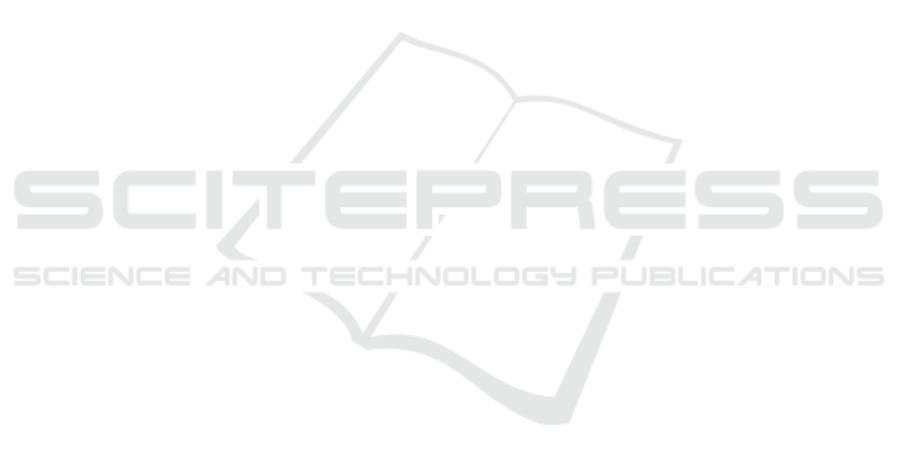
mated vehicles. IEEE Intelligent Vehicles Symposium,
Proceedings, 2018-June:1813–1820.
Bock, F., Sippl, C., Heinz, A., Lauer, C., and German,
R. (2019). Advantageous usage of textual domain-
specific languages for scenario-driven development of
automated driving functions. In 2019 IEEE Interna-
tional Systems Conference, SysCon 2019. IEEE.
Bock, J., Krajewski, R., Eckstein, L., Klimke, J., Sauerbier,
J., and Zlocki, A. (2018). Data basis for scenario-
based validation of had on highways. In 27th Aachen
colloquium automobile and engine technology.
de Gelder, E. and Camp, O. O. d. (2020). Tagging real-
world scenarios for the assessment of autonomous ve-
hicles. arXiv preprint arXiv:2012.01081.
de Gelder, E., Manders, J., Grappiolo, C., Paardekooper,
J.-P., Camp, O. O. d., and De Schutter, B. (2020).
Real-world scenario mining for the assessment of au-
tomated vehicles. arXiv preprint arXiv:2006.00483.
de Gelder, E., Paardekooper, J.-P., Op den Camp, O., and
De Schutter, B. (2019). Safety assessment of auto-
mated vehicles: how to determine whether we have
collected enough field data? Traffic injury prevention,
20(sup1).
Elspas, P., Klose, Y., Isele, S. T., Bach, J., and Sax, E.
(2021). Time series segmentation for driving scenario
detection with fully convolutional networks. In Pro-
ceedings of the 7th International Conference on Vehi-
cle Technology and Intelligent Transport Systems, VE-
HITS 2021. SCITEPRESS.
Elspas, P., Langner, J., Aydinbas, M., Bach, J., and Sax,
E. (2020). Leveraging regular expressions for flexible
scenario detection in recorded driving data. In 2020
IEEE International Symposium on Systems Engineer-
ing (ISSE). IEEE.
Fayyad, U. M., Piatetsky-Shapiro, G., and Smyth, P. (1996).
Knowledge discovery and data mining: Towards a
unifying framework. In Proceedings of the Second In-
ternational Conference on Knowledge Discovery and
Data Mining (KDD-96), pages 82–88. AAAI Press.
Friedl, J. E. (2006). Mastering regular expressions.
O’Reilly Media, Inc.
Hartjen, L., Philipp, R., Schuldt, F., and Friedrich, B.
(2020). Saturation effects in recorded maneuver data
for the test of automated driving.
Hauer, F., Schmidt, T., Holzm
¨
uller, B., and Pretschner, A.
