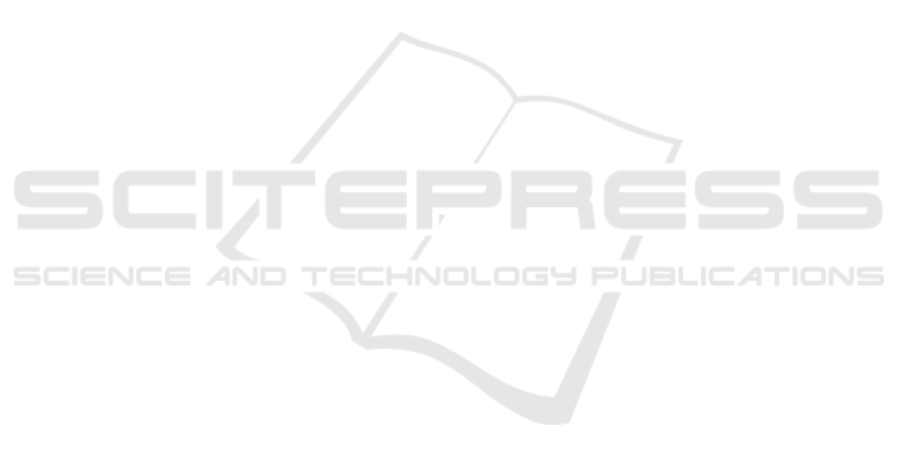
that LIME and image mining techniques can be used
to improve business processes. The results show that
our approach is able to identify relevant process de-
tails. The process of metal injection molding or ex-
trusion is taken as an example from real-world envi-
ronments. The presented concept is applicable for a
large set of similar processes that involve process par-
ticipants in pick-and-place tasks. However, its flexi-
bility allows to extend its scope of application to other
tasks.
Future work should focus on using more power-
ful image mining techniques that increase the robust-
ness and accuracy of the Image Analysis step. We
aim to extend our experiments with more complex
image data from real process environments. We fur-
ther plan to evaluate the proposed ways of integrating
process details in existing process models in a user
study. This includes the investigation of how a certain
feature must be represented in order to be interpreted
correctly and efficiently by process participants.
REFERENCES
Van der Aalst, W. M. P., Van Dongen, B. F., G
¨
unther, C. W.,
Rozinat, A., Verbeek, E., and Weijters, T. (2009).
Prom: The process mining toolkit, in bpm (demos).
In CEUR Workshop Proceedings, volume 489.
Becker, J., Rosemann, M., and von Uthmann, C. (2000).
Guidelines of Business Process Modeling, pages 30–
49. Springer Berlin Heidelberg, Berlin, Heidelberg.
Burkart, N. and Huber, M. F. (2021). A survey on the ex-
plainability of supervised machine learning. Journal
of Artificial Intelligence Research, 70:245–317.
Chinosi, M. and Trombetta, A. (2012). Bpmn: An intro-
duction to the standard. Computer Standards & Inter-
faces, 34(1):124–134.
Dzindolet, M. T., Peterson, S. A., Pomranky, R. A., Pierce,
L. G., G, L., and Beck, H. P. (2003). The role of
trust in automation reliance. International journal of
human-computer studies, 58(6):697–718.
Fichtner, M., Sch
¨
onig, S., and Jablonski, S. (2020). Pro-
cess management enhancement by using image min-
ing techniques: A position paper. In Proceedings of
the 22nd International Conference on Enterprise In-
formation Systems, volume 1 of ICEIS, pages 249–
255.
Fichtner, M., Sch
¨
onig, S., and Jablonski, S. (2021). Us-
ing image mining techniques from a business process
perspective. In Enterprise Information Systems, pages
62–83, Cham. Springer International Publishing.
Front, A., Rieu, D., Santorum, M., and Movahedian, F.
(2017). A participative end-user method for multi-
perspective business process elicitation and improve-
ment. Software & Systems Modeling, 16(3):691–714.
Gounaris, A. (2016). Towards automated performance op-
timization of bpmn business processes. In East Euro-
pean Conference on Advances in Databases and In-
formation Systems, pages 19–28. Springer.
Kluza, K., Baran, M., Bobek, S., and Nalepa, G. J. (2013).
Overview of recommendation techniques in business
process modeling. In Proceedings of 9th Workshop
on Knowledge Engineering and Software Engineering
(KESE9), pages 46–57. Citeseer.
Mendling, J., Reijers, H. A., and van der Aalst, W. M. P.
(2010). Seven process modeling guidelines (7pmg).
Information and Software Technology, 52(2):127–
136.
Niedermann, F., Radesch
¨
utz, S., and Mitschang, B. (2010).
Deep business optimization: A platform for auto-
mated process optimization. INFORMATIK 2010–
Business Process and Service Science–Proceedings of
ISSS and BPSC.
Polyvyanyy, A., Smirnov, S., and Weske, M. (2008). Pro-
cess model abstraction: A slider approach. In 2008
12th International IEEE Enterprise Distributed Ob-
ject Computing Conference, pages 325–331. IEEE.
Popescu, M. C. and Lucian, M. S. (2014). Feature extrac-
tion, feature selection and machine learning for im-
age classification: A case study. In 2014 International
Conference on Optimization of Electrical and Elec-
tronic Equipment (OPTIM), pages 968–973. IEEE.
Pryk
¨
ari, T., Czajkowsk, J., Alarousu, E., and Myllyl
¨
a, R.
(2010). Optical coherence tomography as an accurate
inspection and quality evaluation technique in paper
industry. Optical review, 17(3):218–222.
Radesch
¨
utz, S., Mitschang, B., and Leymann, F. (2008).
Matching of process data and operational data for a
deep business analysis. In Enterprise Interoperability
III, pages 171–182. Springer.
Reichert, M., Kolb, J., Bobrik, R., and Bauer, T. (2012).
Enabling personalized visualization of large business
processes through parameterizable views. In Proceed-
ings of the 27th Annual ACM Symposium on Applied
Computing, pages 1653–1660.
Ribeiro, M. T., Singh, S., and Guestrin, C. (2016). ” why
should i trust you?” explaining the predictions of any
classifier. In Proceedings of the 22nd ACM SIGKDD
international conference on knowledge discovery and
data mining, pages 1135–1144.
Schmidt, R., M
¨
ohring, M., Zimmermann, A., H
¨
arting, R.-
C., and Keller, B. (2016). Potentials of image mining
for business process management. In Intelligent Deci-
sion Technologies 2016, pages 429–440. Springer.
Schonenberg, H., Weber, B., van Dongen, B., and van der
Aalst, W. M. P. (2008). Supporting flexible processes
through recommendations based on history. In In-
ternational Conference on Business Process Manage-
ment, pages 51–66. Springer.
Wiedmann, P. C. K. (2017). Agiles
Gesch
¨
aftsprozessmanagement auf Basis ge-
brauchssprachlicher Modellierung. PhD thesis,
University of Bayreuth, Germany.
ICEIS 2022 - 24th International Conference on Enterprise Information Systems
534