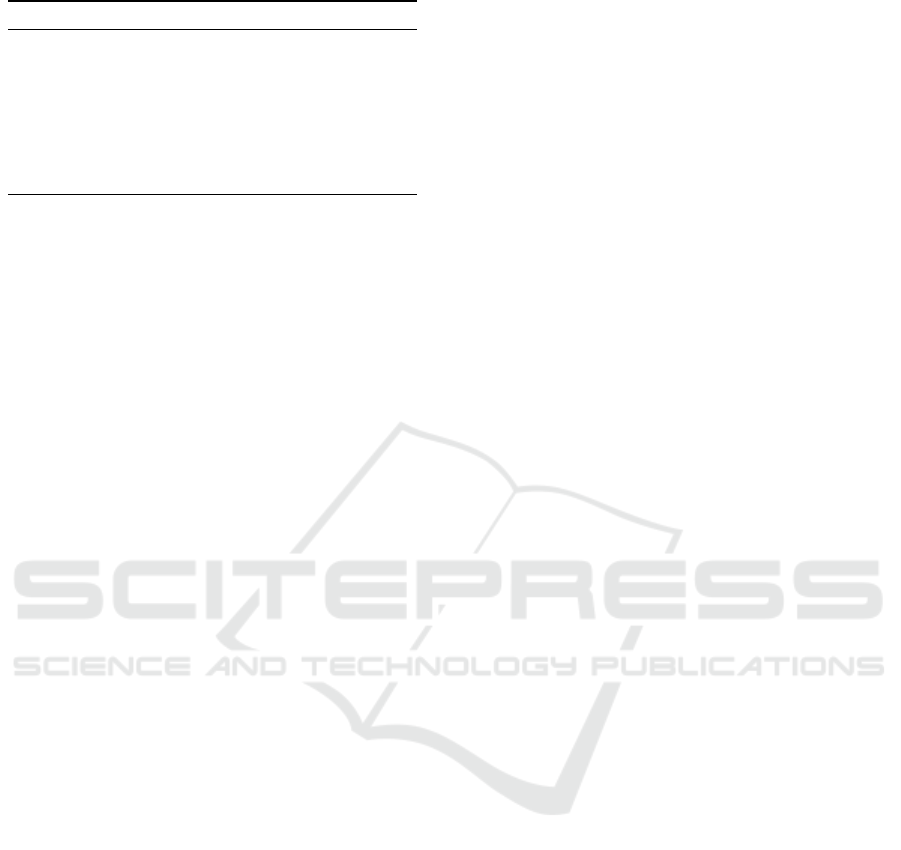
Table 11: Percentage values for query Q
8
.
Reason Type Percentage (%)
reckless driver rear collision 22.0395
pedestrian on the
road
hit a pedestrian on
the road
14.0416
reckless driver side collision 8.4765
reckless driver hit a pedestrian on
the road
5.9364
pedestrian on the
road
run over 4.3538
4.3 Discussion
The Q
1
query allowed us to identify the four districts
in which road sections have a higher probability of fa-
tal vehicle accidents. Based on these results, we carry
out queries Q
2
, Q
3
, Q
4
and Q
5
to infer the probabil-
ities for the reasons and types of accidents that these
fatal accidents occur in these four districts.
The results of the Q
1
query also helped us to de-
velop the last 3 queries, since it allowed us to detect
the section of the Pan-American highway belonging
to the Rimac district as the one with the greatest prob-
ability for fatal vehicle accidents, based on this, we
carry out query Q
6
, to infer the probabilities of the
shifts when these fatal accidents occur in the Rimac
district, resulting in the afternoon.
Based on the result of query Q
6
, we carry out
queries Q
7
and Q
8
that consider vehicular accidents
whose severities are fatal in the Rimac district that
occur in the afternoon, these queries let us identify
the reasons and types of accidents respectively. We
believe that these results will be of great relevance to
carry out preventive actions and thus reduce the num-
ber of these unfortunate accidents.
5 CONCLUSIONS
A Bayesian network was obtained with one of the
highest scores, using the Hill Climbing Search and
Chow Liu algorithm with K2 score- After carrying
out the experiments, it was possible to identify that
very serious accidents occur with a high probability
in the part of the Pan-American Highway belonging
to the Rimac district at the afternoon shift and due to
pedestrian on the road, a reckless driver or speeding.
It is recommended to place a greater police guard
in the part of the Pan-American Highway, belonging
to the Rimac district between 12 and 18 hours and to
carry out awareness campaigns in this area, in order to
reduce the speed of cars and thus reduce the number
of accidents with fatalities. Due to the fact that one of
the factors is speeding, a photo ballot systems with the
respective notice to drivers should be implemented.
Further works can be done with similar analysis of
other critical points in the country, for instance, find
other traffic problems by applying Markov chains,
for example variations in driving patterns, pose esti-
mation for road pedestrians (Fernandez-Ramos et al.,
2021) and looking if car sharing may help to decrease
the traffic accident rate (V
´
asquez-Garaya et al., 2021).
REFERENCES
Chow, C. and Liu, C. (1968). Approximating discrete
probability distributions with dependence trees. IEEE
Transactions on Information Theory, 14(3).
Cooper, G. F. and Herskovits, E. (1992). A bayesian method
for the induction of probabilistic networks from data.
Mach. Learn., 9:309–347.
Deublein, M., Schubert, M., Adey, B. T., and Garc
´
ıa de
Soto, B. (2015). A bayesian network model to pre-
dict accidents on swiss highways. Infrastructure Asset
Management, 2(4).
Fernandez-Ramos, O., Johnson-Ya
˜
nez, D., and Ugarte, W.
(2021). Reproducing arm movements based on pose
estimation with robot programming by demonstration.
In IEEE ICTAI.
Heckerman, D., Geiger, D., and Chickering, D. M.
(1995). Learning bayesian networks: The combina-
tion of knowledge and statistical data. Mach. Learn.,
20(3):197–243.
Hongguo, X., Huiyong, Z., and Fang, Z. (2010). Bayesian
network-based road traffic accident causality analysis.
In WASE ICIE.
Liu, Z., Malone, B. M., and Yuan, C. (2012). Empirical
evaluation of scoring functions for bayesian network
model selection. BMC Bioinform., 13(S-15):S14.
Makaba, T., Doorsamy, W., and Paul, B. S. (2021).
Bayesian network-based framework for cost-
implication assessment of road traffic collisions. Int.
J. Intell. Transp. Syst. Res., 19(1):240–253.
Riggelsen, C. (2008). Approximation Methods for Efficient
Learning of Bayesian Networks, volume 168 of Fron-
tiers in Artificial Intelligence and Applications. IOS
Press.
Russell, S. J. and Norvig, P. (2020). Artificial Intelligence:
A Modern Approach (4th Edition). Pearson.
Schwarz, G. (1978). Estimating the Dimension of a Model.
The Annals of Statistics, 6(2):461 – 464.
V
´
asquez-Garaya, J. P., Munayco-Apolaya, E., and Ugarte,
W. (2021). Carsharing system for urban transport in
lima using internet of things. In WEBIST.
Zhang, N. L. and Poole, D. L. (1994). A simple approach
to bayesian network computations. In Canadian AI.
Zou, X. and Yue, W. (2017). A bayesian network approach
to causation analysis of road accidents using netica.
Journal of Advanced Transportation, 2017.
A Bayesian Network for the Analysis of Traffic Accidents in Peru
315