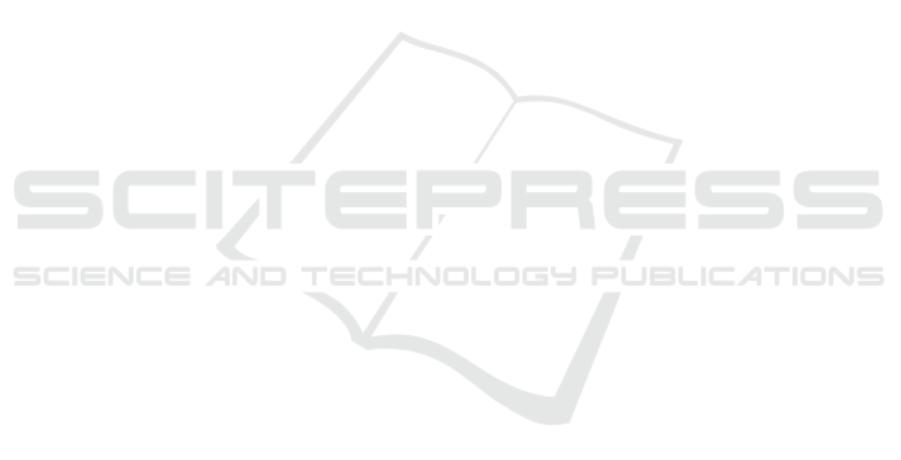
an understanding of these properties, especially those
that are technically important to measure losses - such
as the behavior of moisture content - can increase the
potential for optimizing the use of wood, regardless
of whether the destination is lamination, the chip pro-
duction or even power generation. Increasing the po-
tential for using the same wood will inevitably help
mitigate the acceleration of climate change globally.
ACKNOWLEDGEMENTS
This study was supported by CNPq (305805/2021-5)
and PNPD/CAPES (464880/2019-00).
REFERENCES
ˆ
Angelo, H., Almeida, A. N. d., and Serrano, A. L. M.
(2009). Determinantes da demanda de madeira em
toras para celulose no brasil. Scientia Forestalis,
37(84):491–498.
Bardak, S. and Bardak, T. (2019). Moisture content predic-
tion of wood drying process using fuzzy logic. Acta
Scientiarum Polonorum Silvarum Colendarum Ratio
et Industria Lignaria, 18(1):71–75.
Brazilian Society of Agriculture (2019). Export of
eucalyptus logs doubles with chinese demand (in
portuguese). https://www.sna.agr.br/exportacao-de-
tora-de-eucalipto-dobra-com-demanda-chinesa/. Ac-
cessed: dec., 2021.
Dorigato, A., Fredi, G., and Pegoretti, A. (2019).
Thermo-mechanical behavior of novel wood laminae-
thermoplastic starch biodegradable composites with
thermal energy storage/release capability. Frontiers
in Materials, 6. Article 76.
El Naqa, I. and Murphy, M. J. (2015). What Is Machine
Learning?, pages 3–11. Springer Intl. Pub., Cham.
Erber, G., Kanzian, C., Stampfer, K., et al. (2012). Predict-
ing moisture content in a pine logwood pile for energy
purposes. Silva Fennica, 46(4):555–567.
Estuqui Filho, C. A. (2006). A durabilidade da madeira
na arquitetura sob a ac¸
˜
ao dos fatores naturais: estudo
de casos em bras
´
ılia. Master’s thesis, Faculdade de
Arquitetura e Urbanismo - Universidade de Bras
´
ılia.
Gerwing, J., Vidal, E., Ver
´
ıssimo, A., and Uhl, C. (2000).
Rendimento no processamento de madeira no estado
do Par
´
a. S
´
erie Amaz
ˆ
onia, 18:38.
Gigler, J. K., van Loon, W. K., Van den Berg, J., Sonneveld,
C., and Meerdink, G. (2000). Natural wind drying of
willow stems. Biomass & Bioenergy, 19(3):153–163.
Hu, Z. F. and Khan, M. S. (1997). Why is china growing so
fast? Staff Papers, 44(1):103–131.
