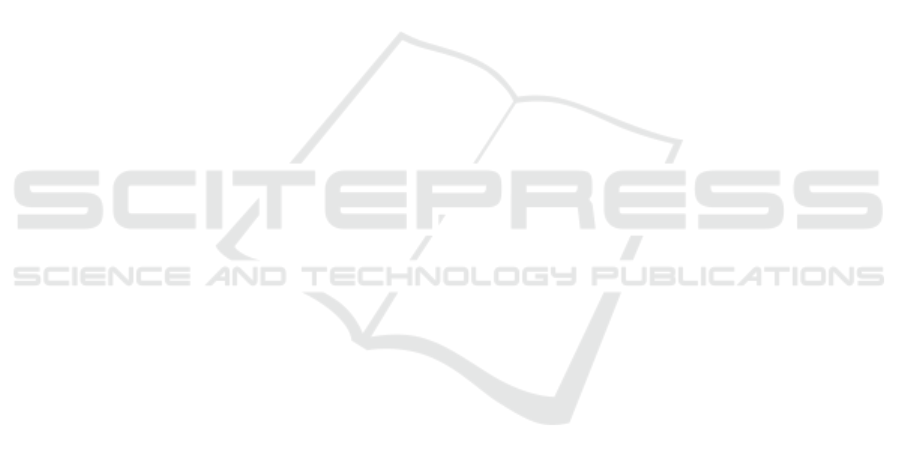
to obtain more interesting insights. Another draw-
back of this work is the omission if multiple-instances
case. One challenge of process mining for artifact-
centric processes is handling with many-to-many re-
lationships between artifacts, which make it diffi-
cult to identify a unique process instance notion to
group related events. Many works have addressed
this problem, from the modeling viewpoint (van der
Aalst et al., 2001; Cohn and Hull, 2009; Lohmann,
2013), or from the process mining algorithm angle
(Schuster et al., 2021; Li et al., 2017; Li et al., 2018).
BAPE adopts a hybrid approach of artifact-centric and
activity-centric, thus does not face exactly the dif-
ficulty of the pure artifact-centric process approach.
Therefore we could still apply the traditional miner al-
gorithms when integrating process mining into BAPE
with the assumption that artifacts are manipulated by
a tasks of the same process instance. However, such
an assumption is not always true, especially for exe-
cution data extracted directly from process actor’s in-
formation systems. There are still more rooms for im-
provement of our works, especially for exploring the
possibility of multiple-instances related to a case and
for investigating other types of anomalies.
REFERENCES
Cohn, D. and Hull, R. (2009). Business artifacts: A data-
centric approach to modeling business operations and
processes. IEEE Data Eng. Bull., 32:3–9.
dos Santos Garcia, C., Meincheim, A., Faria Junior, E. R.,
Dallagassa, M. R., Sato, D. M. V., Carvalho, D. R.,
Santos, E. A. P., and Scalabrin, E. E. (2019). Pro-
cess mining techniques and applications – a system-
atic mapping study. Expert Systems with Applications,
133:260–295.
Ester, M., Kriegel, H.-P., Sander, J., and Xu, X. (1996).
A density-based algorithm for discovering clusters in
large spatial databases with noise. In Proceedings of
the Second International Conference on Knowledge
Discovery and Data Mining, KDD’96, page 226–231.
AAAI Press.
Hajmoosaei, M., Tran, H. N., and Percebois, C. (2017). A
user-centric process management for system and soft-
ware engineering projects. In 7th International Con-
ference on Industrial Engineering and Systems Man-
agement (IESM 2017), pages 123–128, Saarbr
¨
ucken,
Germany.
Jokonowo, B., Claes, J., Sarno, R., and Rochimah, S.
(2018). Process mining in supply chains: A system-
atic literature review. International Journal of Electri-
cal and Computer Engineering, 8:4626–4636.
Kim, K.-S., Pham, D.-L., and Kim, K. P. (2021). p-
algorithm: A sicn-oriented process mining frame-
work. IEEE Access, 9:139852–139875.
Li, G., de Carvalho, R. M., and van der Aalst, W. M. P.
(2017). Automatic discovery of object-centric behav-
ioral constraint models. In Abramowicz, W., editor,
Business Information Systems, pages 43–58, Cham.
Springer International Publishing.
Li, G., de Murillas, E. G. L., de Carvalho, R. M., and van der
Aalst, W. M. P. (2018). Extracting object-centric
event logs to support process mining on databases. In
Mendling, J. and Mouratidis, H., editors, Information
Systems in the Big Data Era, pages 182–199, Cham.
Springer International Publishing.
Lohmann, N. (2013). Compliance by design for artifact-
centric business processes. Information Systems,
38(4):606–618. Special section on BPM 2011 con-
ference.
Pe
˜
na, M. R. and Bayona-Or
´
e, S. (2018). Process mining
and automatic process discovery. In 2018 7th Interna-
tional Conference On Software Process Improvement
(CIMPS), pages 41–46.
Rovani, M., Maggi, F. M., de Leoni, M., and van der Aalst,
W. M. (2015). Declarative process mining in health-
care. Expert Systems with Applications, 42(23):9236–
9251.
Schuster, D., van Zelst, S. J., and van der Aalst, W. M. P.
(2021). Cortado—an interactive tool for data-driven
process discovery and modeling. Lecture Notes in
Computer Science, page 465–475.
Valencia-Parra, A., Ramos-Guti
´
errez, B., Varela-Vaca, A.,
G
´
omez-L
´
opez, M., and Bernal, A. (2019). Enabling
process mining in aircraft manufactures: Extracting
event logs and discovering processes from complex
data. CEUR Workshop Proceedings, 2428:166–177.
van der Aalst, W., Barthelmess, P., Ellis, C., and Wainer, J.
(2001). Proclets: A framework for lightweight inter-
acting workflow processes. International Journal of
Cooperative Information Systems, 10:443–481.
van der Aalst, W., Reijers, H., and Song, M. (2005). Dis-
covering social networks from event logs. Computer
Supported Cooperative Work, 14:549–593.
van der Aalst, W., Weijters, T., and Maruster, L. (2004).
Workflow mining: discovering process models from
event logs. IEEE Transactions on Knowledge and
Data Engineering, 16(9):1128–1142.
van der Aalst, W. M. P. (2016). Process Mining: Data Sci-
ence in Action. Springer, Heidelberg, 2 edition.
van der Aalst, W. M. P., Bolt, A., and van Zelst, S. J. (2017).
Rapidprom: Mine your processes and not just your
data.
Weijters, A., van der Aalst, W., and Medeiros, A. (2006).
Process Mining with the Heuristics Miner-algorithm,
volume 166.
ENASE 2022 - 17th International Conference on Evaluation of Novel Approaches to Software Engineering
370