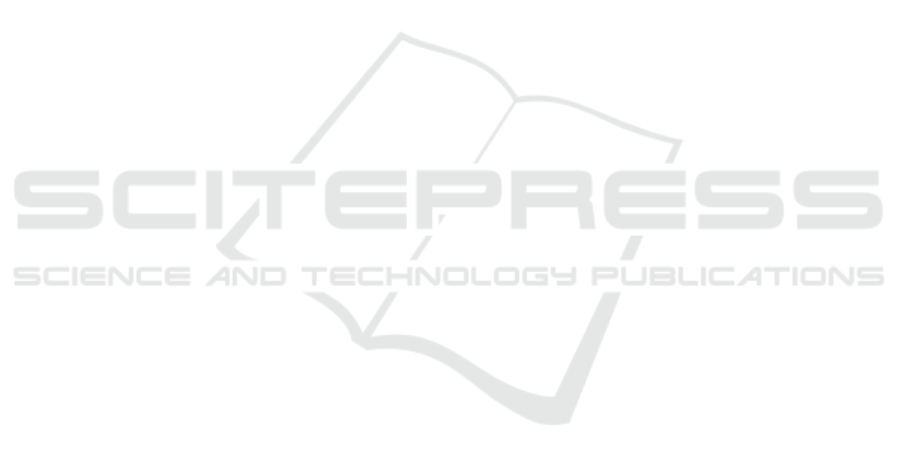
3d: A real and synthetic outdoor point cloud dataset
for challenging tasks in 3d mapping. Remote Sensing,
13(22).
Dosovitskiy, A., Ros, G., Codevilla, F., Lopez, A., and
Koltun, V. (2017). CARLA: An open urban driving
simulator. In Proceedings of the 1st Annual Confer-
ence on Robot Learning, pages 1–16.
Dworak, D., Ciepiela, F., Derbisz, J., Izzat, I., Ko-
morkiewicz, M., and W
´
ojcik, M. (2019). Perfor-
mance of lidar object detection deep learning architec-
tures based on artificially generated point cloud data
from carla simulator. In 2019 24th International Con-
ference on Methods and Models in Automation and
Robotics (MMAR), pages 600–605. IEEE.
Fang, J., Zhou, D., Yan, F., Zhao, T., Zhang, F., Ma, Y.,
Wang, L., and Yang, R. (2020). Augmented lidar sim-
ulator for autonomous driving. IEEE Robotics and
Automation Letters, 5(2):1931–1938.
Fong, W. K., Mohan, R., Hurtado, J. V., Zhou, L., Cae-
sar, H., Beijbom, O., and Valada, A. (2021). Panop-
tic nuscenes: A large-scale benchmark for lidar
panoptic segmentation and tracking. arXiv preprint
arXiv:2109.03805.
Geiger, A., Lenz, P., Stiller, C., and Urtasun, R. (2013).
Vision meets robotics: The kitti dataset. The Inter-
national Journal of Robotics Research, 32(11):1231–
1237.
Hahner, M., Sakaridis, C., Dai, D., and Van Gool, L. (2021).
Fog simulation on real lidar point clouds for 3d object
detection in adverse weather. In Proceedings of the
IEEE/CVF International Conference on Computer Vi-
sion, pages 15283–15292.
Huang, J. and Qiao, C. (2021). Generation for adaption:
A gan-based approach for 3d domain adaption with
point cloud data. ArXiv, abs/2102.07373.
Johnson-Roberson, M., Barto, C., Mehta, R., Sridhar, S. N.,
Rosaen, K., and Vasudevan, R. (2017). Driving in the
matrix: Can virtual worlds replace human-generated
annotations for real world tasks? In 2017 IEEE In-
ternational Conference on Robotics and Automation
(ICRA), pages 746–753. IEEE.
Kesten, R., Usman, M., Houston, J., Pandya, T., Nad-
hamuni, K., Ferreira, A., Yuan, M., Low, B., Jain, A.,
Ondruska, P., Omari, S., Shah, S., Kulkarni, A., Kaza-
kova, A., Tao, C., Platinsky, L., Jiang, W., and Shet,
V. (2019). Level 5. Perception Dataset 2020.
Langer, F., Milioto, A., Haag, A., Behley, J., and Stach-
niss, C. (2020). Domain transfer for semantic seg-
mentation of lidar data using deep neural networks.