(2019). Did we test all scenarios for automated and
autonomous driving systems? In 2019 IEEE Intelli-
gent Transportation Systems Conference, ITSC 2019.
IEEE.
Koopman, P. and Fratrik, F. (2019). How many operational
design domains, objects, and events? In Workshop
on Artificial Intelligence Safety 2019, volume 2301 of
CEUR Workshop Proceedings. CEUR-WS.org.
Langner, J., Grolig, H., Otten, S., Holz
¨
apfel, M., and
Sax, E. (2019). Logical scenario derivation by clus-
tering dynamic-length-segments extracted from real-
world-driving-data. In Proceedings of the 5th Inter-
national Conference on Vehicle Technology and Intel-
ligent Transport Systems, VEHITS 2019. SciTePress.
Menzel, T., Bagschik, G., Isensee, L., Schomburg, A., and
Maurer, M. (2019). From functional to logical scenar-
ios: detailing a keyword-based scenario description
for execution in a simulation environment. In 2019
IEEE Intelligent Vehicles Symposium (IV). IEEE.
Menzel, T., Bagschik, G., and Maurer, M. (2018). Scenarios
for development, test and validation of automated ve-
hicles. In 2018 IEEE Intelligent Vehicles Symposium,
IV 2018. IEEE.
Montanari, F., German, R., and Djanatliev, A. (2020). Pat-
tern recognition for driving scenario detection in real
driving data. In IEEE Intelligent Vehicles Symposium,
IV 2020. IEEE.
Montanari, F., Ren, H., and Djanatliev, A. (2021). Scenario
detection in unlabeled real driving data with a rule-
based state machine supported by a recurrent neural
network. In 93rd IEEE Vehicular Technology Confer-
ence, VTC 2021. IEEE.
M
¨
orchen, F. (2006). Time series knowledge mining.
PEGASUS Project (2019). The PEGASUS method. [On-
line; accessed 05-December-2021].
P
¨
utz, A., Zlocki, A., Bock, J., and Eckstein, L. (2017).
System validation of highly automated vehicles with
a database of relevant traffic scenarios. 12th ITS Eu-
ropean Congress.
Riedmaier, S., Ponn, T., Ludwig, D., Schick, B., and Dier-
meyer, F. (2020). Survey on scenario-based safety as-
sessment of automated vehicles. IEEE Access, 8.
Ries, L., Langner, J., Otten, S., Bach, J., and Sax, E. (2019).
A driving scenario representation for scalable real-
data analytics with neural networks. In 2019 IEEE
Intelligent Vehicles Symposium, IV 2019. IEEE.
Rodrigues, J., Folgado, D., Belo, D., and Gamboa, H.
(2019). SSTS: A syntactic tool for pattern search on
time series. Inf. Process. Manag., 56(1).
Scholtes, M., Westhofen, L., Turner, L. R., Lotto, K.,
Schuldes, M., Weber, H., Wagener, N., Neurohr, C.,
Bollmann, M., K
¨
ortke, F., Hiller, J., Hoss, M., Bock,
J., and Eckstein, L. (2021). 6-layer model for a struc-
tured description and categorization of urban traffic
and environment. IEEE Access, 9.
Silberschatz, A., Korth, H. F., and Sudarshan, S.
(2020). Database System Concepts, Seventh Edition.
McGraw-Hill Book Company.
Ulbrich, S., Menzel, T., Reschka, A., Schuldt, F., and Mau-
rer, M. (2015). Defining and substantiating the terms
scene, situation, and scenario for automated driving.
In 2015 IEEE 18th International Conference on In-
telligent Transportation Systems, volume 2015-Octob.
IEEE.
VDA QMC Working Group 13 / Automotive SIG (2017).
Automotive SPICE Process Assessment / Reference
Model.
Wirth, R. and Hipp, J. (2000). Crisp-dm: Towards a stan-
dard process model for data mining. In Proceedings of
the 4th international conference on the practical ap-
plications of knowledge discovery and data mining.
Springer-Verlag London, UK.
Towards a Scenario Database from Recorded Driving Data with Regular Expressions for Scenario Detection
409