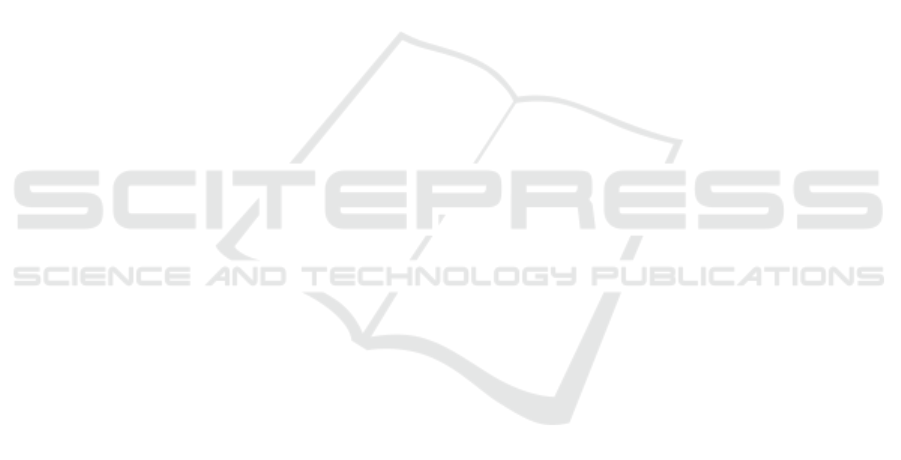
ACKNOWLEDGMENTS
This work was partially funded by Lenovo, as
part of its R&D investment under Brazil’s Infor-
matics Law, CAPES/Brazil (under grant numbers
88887.609129/2021 and 88881.189723/2018-01) and
LSBD/UFC.
REFERENCES
Ara
´
ujo, M., Pereira, A., and Benevenuto, F. (2020).
A comparative study of machine translation
for multilingual sentence-level sentiment anal-
ysis. Information Sciences, 512:1078–1102.
https://repositorio.ufmg.br/bitstream/1843/ESBF-
AQ2PSM/1/matheuslimadiniz.pdf.
Bonadiman, D., Castellucci, G., Favalli, A., Romagnoli, R.,
and Moschitti, A. (2017). Neural sentiment analysis
for a real-world application.
Ca
˜
nete, J., Chaperon, G., Fuentes, R., Ho, J.-H., Kang, H.,
and P
´
erez, J. (2020). Spanish pre-trained bert model
and evaluation data. In PML4DC at ICLR 2020.
Cai, Y., Huang, Q., Lin, Z., Xu, J., Chen, Z., and Li, Q.
(2020). Recurrent neural network with pooling oper-
ation and attention mechanism for sentiment analysis:
A multi-task learning approach. Knowledge-Based
Systems, 203:1–12.
Chen, S., Peng, C., Cai, L., and Guo, L. (2018). A deep neu-
ral network model for target-based sentiment analysis.
In 2018 International Joint Conference on Neural Net-
works (IJCNN), pages 1–7.
Devlin, J., Chang, M.-W., Lee, K., and Toutanova, K.
(2018). Bert: Pre-training of deep bidirectional trans-
formers for language understanding. arXiv preprint
arXiv:1810.04805.
Graff, M., Miranda-Jim
´
enez, S., Tellez, E. S., and
Moctezuma, D. (2018). Evomsa: A multilingual evo-
lutionary approach for sentiment analysis. CoRR,
abs/1812.02307.
Guo, S., H
¨
ohn, S., Xu, F., and Schommer, C. (2018).
Perseus: A personalization framework for sentiment
categorization with recurrent neural network. In
ICAART.
Jianqiang, Z., Xiaolin, G., and Xuejun, Z. (2018). Deep
convolution neural networks for twitter sentiment
analysis. IEEE Access, 6:23253–23260.
Letarte, G., Paradis, F., Gigu
`
ere, P., and Laviolette, F.
(2018). Importance of self-attention for sentiment
analysis. In Proceedings of the 2018 EMNLP Work-
shop BlackboxNLP: Analyzing and Interpreting Neu-
ral Networks for NLP, pages 267–275, Brussels, Bel-
gium. Association for Computational Linguistics.
Mart
´
ınez-C
´
amara, E., D
´
ıaz-Galiano, M., Garc
´
ıa-
Cumbreras, M., Garc
´
ıa-Vega, M., and Villena-Rom
´
an,
J. (2017). Overview of tass 2017.
Rosenthal, S., Farra, N., and Nakov, P. (2017). SemEval-
2017 task 4: Sentiment analysis in Twitter. In Pro-
ceedings of the 11th International Workshop on Se-
mantic Evaluation (SemEval-2017), pages 502–518,
Vancouver, Canada. Association for Computational
Linguistics.
Sarlis, S. and Maglogiannis, I. (2020). On the reusabil-
ity of sentiment analysis datasets in applications with
dissimilar contexts. In Maglogiannis, I., Iliadis, L.,
and Pimenidis, E., editors, Artificial Intelligence Ap-
plications and Innovations, pages 409–418, Cham.
Springer International Publishing.
Socher, R., Perelygin, A., Wu, J., Chuang, J., Manning,
C. D., Ng, A. Y., and Potts, C. (2013). Recursive
deep models for semantic compositionality over a sen-
timent treebank. In Proceedings of the 2013 confer-
ence on empirical methods in natural language pro-
cessing, pages 1631–1642.
Souza, F., Nogueira, R., and Lotufo, R. (2020). BERTim-
bau: pretrained BERT models for Brazilian Por-
tuguese. In 9th Brazilian Conference on Intelligent
Systems, BRACIS, Rio Grande do Sul, Brazil, October
20-23 (to appear).
Sun, Y., Wang, S., Li, Y., Feng, S., Tian, H., Wu, H.,
and Wang, H. (2019). Ernie 2.0: A continual pre-
training framework for language understanding. arXiv
preprint arXiv:1907.12412.
Wang, A., Singh, A., Michael, J., Hill, F., Levy, O., and
Bowman, S. R. (2019). GLUE: A multi-task bench-
mark and analysis platform for natural language un-
derstanding. In the Proceedings of ICLR.
Wei, J. W. and Zou, K. (2019). EDA: easy data augmenta-
tion techniques for boosting performance on text clas-
sification tasks. CoRR, abs/1901.11196.
Wu, X., Lv, S., Zang, L., Han, J., and Hu, S. (2018).
Conditional BERT contextual augmentation. CoRR,
abs/1812.06705.
ICEIS 2022 - 24th International Conference on Enterprise Information Systems
560