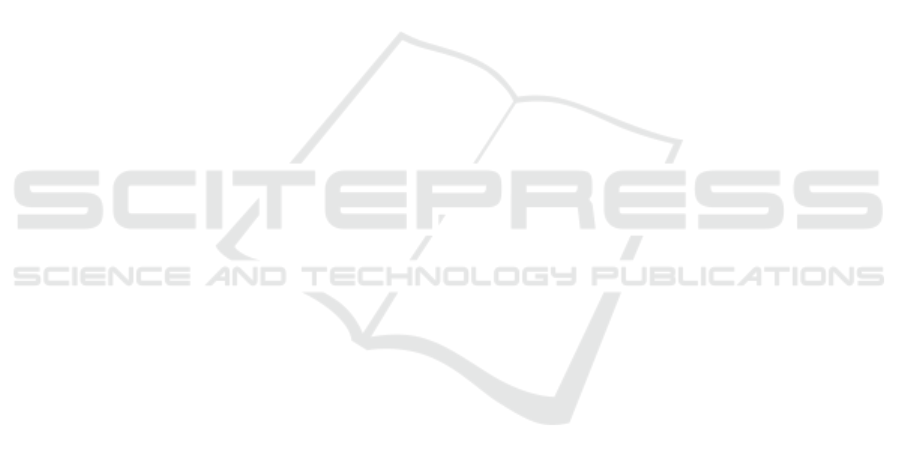
result of the experiment highlights that prediction of
zoom fatigue from the data collected by eye tracker
device and questionnaire has good accuracy for
classification models such as Ada-Boost, Logistic
Regression, SVM and Decision Tree. The feature set
in the research contains 24 variables, which includes
data from the eye tracker device, responses from the
questionnaire, and calculated PERCLOS. The results
of the experiment showed that the data collected by
eye tracker device and questionnaire attained the
highest accuracy in prediction of zoom fatigue with
Ada-Boost at 86% and SVM, Logistic Regression
and Decision Tree with an accuracy 71%. Zoom
fatigue is a form of mental fatigue that can be hard
on brains which makes brains exhausted quickly.
By determining these features new measures can be
practised or introduced for minimizing the effect of
zoom fatigue.
Overall, the research shows that MLETF is capa-
ble of predicting zoom fatigue in online users by the
data extracted from the eye tracker device and the re-
sponse from the questionnaire. The future work of
this research can be extended to the inclusion of more
details from the eye tracker device, and the addition of
personal and subjective traits of the online users. The
impact of length of video, distance from the screen
and eye can also be evaluated for detection of zoom
fatigue. Depending upon the model and license of the
eye tracker device, some attributes such as pupil dila-
tion, pupil fixation, etc. can be extracted from the ex-
periment, which might affect the prediction of zoom
fatigue. Also, subjective and personal traits such as
NASA-TLX can provide details and the effect of cog-
nitive load over an online user’s zoom fatigue. More-
over, this approach can be used to detect zoom fatigue
in domains such as medical, engineer, driving, etc.
REFERENCES
Acı, C¸ .
˙
I., Kaya, M., and Mishchenko, Y. (2019). Dis-
tinguishing mental attention states of humans via an
eeg-based passive bci using machine learning meth-
ods. Expert Systems with Applications, 134:153–166.
Barrios, V. M. G., G
¨
utl, C., Preis, A. M., Andrews, K.,
Pivec, M., M
¨
odritscher, F., and Trummer, C. (2004).
Adele: A framework for adaptive e-learning through
eye tracking. Proceedings of IKNOW, pages 609–616.
Boksem, M. A., Meijman, T. F., and Lorist, M. M. (2006).
Mental fatigue, motivation and action monitoring. Bi-
ological psychology, 72(2):123–132.
Cheng, Q., Wang, W., Jiang, X., Hou, S., and Qin, Y.
(2019). Assessment of driver mental fatigue using fa-
cial landmarks. IEEE Access, 7:150423–150434.
Cui, Z., Sun, H.-M., Yin, R.-N., Gao, L., Sun, H.-B.,
and Jia, R.-S. (2021). Real-time detection method
of driver fatigue state based on deep learning of face
video. Multimedia Tools and Applications, pages 1–
21.
Esposito, F., Otto, T., Zijlstra, F. R., and Goebel, R. (2014).
Spatially distributed effects of mental exhaustion on
resting-state fmri networks. PLoS One, 9(4):e94222.
Fauville, G., Luo, M., Muller Queiroz, A. C., Bailenson,
J. N., and Hancock, J. (2021). Zoom exhaustion &
fatigue scale. Available at SSRN 3786329.
Gao, X.-Y., Zhang, Y.-F., Zheng, W.-L., and Lu, B.-L.
(2015). Evaluating driving fatigue detection algo-
rithms using eye tracking glasses. In 2015 7th In-
ternational IEEE/EMBS Conference on Neural Engi-
neering (NER), pages 767–770. IEEE.
He, J., Choi, W., Yang, Y., Lu, J., Wu, X., and Peng, K.
(2017). Detection of driver drowsiness using wearable
devices: A feasibility study of the proximity sensor.
Applied ergonomics, 65:473–480.
Intayoad, W., Becker, T., and Temdee, P. (2017). So-
cial context-aware recommendation for personalized
online learning. Wireless Personal Communications,
97(1):163–179.
Ivanovi
´
c, M., Kla
ˇ
snja-Mili
´
cevi
´
c, A., Ivkovi
´
c, J., and Porta,
M. (2017). Integration of eye tracking technologies
and methods in an e-learning system. In Proceedings
of the 8th Balkan Conference in Informatics, pages 1–
4.
Joe Louis Paul, I., Sasirekha, S., Uma Maheswari, S., Ajith,
K., Arjun, S., and Athesh Kumar, S. (2019). Eye
gaze tracking-based adaptive e-learning for enhancing
teaching and learning in virtual classrooms. In Infor-
mation and Communication Technology for Competi-
tive Strategies, pages 165–176. Springer.
Jonsson, M. and Brown, J. (2021). Deep learning for
driver sleepiness classification using bioelectrical sig-
nals and karolinska sleepiness scale.
Kacur, J., Polec, J., Csoka, F., and Smolejova, E. (2019).
Gmm based detection of schizophrenia using eye
tracking. In 2019 IEEE Conference on Computational
Intelligence in Bioinformatics and Computational Bi-
ology (CIBCB), pages 1–4. IEEE.
Li, J., Li, H., Umer, W., Wang, H., Xing, X., Zhao, S.,
and Hou, J. (2020). Identification and classification
of construction equipment operators’ mental fatigue
using wearable eye-tracking technology. Automation
in Construction, 109:103000.
Lowndes, B. R., Forsyth, K. L., Blocker, R. C., Dean, P. G.,
Truty, M. J., Heller, S. F., Blackmon, S., Hallbeck,
M. S., and Nelson, H. (2020). Nasa-tlx assessment of
surgeon workload variation across specialties. Annals
of surgery, 271(4):686–692.
Monteiro, T. G., Zhang, H., Skourup, C., and Tannuri, E. A.
(2019). Detecting mental fatigue in vessel pilots using
deep learning and physiological sensors. In 2019 ieee
15th international conference on control and automa-
tion (icca), pages 1511–1516. IEEE.
Morris, B. (2020). Why does zoom exhaust you? science
has an answer. Wall Street Journal, 27.
CSEDU 2022 - 14th International Conference on Computer Supported Education
194