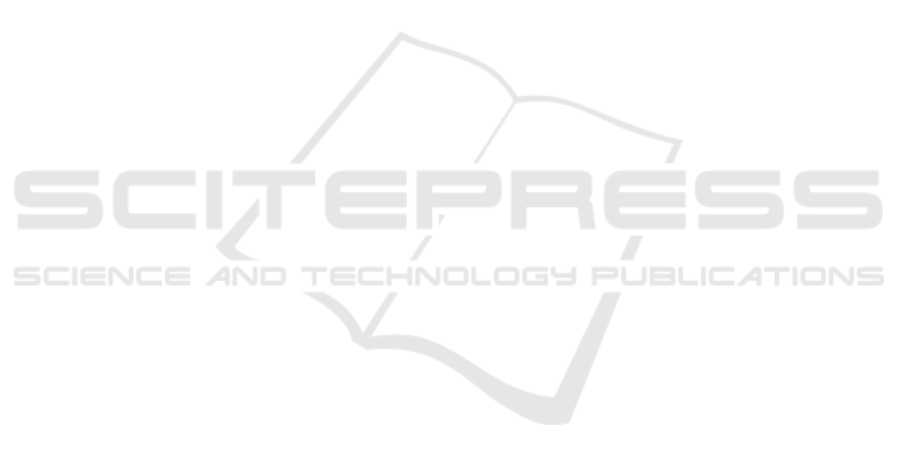
remote sensing data. In IGARSS 2019-2019 IEEE In-
ternational Geoscience and Remote Sensing Sympo-
sium, pages 6344–6347. IEEE.
Gorbova, J., Lusi, I., Litvin, A., and Anbarjafari, G. (2017).
Automated screening of job candidate based on mul-
timodal video processing. In Proceedings of the IEEE
conference on computer vision and pattern recogni-
tion workshops, pages 29–35.
Huang, G., Liu, Z., Van Der Maaten, L., and Weinberger,
K. Q. (2017). Densely connected convolutional net-
works. In Proceedings of the IEEE conference on
computer vision and pattern recognition, pages 4700–
4708.
Huang, G., Liu, Z., and Weinberger, K. Q. (2016).
Densely connected convolutional networks. CoRR,
abs/1608.06993.
Jackson, P. and Haq, S. (2014). Surrey audio-visual ex-
pressed emotion (savee) database. University of Sur-
rey: Guildford, UK.
Kingma, D. P. and Ba, J. (2014). Adam: A
method for stochastic optimization. arXiv preprint
arXiv:1412.6980.
Kong, Q., Cao, Y., Iqbal, T., Wang, Y., Wang, W., and
Plumbley, M. D. (2020). Panns: Large-scale pre-
trained audio neural networks for audio pattern recog-
nition. IEEE ACM Trans. Audio Speech Lang. Pro-
cess., 28:2880–2894.
Kopparapu, S. K. (2015). Non-linguistic analysis of call
center conversations. Springer.
LeCun, Y., Bottou, L., Bengio, Y., and Haffner, P. (1998).
Gradient-based learning applied to document recogni-
tion. Proceedings of the IEEE, 86(11):2278–2324.
Livingstone, S. R. and Russo, F. A. (2018). The ryerson
audio-visual database of emotional speech and song
(ravdess): A dynamic, multimodal set of facial and
vocal expressions in north american english. PloS one,
13(5):e0196391.
Lu, H., Zhang, H., and Nayak, A. (2020a). A deep neural
network for audio classification with a classifier atten-
tion mechanism. arXiv preprint arXiv:2006.09815.
Lu, L. and Hanjalic, A. (2009). Audio Classification, pages
148–154. Springer US, Boston, MA.
Lu, Q., Li, Y., Qin, Z., Liu, X., and Xie, Y. (2020b).
Speech recognition using efficientnet. In Proceedings
of the 2020 5th International Conference on Multime-
dia Systems and Signal Processing, pages 64–68.
Mushtaq, Z. and Su, S.-F. (2020). Environmental sound
classification using a regularized deep convolutional
neural network with data augmentation. Applied
Acoustics, 167:107389.
Mustaqeem, Sajjad, M., and Kwon, S. (2020). Clustering-
based speech emotion recognition by incorporating
learned features and deep bilstm. IEEE Access,
8:79861–79875.
Noroozi, F., Marjanovic, M., Njegus, A., Escalera, S., and
Anbarjafari, G. (2017). Audio-visual emotion recog-
nition in video clips. IEEE Transactions on Affective
Computing, 10(1):60–75.
Palanisamy, K., Singhania, D., and Yao, A. (2020). Re-
thinking CNN models for audio classification. CoRR,
abs/2007.11154.
Paszke, A., Gross, S., Chintala, S., Chanan, G., Yang, E.,
DeVito, Z., Lin, Z., Desmaison, A., Antiga, L., and
Lerer, A. (2017). Automatic differentiation in pytorch.
NIPS Workshop.
Piczak, K. J. (2015). ESC: dataset for environmental sound
classification. In Zhou, X., Smeaton, A. F., Tian, Q.,
Bulterman, D. C. A., Shen, H. T., Mayer-Patel, K., and
Yan, S., editors, Proceedings of the 23rd Annual ACM
Conference on Multimedia Conference, MM ’15, Bris-
bane, Australia, October 26 - 30, 2015, pages 1015–
1018. ACM.
Salamon, J., Jacoby, C., and Bello, J. P. (2014). A dataset
and taxonomy for urban sound research. In Hua,
K. A., Rui, Y., Steinmetz, R., Hanjalic, A., Natsev,
A., and Zhu, W., editors, Proceedings of the ACM In-
ternational Conference on Multimedia, MM ’14, Or-
lando, FL, USA, November 03 - 07, 2014, pages 1041–
1044. ACM.
Seo, M. and Kim, M. (2020). Fusing visual attention CNN
and bag of visual words for cross-corpus speech emo-
tion recognition. Sensors, 20(19):5559.
Thornton, B. (2019). Audio recognition using mel spectro-
grams and convolution neural networks.
Uc¸ar, M. K., Bozkurt, M. R., and Bilgin, C. (2017). Signal
processing and communications applications confer-
ence. IEEE.
Van Uden, C. E. (2019). Comparing brain-like represen-
tations learned by vanilla, residual, and recurrent cnn
architectures. Phd thesis, Dartmouth College.
Xu, Y., Kong, Q., Wang, W., and Plumbley, M. D. (2018).
Large-scale weakly supervised audio classification us-
ing gated convolutional neural network. In 2018 IEEE
international conference on acoustics, speech and sig-
nal processing (ICASSP), pages 121–125. IEEE.
AUDIO-MC: A General Framework for Multi-context Audio Classification
383