J
´
unior, A. S. and Alves, J. E. (2019). Fatores intervenientes
no armazenamento de laminados. Gepros: Gest
˜
ao da
Produc¸
˜
ao, Operac¸
˜
oes e Sistemas, 14(5):190.
Kofman, P. D. and Kent, T. (2009). Long term storage and
seasoning of conifer energy wood. Coford connects–
Harvesting/Transportaions, 20:1–4.
Kr
¨
oll, K. (1959). Selbstt
¨
atiges steuern und regeln der trock-
ner. In Trockner und Trocknungsverfahren, pages
548–563. Springer.
Lima, R., da Silva Cardoso, G., de Proenc¸a, G., and
da Costa, W. G. (2017). Influ
ˆ
encia do tempo de ar-
mazenamento (tpc) da madeira no aceite de cavacos
de eucalipto para a fabricac¸
˜
ao de polpa. In Congresso
Internacional de Celulose e Papel. ABTCP.
Mitchell, P. H. (2019). Development of an air drying index
for lumber. BioResources, 14(3):6657–6665.
Mitchell, T. (1997). Machine learning. MacGraw-Hill.
Morin, M., Gaudreault, J., Brotherton, E., Paradis, F., Rol-
land, A., Wery, J., and Laviolette, F. (2020). Machine
learning-based models of sawmills for better wood al-
location planning. Int. Journal of Prod. Econ., 222.
Raitila, J., Heiskanen, V.-P., Routa, J., Kolstr
¨
om, M., and
Sikanen, L. (2015). Comparison of moisture predic-
tion models for stacked fuelwood. BioEnergy Re-
search, 8(4):1896–1905.
Rezende, R. N., Lima, J. T., de Ramos, L. E., Faria, A.
L. R., et al. (2010). Secagem ao ar livre de toras de
eucalyptus grandis em Lavras/MG. Cerne, 16:41–47.
Russell, S. and Norvig, P. (2002). Artificial intelligence: a
modern approach. Prentice Hall.
Simpson, W. T. (2000). Estimates of air drying times for
several hardwoods and softwoods, volume 121. US
Dept. of Agriculture, Forest Service, Forest Prod. Lab.
Simpson, W. T. (2004). Estimating air drying times of lum-
ber with multiple regression, volume 293. US Dept of
Agriculture, Forest Service, Forest Prod. Lab.
Souza, G. S. A. d. (2019). Aprendizado de m
´
aquina em
aplicac¸
˜
oes de manejo florestal. PhD thesis, UFV.
Th
¨
ornqvist, T. (1985). Drying and storage of forest residues
for energy production. Biomass, 7(2):125–134.
Tomczak, A., Grodzi
´
nski, G., Jakubowski, M., Jelonek, T.,
and Grzywi
´
nski, W. (2018). Effects of short-term stor-
age method on moisture loss and weight change in
beech timber. Croatian J. of Forest Eng, 39(1):35–43.
Tsoumis, G. et al. (1991). Science and technology of wood:
structure, properties, utilization, volume 115. Van
Nostrand Reinhold New York.
Vieira, P. A., Buainain, A. M., and Figueiredo, E. V. C.
(2019). O brasil alimentar
´
a a china ou a china engolir
´
a
o brasil? Tempo do Mundo, 2(1):51–82.
Visser, R., Berkett, H., and Spinelli, R. (2014). Determining
the effect of storage conditions on the natural drying
of radiata pine logs for energy use. New Zealand Jour-
nal of Forestry Science, 44(1):1–8.
Walker, J. C. (2006). Primary wood processing: principles
and practice. Springer Science & Business Media.
Wen, S., Deng, M., and Inoue, A. (2012). Moisture con-
tent prediction of wood drying process using svm-
based model. Int. J. Inov. Comput. Infomation Con-
trol, 8:4083–4093.
Wu, H. and Avramidis, S. (2006). Prediction of timber kiln
drying rates by neural networks. Drying Technology,
24(12):1541–1545.
Zhang, X.-D. (2020). Machine learning. In A Matrix Al-
gebra Approach to Artificial Intelligence, pages 223–
440. Springer.
ICEIS 2022 - 24th International Conference on Enterprise Information Systems
528