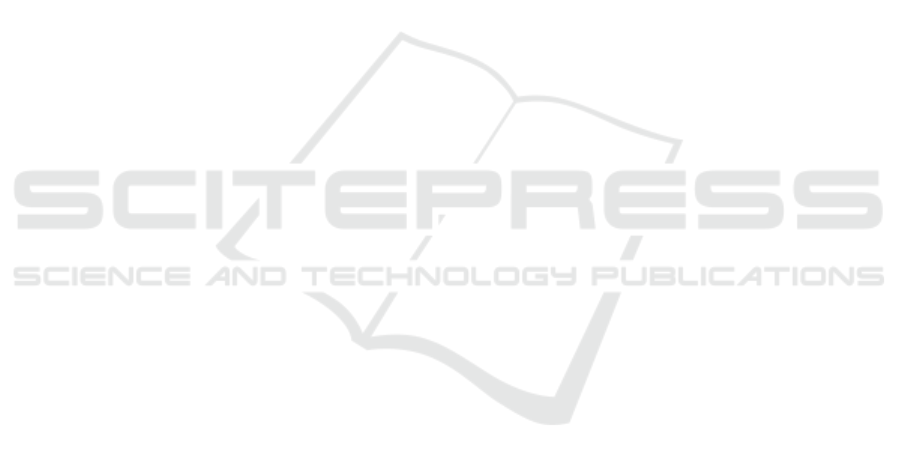
REFERENCES
Azam, F., Rizvi, A., Khan, W. Z., Aalsalem, M. Y., Yu, H.,
and Zikria, Y. B. (2021). Aircraft classification based
on pca and feature fusion techniques in convolutional
neural network. IEEE Access, 9:161683–161694.
Boughorbel, S., Tarel, J. P., and Boujemaa, N. (2005). The
intermediate matching kernel for image local features.
In Proceedings. 2005 IEEE International Joint Con-
ference on Neural Networks, 2005., volume 2, pages
889–894. IEEE.
Boumeddane, S., Hamdad, L., Dabo-Niang, S., and Had-
dadou, H. (2019). Spatial kernel discriminant analy-
sis: Applied for hyperspectral image classification. In
ICAART (2), pages 184–191.
Chaib, S., Liu, H., Gu, Y., and Yao, H. (2017). Deep fea-
ture fusion for vhr remote sensing scene classification.
IEEE Transactions on Geoscience and Remote Sens-
ing, 55(8):4775–4784.
Chang, C.-C. and Lin, C.-J. (2011). Libsvm: a library for
support vector machines. ACM transactions on intel-
ligent systems and technology (TIST), 2(3):1–27.
Chen, J., Zhang, B., and Wang, C. (2014). Backscatter-
ing feature analysis and recognition of civilian aircraft
in terrasar-x images. IEEE Geoscience and Remote
Sensing Letters, 12(4):796–800.
Cheng, G., Han, J., Guo, L., and Liu, T. (2015a). Learning
coarse-to-fine sparselets for efficient object detection
and scene classification. In Proceedings of the IEEE
conference on computer vision and pattern recogni-
tion, pages 1173–1181.
Cheng, G., Han, J., and Lu, X. (2017). Remote sensing
image scene classification: Benchmark and state of
the art. Proceedings of the IEEE, 105(10):1865–1883.
Cheng, G., Yang, C., Yao, X., Guo, L., and Han, J. (2018).
When deep learning meets metric learning: Remote
sensing image scene classification via learning dis-
criminative cnns. IEEE transactions on geoscience
and remote sensing, 56(5):2811–2821.
Cheng, G., Zhou, P., Han, J., Guo, L., and Han, J. (2015b).
Auto-encoder-based shared mid-level visual dictio-
nary learning for scene classification using very high
resolution remote sensing images. IET Computer Vi-
sion, 9(5):639–647.
