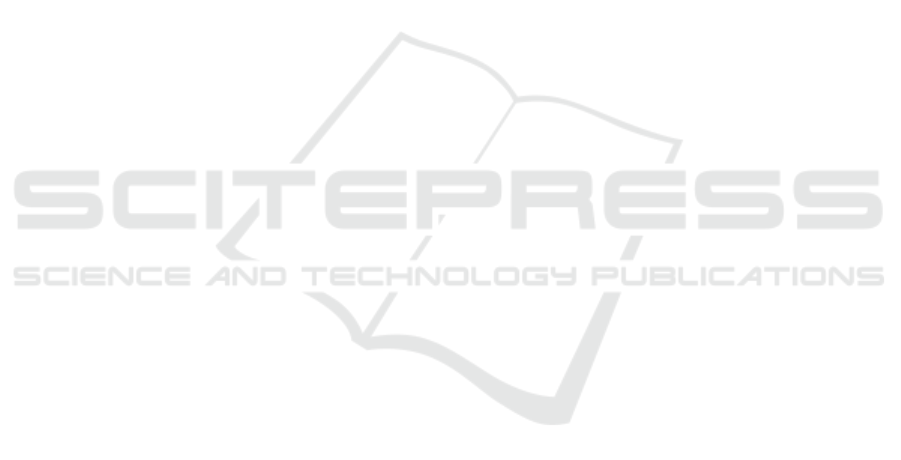
ings of the 1st Conference of the Asia-Pacific Chap-
ter of the Association for Computational Linguistics
and the 10th International Joint Conference on Natu-
ral Language Processing.
Devlin, J., Chang, M., Lee, K., and Toutanova, K. (2018).
BERT: pre-training of deep bidirectional transformers
for language understanding. CoRR, abs/1810.04805.
Ferri, P. (2019). Mooc, digital university teaching and learn-
ing analytics. opportunities and perspectives. ITAL-
IAN JOURNAL OF EDUCATIONAL RESEARCH,
page 13–26.
Fincham, E., Whitelock-Wainwright, A., Kovanovi
´
c, V.,
Joksimovi
´
c, S., van Staalduinen, J.-P., and Ga
ˇ
sevi
´
c,
D. (2019). Counting Clicks is Not Enough: Validating
a Theorized Model of Engagement in Learning Ana-
lytics. In Proceedings of the 9th International Con-
ference on Learning Analytics & Knowledge, pages
501–510, Tempe AZ USA. ACM.
Joksimovi
´
c, S., Poquet, O., Kovanovi
´
c, V., Dowell, N.,
Mills, C., Ga
ˇ
sevi
´
c, D., Dawson, S., Graesser, A. C.,
and Brooks, C. (2018). How Do We Model Learn-
ing at Scale? A Systematic Review of Research on
MOOCs. Review of Educational Research, 88(1):43–
86. Publisher: American Educational Research Asso-
ciation.
Joksimovic, S., Jovanovic, J., Kovanovic, V., Gasevic,
D., Milikic, N., Zouaq, A., and Van Staalduinen, J.
(2020). Comprehensive analysis of discussion fo-
rum participation: from speech acts to discussion dy-
namics and course outcomes. IEEE Transactions on
Learning Technologies, 13(1):38–51.
Joulin, A., Grave, E., Bojanowski, P., and Mikolov, T.
(2016). Bag of tricks for efficient text classification.
arXiv preprint arXiv:1607.01759.
Le, Q. and Mikolov, T. (2014). Distributed Representations
of Sentences and Documents. In International Confer-
ence on Machine Learning, pages 1188–1196. PMLR.
ISSN: 1938-7228.
Martinc, M., Pollak, S., and Robnik-
ˇ
Sikonja, M.
(2021). Supervised and unsupervised neural ap-
proaches to text readability. Computational Linguis-
tics, 47(1):141–179.
Mikolov, T., Sutskever, I., Chen, K., Corrado, G. S., and
Dean, J. (2013). Distributed representations of words
and phrases and their compositionality. In Burges, C.
J. C., Bottou, L., Welling, M., Ghahramani, Z., and
Weinberger, K. Q., editors, Advances in Neural Infor-
mation Processing Systems, volume 26. Curran Asso-
ciates, Inc.
Moreno-Marcos, P. M., Mu
˜
noz-Merino, P. J., Maldonado-
Mahauad, J., P
´
erez-Sanagust
´
ın, M., Alario-Hoyos, C.,
and Delgado Kloos, C. (2020). Temporal analysis
for dropout prediction using self-regulated learning
strategies in self-paced moocs. Computers & Educa-
tion, 145:103728.
Piolat, A., Booth, R., Chung, C., Davids, M., and Pen-
nebaker, J. (2011). La version franc¸aise du dictio-
nnaire pour le LIWC : modalit
´
es de construction et
exemples d’utilisation. Psychologie Francaise - PSY-
CHOL FR, 56:145–159.
Ramesh, A., Goldwasser, D., Huang, B., III, H. D., and
Getoor., L. (2013). Modeling learner engagement in
moocs using probabilistic soft logic.
Reschly, A. L. and Christenson, S. L. (2012). Jingle, Jan-
gle, and Conceptual Haziness: Evolution and Future
Directions of the Engagement Construct. In Chris-
tenson, S. L., Reschly, A. L., and Wylie, C., editors,
Handbook of Research on Student Engagement, pages
3–19. Springer US, Boston, MA.
Salazar, J., Liang, D., Nguyen, T. Q., and Kirchhoff, K.
(2020). Masked language model scoring. Proceed-
ings of the 58th Annual Meeting of the Association for
Computational Linguistics.
Tausczik, Y. R. and Pennebaker, J. W. (2010). The psy-
chological meaning of words: Liwc and computerized
text analysis methods. Journal of Language and So-
cial Psychology, 29(1):24–54.
Tucker, C., Pursel, B., and Divinsky, A. (2014). Min-
ing Student-Generated Textual Data In MOOCS And
Quantifying Their Effects on Student Performance
and Learning Outcomes Mining Student-Generated
Textual Data in MOOCS and Quantifying Their Ef-
fects on Student Performance and Learning Out-
comes. Computers in Education Journal, 5:84–95.
Wen, M., Yang, D., and Ros
´
e, C. (2014a). Linguistic Reflec-
tions of Student Engagement in Massive Open Online
Courses.
Wen, M., Yang, D., and Ros
´
e, C. (2014b). Sentiment anal-
ysis in MOOC discussion forums: What does it tell
us? Proceedings of Educational Data Mining, pages
130–137.
Whitehill, J., Williams, J., Lopez, G., Coleman, C., and Re-
ich, J. (2015). Beyond Prediction: First Steps Toward
Automatic Intervention in MOOC Student Stopout.
Wise, A. F. and Cui, Y. (2018). Learning communities in the
crowd: Characteristics of content related interactions
and social relationships in MOOC discussion forums.
Computers & Education, 122(1):221–242. Publisher:
Elsevier Ltd.
Yang, D., Wen, M., Howley, I., Kraut, R., and Rose, C.
(2015). Exploring the Effect of Confusion in Discus-
sion Forums of Massive Open Online Courses. pages
121–130.
CSEDU 2022 - 14th International Conference on Computer Supported Education
104