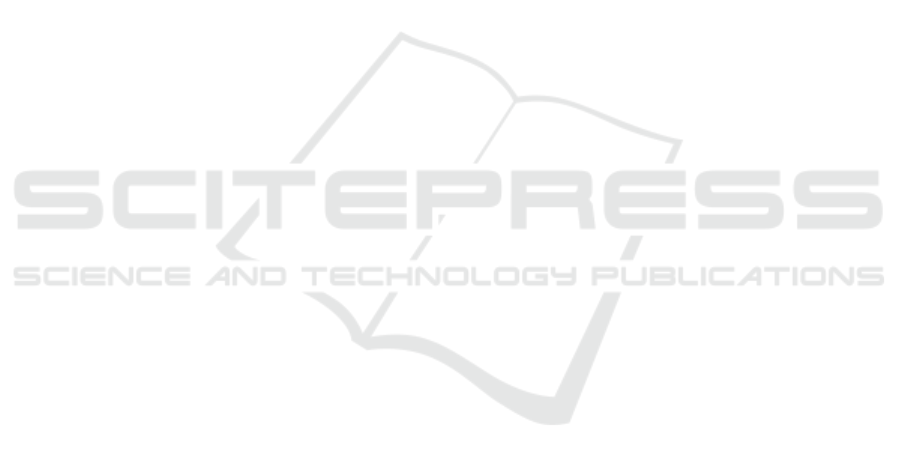
robust IQA method on all the distortion types and
databases. It is also possible to integrate the sparse
significance maps with the modern deep models. Fi-
nally, we would like to remark that SSIQM shows a
well moderate correlation with the HVS and holding
the promise to evaluate the images in a robust and ef-
fective manner when it is used in video codecs and
applications that consider image quality.
REFERENCES
A. Hyv
¨
arinen, J. K. and E. Oja, J. W. (2010). Independent
component analysis. New York, NY, 62(3):412–416.
Ahar, A., Barri, A., and Schelkens, P. (2018). From sparse
coding significance to perceptual quality: A new ap-
proach for image quality assessment. IEEE Transac-
tions on Image Processing, 27(2):879–893.
Bae, S.-H. and Kim, M. (2014). A novel generalized dct-
based jnd profile based on an elaborate cm-jnd model
for variable block-sized transforms in monochrome
images. IEEE Transactions on Image Processing,
23(8):3227–3240.
Bae, S.-H. and Kim, M. (2016). A novel image quality as-
sessment with globally and locally consilient visual
quality perception. IEEE Transactions on Image Pro-
cessing, 25(5):2392–2406.
Bell, A. J. and Sejnowski, T. J. (1995). An information-
maximization approach to blind separation and blind
deconvolution. Neural computation, 7(6):1129–1159.
Chandler, D. M. and Hemami, S. S. (2007). Vsnr: A
wavelet-based visual signal-to-noise ratio for natural
images. IEEE Transactions on Image Processing,
16(9):2284–2298.
Chang, H.-W., Yang, H., Gan, Y., and Wang, M.-H. (2013).
Sparse feature fidelity for perceptual image quality as-
sessment. IEEE Transactions on Image Processing,
22(10):4007–4018.
Comon, P. (1994). Independent component analysis, a new
concept? Signal processing, 36(3):287–314.
Daly, S. J. (1992). Visible differences predictor: an algo-
rithm for the assessment of image fidelity. In Hu-
man Vision, Visual Processing, and Digital Display
III, volume 1666, pages 2–15. International Society
for Optics and Photonics.
Damera-Venkata, N., Kite, T. D., Geisler, W. S., Evans,
B. L., and Bovik, A. C. (2000). Image quality as-
sessment based on a degradation model. IEEE trans-
actions on image processing, 9(4):636–650.
Farah, M. J. (2004). Visual agnosia. MIT press.
Guha, T., Nezhadarya, E., and Ward, R. K. (2014). Sparse
representation-based image quality assessment. Sig-
nal Processing: Image Communication, 29(10):1138–
1148.
Khellah, F. M. (2011). Texture classification using domi-
nant neighborhood structure. IEEE Transactions on
Image Processing, 20(11):3270–3279.
Kim, J. and Lee, S. (2016). Fully deep blind image quality
predictor. IEEE Journal of selected topics in signal
processing, 11(1):206–220.
Kim, J., Nguyen, A.-D., and Lee, S. (2018). Deep cnn-
based blind image quality predictor. IEEE trans-
actions on neural networks and learning systems,
30(1):11–24.
Larson, E. C. and Chandler, D. M. (2010). Most appar-
ent distortion: full-reference image quality assessment
and the role of strategy. Journal of electronic imaging,
19(1):011006.
Lee, T.-W., Girolami, M., and Sejnowski, T. J. (1999). Inde-
pendent component analysis using an extended info-
max algorithm for mixed subgaussian and supergaus-
sian sources. Neural computation, 11(2):417–441.
Li, L., Cai, H., Zhang, Y., Lin, W., Kot, A. C., and Sun,
X. (2016). Sparse representation-based image quality
index with adaptive sub-dictionaries. IEEE Transac-
tions on Image Processing, 25(8):3775–3786.
Liang, D., Gao, X., Lu, W., and Li, J. (2021). Deep blind
image quality assessment based on multiple instance
regression. Neurocomputing, 431:78–89.
Lin, K.-Y. and Wang, G. (2018). Hallucinated-iqa: No-
reference image quality assessment via adversarial
learning. In 2018 IEEE/CVF Conference on Computer
Vision and Pattern Recognition, pages 732–741.
Liu, A., Lin, W., and Narwaria, M. (2011). Image quality
assessment based on gradient similarity. IEEE Trans-
actions on Image Processing, 21(4):1500–1512.
Liu, Y., Zhai, G., Gu, K., Liu, X., Zhao, D., and Gao,
W. (2017). Reduced-reference image quality assess-
ment in free-energy principle and sparse representa-
tion. IEEE Transactions on Multimedia, 20(2):379–
391.
Ma, J., Wu, J., Li, L., Dong, W., Xie, X., Shi, G., and Lin,
W. (2021). Blind image quality assessment with active
inference. IEEE Transactions on Image Processing,
30:3650–3663.
Olshausen, B. A. and Field, D. J. (1996). Emergence
of simple-cell receptive field properties by learn-
ing a sparse code for natural images. Nature,
381(6583):607–609.
Olshausen, B. A. and Field, D. J. (1997). Sparse coding
with an overcomplete basis set: A strategy employed
by v1? Vision research, 37(23):3311–3325.
Pan, D., Shi, P., Hou, M., Ying, Z., Fu, S., and Zhang, Y.
(2018). Blind predicting similar quality map for image
quality assessment. In Proceedings of the IEEE con-
ference on computer vision and pattern recognition,
pages 6373–6382.
Pedregosa, F., Varoquaux, G., Gramfort, A., Michel, V.,
Thirion, B., Grisel, O., Blondel, M., Prettenhofer,
P., Weiss, R., Dubourg, V., Vanderplas, J., Passos,
A., Cournapeau, D., Brucher, M., Perrot, M., and
Duchesnay, E. (2011). Scikit-learn: Machine learning
in Python. Journal of Machine Learning Research,
12:2825–2830.
Ponomarenko, N., Jin, L., Ieremeiev, O., Lukin, V., Egiazar-
ian, K., Astola, J., Vozel, B., Chehdi, K., Carli, M.,
Battisti, F., and Jay Kuo, C.-C. (2015). Image database
An Image Quality Assessment Method based on Sparse Neighbor Significance
43