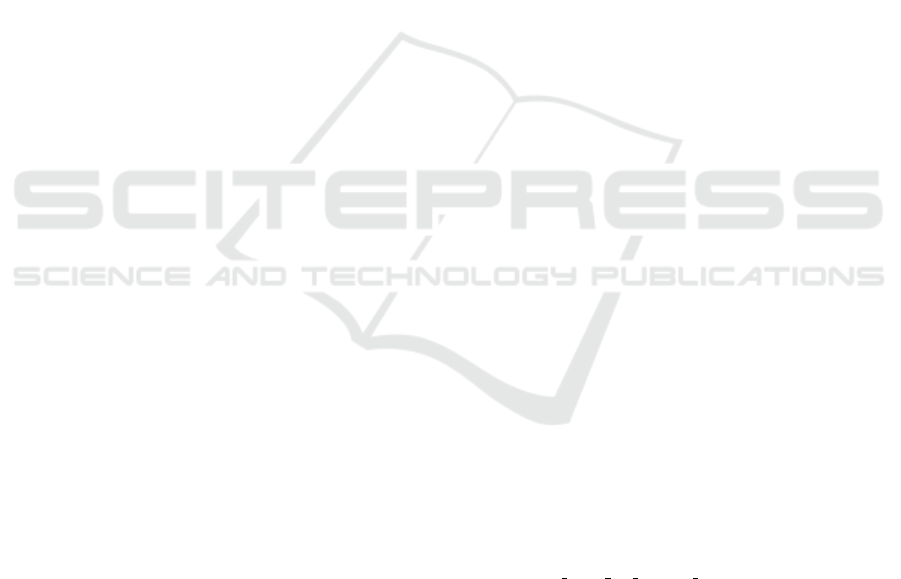
consequence is that some more effort will need to be
invested in order to improve the usability in terms of
system setup. The good achievement is that the main
novel characteristics of the platform have been well
received and considered relevant and useful by the ex-
perts.
Figure 5 shows the positioning of the BRAIN-IoT
Platform relative to the benchmark. The results show
the good feeling w.r.t. the Novelty and Dependabil-
ity, for which the score is Excellent. Considering the
benchmark refers to solutions in every Information
and Communication Technologies (ICT) field, meant
to be commercial products, the curve of the BRAIN-
IoT platform is in line with the expectation of a re-
search and innovation action, where the maturity of
the developed solution is still about around TRL 6,
but the innovative aspects are well evident.
A similar evaluation has been performed to evalu-
ate the software within the scope of the two applica-
tion scenarios. When applied to the Service Robotics
scenario, despite the perspicuity remains in line with
the values determined before, the attractiveness is
much higher. This is because the perception may
change a lot when you think about the technology ap-
plied to a specific context, and the benefits that the
platform could bring are much more evident. Basi-
cally, the stakeholder but himself in the shoes of the
person who will benefit from the execution of the plat-
form, instead of the IT manager who is supposed to
install and setup the system. This means that, despite
the BRAIN-IoT Platform continues to be evaluated as
complicate to setup, it is considered good for manag-
ing swarm robotics applications.
Figure 6 shows the positioning of the BRAIN-IoT
Platform relative to the benchmark. It shows even
clearly how the BRAIN-IoT Platform is considered
above the average in a context of SRWL. Instead,
the dependability is decreased because of the secu-
rity perspective in this context is more in line with
the state of the art technologies. As for the SRWL
scenario, a similar analysis could be made for the CI-
WDM. However, differently from the other scenario,
here the perspicuity is higher: while for the SRWL
scenario, the robotic applications developer cannot ig-
nore the system setup phase, in the case of the man-
agement of the CIWDM, the platform is evaluated
from the perspective of the pure end-user. Figure
7 shows the positioning of the BRAIN-IoT Platform
relative to the benchmark. In this case, the platform
has been evaluated as beneficial for managing a criti-
cal infrastructure, especially considering the security
features and the resiliency capabilities.
8 CONCLUSION
BRAIN-IoT platform aims to pave the way of the
research around the strict requirements of the next-
generation IoT paradigm(AIOTI, 2020). In other
words BRAIN-IoT platform is a first implementa-
tion of a meta operating system for the IoT domain,
which facilitates the implementation of secure and
self-adaptive IoT applications. The platform has been
tested and applied to robotics scenarios in which
BRAIN-IoT enables the self-controlled robots inter-
act with the environment and adopt their behaviors
correspondingly. In addition, BRAIN-IoT platform
demonstrated its advanced features in industry and
agile critical infrastructures management especially
for the physical infrastructure monitoring, and abnor-
mal behavior detection and prediction. Such meta op-
erating system maps toward the 3D architecture de-
fined by AIOTI but some functionalities still need to
be either covered or enhanced, such as safety, trust-
worthiness. As part of the future works, the authors
plan to extend the BRAIN-IoT platform implement-
ing these functionalities with the aim to implement a
full featured meta operating system for the IoT.
ACKNOWLEDGMENT
The research leading to these results has been sup-
ported by the European Union through the BRAIN-
IoT project H2020-EU.2.1.1. Grant agreement ID:
780089.
REFERENCES
Adolphs, P. and Epple, U. (2015). Reference architecture
model industrie 4.0 (rami4.0). Standard, ZVEI – Ger-
man Electrical and Electronic Manufacturers’ Associ-
ation.
AIOTI (2020). High Level Architecture (HLA).
Standard, AIOTI WG Standardisation.
https://aioti.eu/wp-content/uploads/2020/12/
AIOTI HLA R5 201221 Published.pdf.
Basu, A., Bensalem, S., Bozga, M., Combaz, J., Jaber,
M., Nguyen, T.-H., and Sifakis, J. (2011). Rigor-
ous Component-Based System Design Using the BIP
Framework. IEEE Software, 28(3):41–48.
Bauer, M., Boussard, M., Bui, N., Carrez, F., Jardak, C.,
Loof, D., Magerkurth, C., Meissner, S., Nettstr
¨
ater,
A., Olivereau, A., Thoma, M., Walewski, Stefa, J.,
and Salinas, A. (2013). Internet of things – architec-
ture iot-a deliverable d1.5 – final architectural refer-
ence model for the iot v3.0. Technical report.
Chehida, S., Baouya, A., Alonso, D. F., Brun, P.-E., Mas-
sot, G., Bozga, M., and Bensalem, S. (2021). Asset-
IoTBDS 2022 - 7th International Conference on Internet of Things, Big Data and Security
76