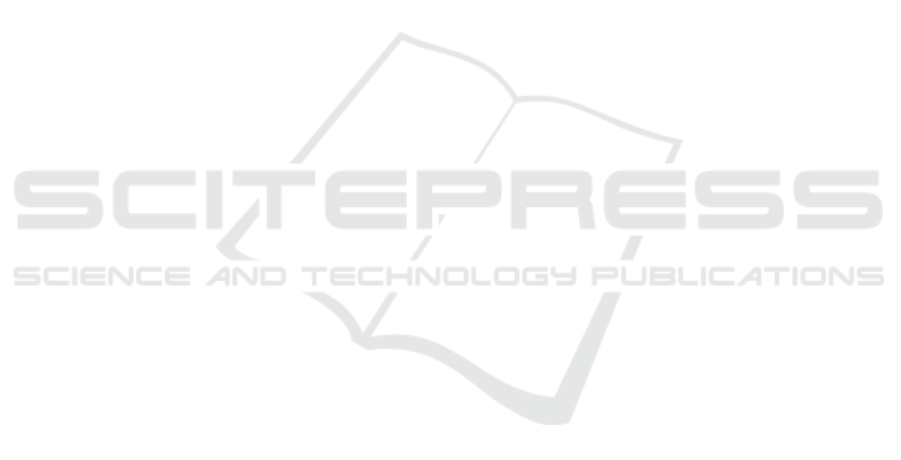
as compared to other sectors. We also showed that
companies in the finance sector have similarities in
their ER movements as to that of big-size companies,
even though they mostly had the lowest capitalization.
Based on the obtained results, it can be concluded that
investment in a small company with low capitaliza-
tion in the finance sector, even during the crises, may
yield a higher return than investment in large compa-
nies. From 2000 to 2004, companies in the finance
sector kept their consistency with low capitalization
and got the same ER as big companies with high cap-
italization (RQ1). Using the population analysis, we
did not find any parameters outside network charac-
teristics that significantly affected the behavior of the
companies under study (RQ2).
The proposed model and the reported results rep-
resent a starting point for a new direction in analyz-
ing financial markets. The results show the viabil-
ity of this new approach. However, additional studies
with larger and more diverse data sets are necessary
to make a case for utilizing the concept of population
analysis in making important financial decisions. The
limitation of this study is that we analyzed the market
for a limited sample during the 2000-2004 time pe-
riod. To further validate the obtained results, we plan
to conduct a more comprehensive study using the pro-
posed approach for different time periods and utiliz-
ing different types of data sets. We intend to apply
the concept of population analysis on different sets of
data tied to independently-established major crises in
order to recognize the patterns that may be otherwise
obfuscated. In addition to ER, future studies also in-
clude exploring other indicators such as different eco-
nomic sectors and companies’ sizes for comparing the
behavior of companies in financial markets.
REFERENCES
Batushansky, A., Toubiana, D., and Fait, A. (2016).
Correlation-based network generation, visualization,
and analysis as a powerful tool in biological studies:
a case study in cancer cell metabolism. BioMed re-
search international, 2016.
Bettman, J. L., Sault, S. J., and Schultz, E. L. (2009). Fun-
damental and technical analysis: substitutes or com-
plements? Accounting & Finance, 49(1):21–36.
Bonanno, G., Caldarelli, G., Lillo, F., Micciche, S., Vande-
walle, N., and Mantegna, R. N. (2004). Networks of
equities in financial markets. The European Physical
Journal B, 38(2):363–371.
Chetti, P. and Ali, H. (2019). Analyzing the structural health
of civil infrastructures using correlation networks and
population analysis. In Proceedings of the Eighth In-
ternational Conference on Data Analytics, pages 12–
19.
Chetti, P. and Ali, H. (2020). Estimating the inspection
frequencies of civil infrastructures using correlation
networks and population analysis. International Jour-
nal on Advances in Intelligent Systems, 13(1&2):125–
136.
Chi, K. T., Liu, J., and Lau, F. C. (2010). A network per-
spective of the stock market. Journal of Empirical
Finance, 17(4):659–667.
Cooper, K., Hassan, W., and Ali, H. (2019). Identification
of temporal network changes in short-course gene ex-
pression from c. elegans reveals structural volatility.
International Journal of Computational Biology and
Drug Design, 12(2):171–188.
Dempsey, K., Thapa, I., Bastola, D., and Ali, H. (2011).
Identifying modular function via edge annotation in
gene correlation networks using gene ontology search.
In 2011 IEEE International Conference on Bioinfor-
matics and Biomedicine Workshops (BIBMW), pages
255–261. IEEE.
Dempsey, K. M. and Ali, H. H. (2014). Identifying aging-
related genes in mouse hippocampus using gateway
nodes. BMC systems biology, 8(1):62.
Fuchsberger, A. and Ali, H. (2017). A correlation network
model for structural health monitoring and analyzing
safety issues in civil infrastructures. In Proceedings of
the 50th Hawaii International Conference on System
Sciences.
Gupta, A. and Dhingra, B. (2012). Stock market predic-
tion using hidden markov models. In 2012 Students
Conference on Engineering and Systems, pages 1–4.
IEEE.
Hatami, Z., Chetti, P., Ali, H., and Volkman, D. (2022).
A novel population analysis approach for analyzing
financial markets under crises – 2008 economic crash
and covid-19 pandemic.
Hatami, Z., Hall, M., and Thorne, N. (2021). Identifying
early opinion leaders on covid-19 on twitter. In In-
ternational Conference on Human-Computer Interac-
tion, pages 280–297. Springer.
Kenett, D. Y., Preis, T., Gur-Gershgoren, G., and Ben-
Jacob, E. (2012). Dependency network and node in-
fluence: Application to the study of financial mar-
kets. International Journal of Bifurcation and Chaos,
22(07):1250181.
Kim, S., Thapa, I., and Ali, H. H. (2018). A graph-theoretic
approach for identifying bacterial inter-correlations
and functional pathways in microbiome data. In 2018
IEEE International Conference on Bioinformatics and
Biomedicine (BIBM), pages 405–411. IEEE.
Lancellotta, P. I., Str
¨
oele, V., Braga, R. M., David, J. M. N.,
and Campos, F. (2018). Semantic analysis and com-
plex networks as conjugated techniques supporting
decision making. In ICEIS (2), pages 195–202.
Mi
´
skiewicz, J. (2012). Analysis of time series correlation.
the choice of distance metrics and network structure.
Acta Phys. Pol. A, 121:B–89.
Rastegari, E., Azizian, S., and Ali, H. (2019). Machine
learning and similarity network approaches to support
automatic classification of parkinson’s diseases using
accelerometer-based gait analysis. In Proceedings of
ICEIS 2022 - 24th International Conference on Enterprise Information Systems
576