In 2020 IEEE/RSJ International Conference on Intel-
ligent Robots and Systems (IROS), pages 8263–8270.
IEEE.
Manivasagam, S., Wang, S., Wong, K., Zeng, W.,
Sazanovich, M., Tan, S., Yang, B., Ma, W.-C., and
Urtasun, R. (2020). Lidarsim: Realistic lidar simu-
lation by leveraging the real world. In Proceedings
of the IEEE/CVF Conference on Computer Vision and
Pattern Recognition, pages 11167–11176.
Meng, Q., Wang, W., Zhou, T., Shen, J., Gool, L. V., and
Dai, D. (2020). Weakly supervised 3d object detection
from lidar point cloud. In European Conference on
Computer Vision, pages 515–531. Springer.
Sallab, A. E., Sobh, I., Zahran, M., and Essam, N.
(2019). Lidar sensor modeling and data augmenta-
tion with gans for autonomous driving. arXiv preprint
arXiv:1905.07290.
Saltori, C., Lathuili
´
ere, S., Sebe, N., Ricci, E., and Galasso,
F. (2020). Sf-uda 3d: Source-free unsupervised do-
main adaptation for lidar-based 3d object detection.
In 2020 International Conference on 3D Vision (3DV),
pages 771–780. IEEE.
Sun, B., Feng, J., and Saenko, K. (2017). Correlation align-
ment for unsupervised domain adaptation. In Domain
Adaptation in Computer Vision Applications, pages
153–171. Springer.
Sun, P., Kretzschmar, H., Dotiwalla, X., Chouard, A., Pat-
naik, V., Tsui, P., Guo, J., Zhou, Y., Chai, Y., Caine,
B., et al. (2020). Scalability in perception for au-
tonomous driving: Waymo open dataset. In Proceed-
ings of the IEEE/CVF Conference on Computer Vision
and Pattern Recognition, pages 2446–2454.
Tomasello, P., Sidhu, S., Shen, A., Moskewicz, M. W., Red-
mon, N., Joshi, G., Phadte, R., Jain, P., and Iandola,
F. (2019). Dscnet: Replicating lidar point clouds with
deep sensor cloning. In Proceedings of the IEEE/CVF
Conference on Computer Vision and Pattern Recogni-
tion Workshops, pages 0–0.
Triess, L. T., Dreissig, M., Rist, C. B., and Z
¨
ollner, J. M.
(2021). A survey on deep domain adaptation for lidar
perception. In Proc. IEEE Intelligent Vehicles Sympo-
sium (IV) Workshops.
Vacek, P., Ja
ˇ
sek, O., Zimmermann, K., and Svoboda, T.
(2021). Learning to predict lidar intensities. IEEE
Transactions on Intelligent Transportation Systems.
Wu, B., Zhou, X., Zhao, S., Yue, X., and Keutzer, K.
(2019). Squeezesegv2: Improved model structure
and unsupervised domain adaptation for road-object
segmentation from a lidar point cloud. In 2019 In-
ternational Conference on Robotics and Automation
(ICRA), pages 4376–4382. IEEE.
Yang, Z., Sun, Y., Liu, S., and Jia, J. (2020). 3dssd: Point-
based 3d single stage object detector. In Proceedings
of the IEEE/CVF conference on computer vision and
pattern recognition, pages 11040–11048.
Yue, X., Wu, B., Seshia, S. A., Keutzer, K., and
Sangiovanni-Vincentelli, A. L. (2018). A lidar point
cloud generator: from a virtual world to autonomous
driving. In Proceedings of the 2018 ACM on Inter-
national Conference on Multimedia Retrieval, pages
458–464.
Zhao, S., Wang, Y., Li, B., Wu, B., Gao, Y., Xu, P., Dar-
rell, T., and Keutzer, K. (2020). epointda: An end-to-
end simulation-to-real domain adaptation framework
for lidar point cloud segmentation. arXiv preprint
arXiv:2009.03456, 2.
Simulation-to-Reality Domain Adaptation for Offline 3D Object Annotation on Pointclouds with Correlation Alignment
149