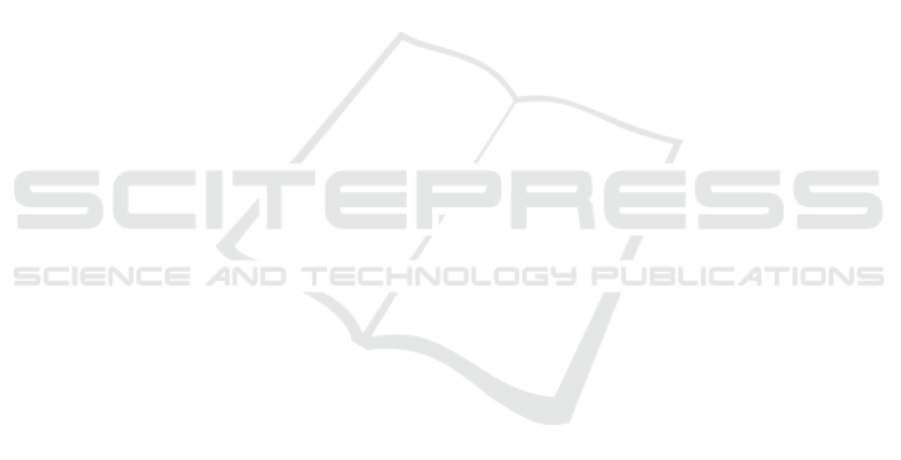
that the p-value generally decreases or stays the same
when word difficulty is explicitly added to the word
vectors. A smaller p-value indicates that the differ-
ences between scores at the adjacent levels are more
significant. The only cases where there is an increase
in the p-value was in the Superlative-Native score dif-
ferences. We may get this result because the number
of participants is not enough to appreciate a differ-
ence. Overall, we showed that introducing semantic
diversity when assessing vocabulary knowledge pos-
itively impacts the results and that word difficulty is
also indispensable.
5 CONCLUSION
This paper presented Vocabulary Volume, a new met-
ric to assess vocabulary knowledge. While the ex-
isting metrics consider only word difficulty, Vocabu-
lary Volume considers the semantic diversity as well
as word difficulty. We formalised the semantic diver-
sity by the volume of a convex hull that covers all
words represented by vectors in the semantic space.
Using data from a test assessing Japanese free produc-
tive vocabulary, we verified that the proposed metric
is valid to assess vocabulary knowledge by showing
it can distinguish learners’ responses with different
proficiency levels. We also confirmed that introduc-
ing semantic diversity into the word vector represen-
tations is effective. After exploring various configu-
rations for calculating the proposed metric, we con-
clude that as far as the data we used, the configuration
that adopts the BERT embeddings, PCA reducing to
dimension size four and frequency ranks as word dif-
ficulty achieves the best results.
In future work, we will evaluate the metric using
data from language learners of other languages than
Japanese and data from more diverse vocabulary as-
sessment tests.
ACKNOWLEDGEMENTS
This work was partially supported by JSPS KAK-
ENHI Grant Number JP19H04167 and JP21K18358.
REFERENCES
Alcoy, J. C. O. (2013). The schnabel method: An ecologi-
cal approach to productive vocabulary size estimation.
International Proceedings of Economics Development
and Research, 68:19–24.
Beglar, D. and Nation, P. (2007). A vocabulary size test.
The Language Teacher, 31(7):9–13.
Devlin, J., Chang, M.-W., Lee, K., and Toutanova, K.
(2019). BERT: Pre-training of deep bidirectional
transformers for language understanding. In Proceed-
ings of the 2019 Conference of the North American
Chapter of the Association for Computational Lin-
guistics: Human Language Technologies, Volume 1
(Long and Short Papers), pages 4171–4186.
Dong, T., Shi, W.-X., and Huang, Y.-H. (2010). A research
on evaluation of written productive vocabulary based
on sugeno measure. In Proceedings of 2010 Interna-
tional Conference on Machine Learning and Cyber-
netics, volume 1, pages 533–536. IEEE.
Fitzpatrick, T. and Clenton, J. (2017). Making sense of
learner performance on tests of productive vocabulary
knowledge. Tesol Quarterly, 51(4):844–867.
Gonz
´
alez, R. A. and P
´
ıriz, A. M. P. (2016). Measuring
the productive vocabulary of secondary school clil stu-
dents: Is Lex30 a valid test for low-level school learn-
ers? Vial-vigo International Journal of Applied Lin-
guistics, pages 31–54.
Graesser, A. C., McNamara, D. S., Louwerse, M. M., and
Cai, Z. (2004). Coh-metrix: Analysis of text on cohe-
sion and language. Behavior Research Methods, In-
struments, & Computers, 36(2):193–202.
Grave, E., Bojanowski, P., Gupta, P., Joulin, A., and
Mikolov, T. (2018). Learning word vectors for
157 languages. In Proceedings of the International
Conference on Language Resources and Evaluation
(LREC 2018), pages 3483–3487.
Henriksen, B. (1999). Three dimensions of vocabulary de-
velopment. Studies in Second Language Acquisition,
21(2):303–317.
Koizumi, R. (2003). A productive vocabulary knowledge
test for novice Japanese learners of English: Validity
and its scoring methods. JABAET Journal, 7:23–52.
Laufer, B. and Nation, P. (1995). Vocabulary size and use:
Lexical richness in L2 written production. Applied
Linguistics, 16(3):307–322.
Laufer, B. and Nation, P. (1999). A vocabulary-size test
of controlled productive ability. Language Testing,
16(1):33–51.
Laufer, B. and Paribakht, T. S. (1998). The relation-
ship between passive and active vocabularies: Ef-
fects of languagelearning context. Language Learn-
ing, 48(3):365–391.
Lin, T.-Y., Maire, M., Belongie, S., Hays, J., Perona, P., Ra-
manan, D., Doll
´
ar, P., and Zitnick, C. L. (2014). Mi-
crosoft COCO: Common objects in context. In Euro-
pean conference on computer vision, pages 740–755.
Springer.
Maekawa, K., Yamazaki, M., Ogiso, T., Maruyama, T.,
Ogura, H., Kashino, W., Koiso, H., Yamaguchi, M.,
Tanaka, M., and Den, Y. (2014). Balanced corpus of
contemporary written Japanese. Language Resources
and Evaluation, 48(2):345–371.
Manabe, H., Oka, T., Umikawa, Y., Takaoka, K., Uchida,
Y., and Asahara, M. (2019). Japanese word distributed
CSEDU 2022 - 14th International Conference on Computer Supported Education
64