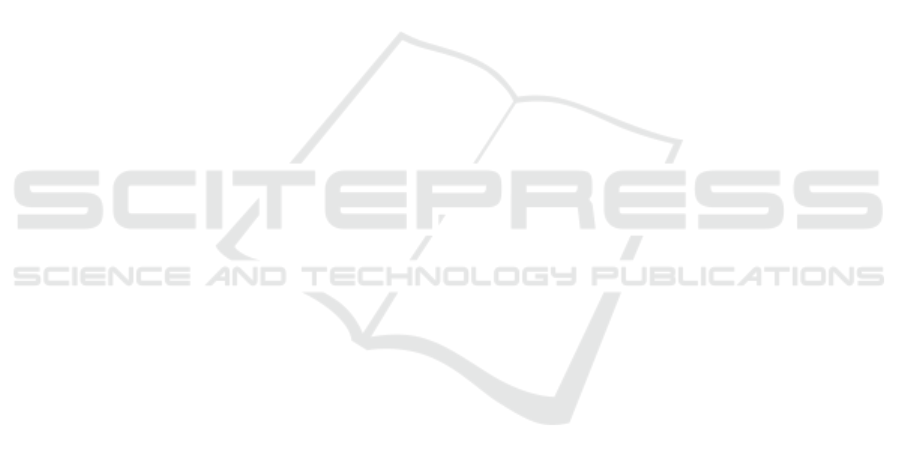
is created to train the LSTM model and includes ac-
tivities observed near a vehicle in pedestrian zones. A
full and divided approach for input skeleton data was
used, where the latter perform better. Furthermore,
the lower body classification is more accurate. How-
ever, it is noticeable that the model’s performance can
be significantly affected due to missing skeleton joints
or inaccurate joint data estimations, especially for the
upper body. A person carrying a cup or bag was rec-
ognized as texting as the model is unable to detect
objects. This could be solved by adding RGB data.
Understanding activities from pedestrians enables
an AV to make intelligent decisions based on the ac-
tivity identified. An AV does not need to stop for
a pedestrian parallel crossing towards the bus and is
aware. But it might be helpful for a person to warn by
voice command for a person using a phone to avoid
unnecessary stops; hence, reducing travel time for in-
side passengers. The model can be enhanced further
with more activities, for example, waiting, jogging,
including cyclists. Overall, the model shows better
results with the divided approach.
REFERENCES
Andriluka, M., Pishchulin, L., Gehler, P., and Schiele, B.
(2014). 2d human pose estimation: New bench-
mark and state of the art analysis. In Proceedings of
the IEEE Conference on computer Vision and Pattern
Recognition, pages 3686–3693.
Asuncion, A. and Newman, D. (2007). Uci machine learn-
ing repository.
Cao, Z., Hidalgo, G., Simon, T., Wei, S.-E., and Sheikh, Y.
(2019). Openpose: realtime multi-person 2d pose esti-
mation using part affinity fields. IEEE transactions on
pattern analysis and machine intelligence, 43(1):172–
186.
Chen, Y., Zhong, K., Zhang, J., Sun, Q., Zhao, X., et al.
(2016). Lstm networks for mobile human activity
recognition. In Proceedings of the 2016 International
Conference on Artificial Intelligence: Technologies
and Applications, Bangkok, Thailand, pages 24–25.
Duan, H., Zhao, Y., Chen, K., Shao, D., Lin, D., and Dai, B.
(2021). Revisiting skeleton-based action recognition.
arXiv preprint arXiv:2104.13586.
Geiger, A., Lenz, P., and Urtasun, R. (2012). Are we ready
for autonomous driving? the kitti vision benchmark
suite. In 2012 IEEE conference on computer vision
and pattern recognition, pages 3354–3361. IEEE.
Hariyono, J. and Jo, K.-H. (2015). Pedestrian action recog-
nition using motion type classification. In 2015 IEEE
2nd International Conference on Cybernetics (CYB-
CONF), pages 129–132. IEEE.
Hwang, S., Park, J., Kim, N., Choi, Y., and So Kweon, I.
(2015). Multispectral pedestrian detection: Bench-
mark dataset and baseline. In Proceedings of the IEEE
conference on computer vision and pattern recogni-
tion, pages 1037–1045.
Jan, Q. H. and Berns, K. (2021). Safety-configuration of
autonomous bus in pedestrian zone. In VEHITS, pages
698–705.
Jan, Q. H., Klein, S., and Berns, K. (2019). Safe and effi-
cient navigation of an autonomous shuttle in a pedes-
trian zone. In International Conference on Robotics in
Alpe-Adria Danube Region, pages 267–274. Springer.
Le, X.-H., Ho, H. V., Lee, G., and Jung, S. (2019). Applica-
tion of long short-term memory (lstm) neural network
for flood forecasting. Water, 11(7):1387.
Lin, T.-Y., Maire, M., Belongie, S., Hays, J., Perona, P.,
Ramanan, D., Doll
´
ar, P., and Zitnick, C. L. (2014).
Microsoft coco: Common objects in context. In Euro-
pean conference on computer vision, pages 740–755.
Springer.
Liu, J., Shahroudy, A., Perez, M., Wang, G., Duan, L.-Y.,
and Kot, A. C. (2019). Ntu rgb+ d 120: A large-
scale benchmark for 3d human activity understanding.
IEEE transactions on pattern analysis and machine
intelligence, 42(10):2684–2701.
Ojo, T., Adetona, C. O., Agyemang, W., and Afukaar, F. K.
(2019). Pedestrian risky behavior and safety at zebra
crossings in a ghanaian metropolitan area. Traffic in-
jury prevention, 20(2):216–219.
Pandey, P. and Aghav, J. V. (2020). Pedestrian activity
recognition using 2-d pose estimation for autonomous
vehicles. In ICT Analysis and Applications, pages
499–506. Springer.
Pop, D. O., Rogozan, A., Nashashibi, F., and Bensrhair,
A. (2017). Pedestrian recognition through differ-
ent cross-modality deep learning methods. In 2017
IEEE International Conference on Vehicular Elec-
tronics and Safety (ICVES), pages 133–138. IEEE.
Rasouli, A., Kotseruba, I., and Tsotsos, J. K. (2017). Are
they going to cross? a benchmark dataset and baseline
for pedestrian crosswalk behavior. In Proceedings of
the IEEE International Conference on Computer Vi-
sion Workshops, pages 206–213.
Sanchez-Caballero, A., de L
´
opez-Diz, S., Fuentes-Jimenez,
D., Losada-Guti
´
errez, C., Marr
´
on-Romera, M.,
Casillas-Perez, D., and Sarker, M. I. (2020). 3dfcnn:
Real-time action recognition using 3d deep neural net-
works with raw depth information. arXiv preprint
arXiv:2006.07743.
Shahroudy, A., Liu, J., Ng, T.-T., and Wang, G. (2016). Ntu
rgb+ d: A large scale dataset for 3d human activity
analysis. In Proceedings of the IEEE conference on
computer vision and pattern recognition, pages 1010–
1019.
Pedestrian Activity Recognition from 3D Skeleton Data using Long Short Term Memory Units
375