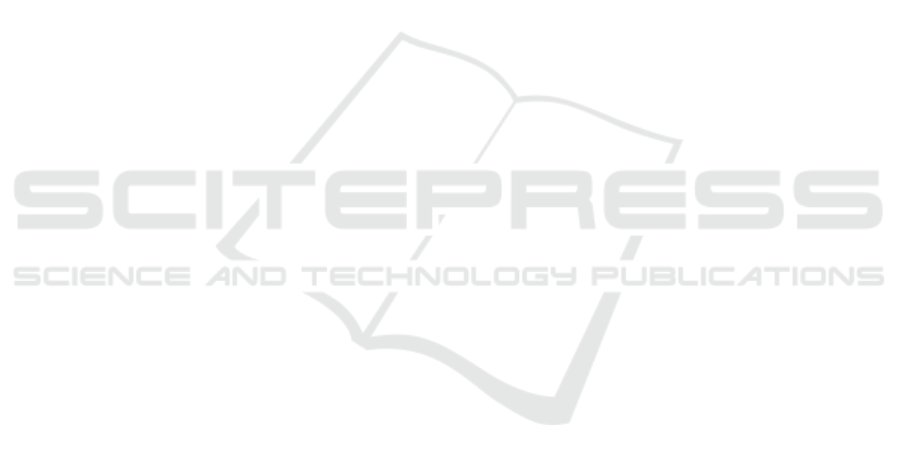
7 CONCLUSIONS
We conducted an online survey with current, former,
and potential users of UBI, analysing their responses
through a qualitative and quantitative analysis.
The results indicate that privacy concerns arise if
driving data are stored and shared. Given the choice,
our participants would rather use self-sufficient UBI
implementations that perform all the analysis locally
and avoid sending data to the insurer or other parties.
We find that participants prioritize saving money
over improving their driving style, when it comes to
one’s perception of UBI utility. It should be noted that
no participants reported any actual savings they have
made via UBI, nor do they know anyone who has.
The issue is exacerbated by the lack of transparency of
the scoring algorithms, which made some participants
conclude that UBI is only meant to benefit insurers.
Therefore, we consider that improving transparency
should be a top priority, otherwise a growing share of
users might be disappointed, thus reducing adoption.
Based on the results, we propose several recom-
mendations for insurers, aimed at increasing UBI ac-
ceptance (see Section 5.1).
ACKNOWLEDGEMENTS
We thank Zinaida Benenson and Freya Gassmann
for their valuable comments on earlier versions of
this paper. This research has received funding from
the H2020 Marie Skłodowska-Curie EU project “Pri-
vacy&Us” under the grant agreement No 675730.
REFERENCES
Arvidsson, S. et al. (2011). Reducing asymmetric informa-
tion with usage-based automobile insurance. Swedish
National Road & Transport Research Institute (VTI),
(2010):2.
Bellatti, J., Brunner, A., Lewis, J., Annadata, P., Eltarjaman,
W., Dewri, R., and Thurimella, R. (2017). Driving
habits data: Location privacy implications and solu-
tions. IEEE Security & Privacy, 15(01):12–20.
Braun, V. and Clarke, V. (2006). Using thematic analysis in
psychology. 3(2):77–101.
Buehler, R. and Pucher, J. (2017). Trends in walking and cy-
cling safety: Recent evidence from high-income coun-
tries, with a focus on the united states and germany.
107(2):281–287.
Derikx, S., De Reuver, M., and Kroesen, M. (2016). Can
privacy concerns for insurance of connected cars be
compensated? Electronic markets, 26(1):73–81.
Dewri, R., Annadata, P., Eltarjaman, W., and Thurimella, R.
(2013). Inferring trip destinations from driving habits
data. In Proceedings of the 12th ACM workshop on
Workshop on privacy in the electronic society, pages
267–272.
Dinev, T. and Hart, P. (2006). An extended privacy calcu-
lus model for e-commerce transactions. Information
systems research, 17(1):61–80.
Gao, X., Firner, B., Sugrim, S., Kaiser-Pendergrast, V.,
Yang, Y., and Lindqvist, J. (2014). Elastic pathing:
Your speed is enough to track you. In Proceedings of
the 2014 ACM International Joint Conference on Per-
vasive and Ubiquitous Computing, pages 975–986.
H
¨
andel, P., Ohlsson, J., Ohlsson, M., Skog, I., and Nygren,
E. (2013). Smartphone-based measurement systems
for road vehicle traffic monitoring and usage-based in-
surance. IEEE systems journal, 8(4):1238–1248.
Iqbal, M. U. and Lim, S. (2006). A privacy preserving gps-
based pay-as-you-drive insurance scheme. In Sympo-
sium on GPS/GNSS (IGNSS2006), pages 17–21.
Kaiser, H. F. and Rice, J. (1974). Little jiffy, mark iv. Edu-
cational and psychological measurement, 34(1):111–
117.
Litman, T. (2007). Distance-based vehicle insurance feasi-
bility, costs and benefits. Victoria, 11.
Mayer, P. (2012). Empirical Investigations on User Percep-
tion and the Effectiveness of Persuasive Technologies.
PhD thesis, University of St. Gallen.
Quintero, J., Railean, A., and Benenson, Z. (2020). Accep-
tance factors of car insurance innovations: The case of
usage-based insurance. Journal of Traffic and Logis-
tics Engineering Vol, 8(2).
SAE J3016 (2018). Taxonomy and Definitions for Terms
Related to Driving Automation Systems for On-Road
Motor Vehicles. Standard, Society of Automotive En-
gineers (SAE).
Soleymanian, M., Weinberg, C. B., and Zhu, T. (2019). Sen-
sor data and behavioral tracking: Does usage-based
auto insurance benefit drivers? Marketing Science,
38(1):21–43.
Taylor, R. (1990). Interpretation of the correlation coeffi-
cient: a basic review. Journal of diagnostic medical
sonography, 6(1):35–39.
Tian, X., Prybutok, V. R., Mirzaei, F. H., and Dinulescu,
C. C. (2020). Millennials acceptance of insurance
telematics: An integrative empirical study. Tian, Xi-
aoguang, pages 156–181.
Troncoso, C., Danezis, G., Kosta, E., Balasch, J., and Pre-
neel, B. (2010). Pripayd: Privacy-friendly pay-as-
you-drive insurance. IEEE Transactions on Depend-
able and Secure Computing, 8(5):742–755.
Wahlstr
¨
om, J., Skog, I., Rodrigues, J. G., H
¨
andel, P., and
Aguiar, A. (2016). Map-aided dead-reckoning—a
study on locational privacy in insurance telematics.
arXiv preprint arXiv:1611.07910.
Warshaw, P. R. and Davis, F. D. (1985). Disentangling be-
havioral intention and behavioral expectation. Journal
of experimental social psychology, 21(3):213–228.
Users’ Privacy Concerns and Attitudes towards Usage-based Insurance: An Empirical Approach
299