Dalal, N. and Triggs, B. (2005). Histograms of oriented
gradients for human detection. In 2005 IEEE com-
puter society conference on computer vision and pat-
tern recognition (CVPR’05), volume 1, pages 886–
893. Ieee.
Dileep, A. D. and Sekhar, C. C. (2013). Gmm-based inter-
mediate matching kernel for classification of varying
length patterns of long duration speech using support
vector machines. IEEE Transactions on Neural Net-
works and Learning Systems, 25(8):1421–1432.
Ha, S.-W. and Moon, Y.-H. (2011). Multiple object track-
ing using sift features and location matching. Interna-
tional Journal of Smart Home, 5(4):17–26.
He, K., Zhang, X., Ren, S., and Sun, J. (2016). Iden-
tity mappings in deep residual networks. In Euro-
pean conference on computer vision, pages 630–645.
Springer.
He, N., Fang, L., Li, S., Plaza, A., and Plaza, J. (2018).
Remote sensing scene classification using multilayer
stacked covariance pooling. IEEE Transactions on
Geoscience and Remote Sensing, 56(12):6899–6910.
Huang, G., Liu, Z., Van Der Maaten, L., and Weinberger,
K. Q. (2017). Densely connected convolutional net-
works. In Proceedings of the IEEE conference on
computer vision and pattern recognition, pages 4700–
4708.
Krizhevsky, A., Sutskever, I., and Hinton, G. E. (2012). Im-
agenet classification with deep convolutional neural
networks. Advances in neural information processing
systems, 25:1097–1105.
Lee, K.-A., You, C., Li, H., and Kinnunen, T. (2007). A
gmm-based probabilistic sequence kernel for speaker
verification. In Eighth Annual Conference of the In-
ternational Speech Communication Association. Cite-
seer.
Li, F., Feng, R., Han, W., and Wang, L. (2020). High-
resolution remote sensing image scene classification
via key filter bank based on convolutional neural net-
work. IEEE Transactions on Geoscience and Remote
Sensing, 58(11):8077–8092.
Li, P., Ren, P., Zhang, X., Wang, Q., Zhu, X., and Wang, L.
(2018). Region-wise deep feature representation for
remote sensing images. Remote Sensing, 10(6):871.
Lin, J., Li, X., and Pan, H. (2018). Aircraft recognition
in remote sensing images based on deep learning. In
2018 33rd Youth Academic Annual Conference of Chi-
nese Association of Automation (YAC), pages 895–
899. IEEE.
Liu, G., Sun, X., Fu, K., and Wang, H. (2012). Aircraft
recognition in high-resolution satellite images using
coarse-to-fine shape prior. IEEE Geoscience and Re-
mote Sensing Letters, 10(3):573–577.
Lowe, D. G. (2004). Distinctive image features from scale-
invariant keypoints. International journal of computer
vision, 60(2):91–110.
Perveen, N., Roy, D., and Chalavadi, K. M. (2020). Fa-
cial expression recognition in videos using dynamic
kernels. IEEE Transactions on Image Processing,
29:8316–8325.
Simonyan, K. and Zisserman, A. (2014). Very deep con-
volutional networks for large-scale image recognition.
arXiv preprint arXiv:1409.1556.
Sitaula, C., Xiang, Y., Basnet, A., Aryal, S., and Lu, X.
(2020). Hdf: hybrid deep features for scene image
representation. In 2020 International Joint Confer-
ence on Neural Networks (IJCNN), pages 1–8. IEEE.
Smola, A. J. and Sch
¨
olkopf, B. (2004). A tutorial on
support vector regression. Statistics and computing,
14(3):199–222.
Szegedy, C., Liu, W., Jia, Y., Sermanet, P., Reed, S.,
Anguelov, D., Erhan, D., Vanhoucke, V., and Rabi-
novich, A. (2015). Going deeper with convolutions.
In Proceedings of the IEEE conference on computer
vision and pattern recognition, pages 1–9.
IMPROVE 2022 - 2nd International Conference on Image Processing and Vision Engineering
172