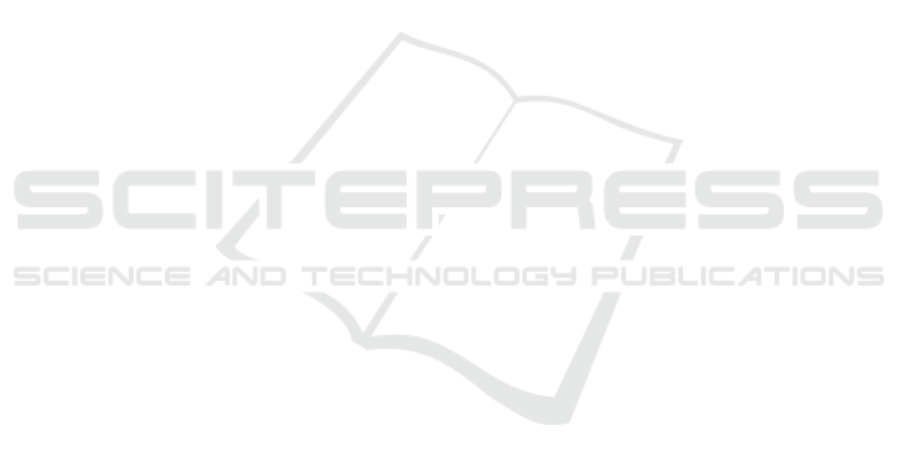
In order to outline the behavior of the prediction
approach over a longer distance, figure 10 illustrates
the SOC and fuel consumption prediction for the en-
tire test drive sequence of 6.5 km.
4 CONCLUSION
In this paper, an approach is presented to predict the
energy demand of a hybrid electrical vehicle, based
on route data and speed values. The approach is build
to be suitable for an application within optimization
algorithms, as it only relies on input data for a single
transition between two operation points. The predic-
tion procedure is composted by a series of individual
models that represent the behavior of the single power
train components and can be described as follows.
For given speed and elevation values in two con-
secutive route points, the required drive torque at the
wheels is calculated using a longitudinal vehicle dy-
namic model. Further, a gearbox model is build and
identified to estimate the transmission behavior, based
on the wheel torque and vehicle speed. In order to de-
termine which part of the required total drive torque
will be applied by the individual drives, a model is
build to estimate a torque distribution ratio, based on
the total drive torque, the vehicle speed and the pre-
vious state of charge. Having the separate torque val-
ues, the combustion engine’s required amount of fuel
can be derived from a characteristic diagram. For the
electric part of the power train, the current and the
voltage are estimated and used within a battery model
to determine the required electric energy.
A data set of 525 driven kilometers is created to
identify and validate the prediction approach on a
Volkswagen Golf VII GTE. Using drive sequences of
5 to 7km within a 6-fold cross validation procedure,
an averaged root mean square error of 0.91 % can be
determined for the prediction of the state of charge.
Regarding the prediction of the amount of fuel re-
quired by the combustion engine, this error value re-
sults to 0.05 liter. The main part of prediction inaccu-
racies can be attributed to the estimation of the single
torques, to be applied by the individual engines. In
dynamic driving situation, in which both engines are
required, the estimation approach is not always capa-
ble to predict the correct distribution of the required
total drive torque. This might be approved by a more
complex model or by including the current accelera-
tion, when estimating the torque distribution. In ad-
dition, future work will investigate data-driven meth-
ods, such as neural networks, for their ability of esti-
mating the energy demand. Other advantages can be
assumed in involving more than one previous opera-
tion point, if they are given within the target applica-
tion of the prediction approach.
REFERENCES
Bellman, R. E. (2003). Dynamic programming. Dover Pub-
lications, Mineola, N.Y.
Binder, A. (2018). Elektrische Maschinen und Antriebe:
Grundlagen, Betriebsverhalten. Springer Berlin Hei-
delberg, Berlin, Heidelberg, 2. aufl. 2017 edition.
Elgowainy, A., editor (2021). Electric, Hybrid, and Fuel
Cell Vehicles. Springer eBook Collection. Springer
New York and Imprint Springer, New York, NY, 1st
ed. 2021 edition.
Fiori, C., Ahn, K., and Rakha, H. A. (2018). Micro-
scopic series plug-in hybrid electric vehicle energy
consumption model: Model development and valida-
tion. Transportation Research Part D: Transport and
Environment, 63:175–185.
Freuer, A. (2016). Ein Assistenzsystem f
¨
ur die en-
ergetisch optimierte L
¨
angsf
¨
uhrung eines Elektro-
fahrzeugs. Springer Fachmedien Wiesbaden, Wies-
baden.
HERE Maps (2021). https://www.here.com/platform. (last
viewed 03/11/2022).
H
¨
ulsebusch, D. (2018). Fahrerassistenzsysteme zur en-
ergieeffizienten L
¨
angsregelung - Analyse und Opti-
mierung der Fahrsicherheit. PhD thesis, Karlsruher
Institut f
¨
ur Technologie.
Mitschke, M. and Wallentowitz, H., editors (2004). Dy-
namik der Kraftfahrzeuge. VDI-Buch. Springer
Berlin Heidelberg, Berlin, Heidelberg and s.l., vierte,
neubearbeitete auflage edition.
Nelles, O. (2001). Nonlinear System Identification: From
Classical Approaches to Neural Networks and Fuzzy
Models. Springer eBook Collection. Springer, Berlin
and Heidelberg.
Pitanuwat, S., Aoki, H., IIzuka, S., and Morikawa, T.
(2019). Development of hybrid vehicle energy con-
sumption model for transportation applications—part
ii: Traction force-speed based energy consumption
modeling. World Electric Vehicle Journal, 10(2):22.
Radke, T. (2013). Energieoptimale L
¨
angsf
¨
uhrung
von Kraftfahrzeugen durch Einsatz vorausschauen-
der Fahrstrategien. Karlsruher Schriftenreihe
Fahrzeugsystemtechnik. KIT Scientific Publishing.
Rosenzweig, J. and Bartl, M. (2015). A review and analysis
of literature on autonomous driving. The Making of
Innovation, pages 1–57.
Sajadi-Alamdari, S. A., Voos, H., and Darouach, M. (2020).
Ecological advanced driver assistance system for opti-
mal energy management in electric vehicles. IEEE In-
telligent Transportation Systems Magazine, pages 92–
109.
Zhang, F., Wang, L., Coskun, S., Pang, H., Cui, Y., and Xi,
J. (2020). Energy management strategies for hybrid
electric vehicles: Review, classification, comparison,
and outlook. Energies, 13(13):3352.
Energy Demand Prediction in Hybrid Electrical Vehicles for Speed Optimization
123