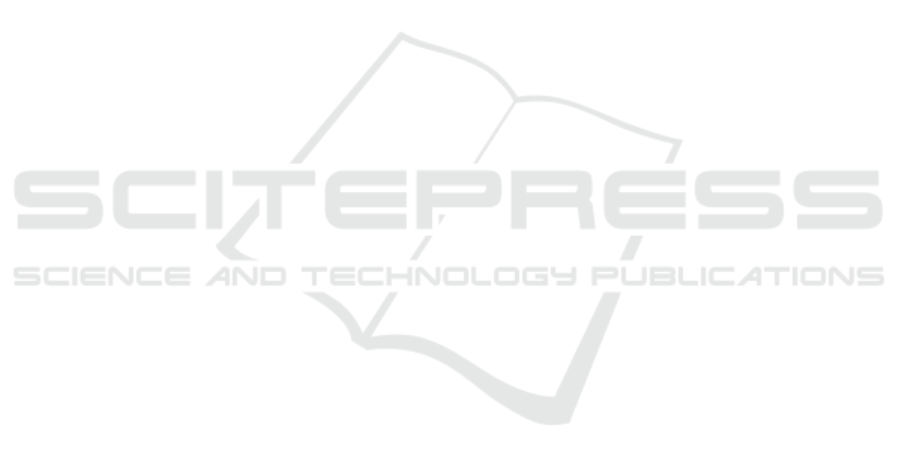
havior for each profile. In this sense, the proposal
of this work satisfactorily meets the need and per-
sonalizes personalized pedagogical actions in an au-
tomated manner. From this, we understand that in
future works, it is necessary to improve the adapta-
tion functions (F
1
and F
2
) to provide pedagogical se-
quences more adjusted to the students’ profiles.
6 CONCLUSIONS
This paper presents an approach to automatically se-
quencing customized pedagogical actions to the stu-
dent. Using two cognitive theories, such as the Re-
vised Bloom Taxonomy and the student’s RASI pro-
file, it was possible to sequence actions in an inde-
pendent way of the curriculum structure, considering
the learning process. Using digital activities provided
by Bloom’s Digital Taxonomy, a satisfaction experi-
ment was carried out in which sequences of activities
were recommended for students from the sequences
of actions generated by the PSO.
An important finding of this work concerns the
feasibility of personalized pedagogical recommenda-
tions through digital activities that consider a student
RASI profile. Thus, it was shown that the relation-
ship established between the BT and the RASI is an
effective approach to solve this problem. From the
results of the experiment, it was concluded that stu-
dents were satisfied with the quantity and quality of
activities recommended by the PSO.
In this sense, the binary PSO developed from the
proposed methodology proved to be an efficient ap-
proach to solve the sequencing problem. The op-
timization process was able to find sequences com-
posed of actions that were relevant and in adequate
quantity for each student. A limitation in this study
is the discrepancy between the predominant profiles
of the students who participated in the experiment,
which requires a more in-depth statistical analysis of
the data obtained. As future works, we intend to feed-
back the reference values of the optimization objec-
tives from the satisfaction survey results and carry out
the integration with a Virtual Learning Environment
in order to automate the recommendation process.
ACKNOWLEDGMENT
The authors thank the Federal University of
Uberl
ˆ
andia, the Goiano Federal Institute, and the Fed-
eral Institute of Tri
ˆ
angulo Mineiro for supporting this
research.
REFERENCES
Al-Muhaideb, S. and Menai, M. E. B. (2011). Evolutionary
computation approaches to the curriculum sequencing
problem. Natural Computing, 10(2):891–920.
Brown, M., McCormack, M., Reeves, J., Brook, D. C., Gra-
jek, S., Alexander, B., Bali, M., Bulger, S., Dark, S.,
Engelbert, N., et al. (2020). 2020 educause horizon
report teaching and learning edition. Technical report,
EDUCAUSE.
Chu, C.-P., Chang, Y.-C., and Tsai, C.-C. (2011). Pc 2 pso:
personalized e-course composition based on particle
swarm optimization. Applied Intelligence, 34(1):141–
154.
Churches, A. (2010). Bloom’s digital taxonomy.
da Costa, N. T. and Fernandes, M. A. (2021). Sequenci-
amento de ac¸
˜
oes pedag
´
ogicas baseadas na taxonomia
de bloom usando planejamento automatizado apoiado
por algoritmo gen
´
etico. Revista Brasileira de In-
form
´
atica na Educac¸
˜
ao, 29:485–501.
De-Marcos, L., Mart
´
ınez, J. J., Guti
´
errez, J. A., Barchino,
R., and Guti
´
errez, J. M. (2009). A new sequenc-
ing method in web-based education. In 2009 IEEE
Congress on Evolutionary Computation, pages 3219–
3225. IEEE.
Engelbrecht, A. P. (2006). Fundamentals of Computational
Swarm Intelligence. John Wiley & Sons, Inc., Hobo-
ken, NJ, USA.
Entwistle, N. (2018). Student learning and academic under-
standing: A research perspective with implications for
teaching. Academic Press.
Entwistle, N. and Tait, H. (2013). Approaches and study
skills inventory for students (assist) (incorporating the
revised approaches to studying inventory - rasi). Edin-
burgh: Centre for Research on Learning and Instruc-
tion, University of Edinburgh.
Fusilier, M., Bhuyan, R., Russell, J., Lin, S., and Yang,
S. (2021). Studying approaches: samples in china,
kuwait, and usa. Journal of Applied Research in
Higher Education, ahead-of-print.
Go
ˇ
stautait
˙
e, D. (2019). Principal component analysis
and bloom taxonomy to personalise learning. In
EDULEARN19 Proceedings 11th International Con-
ference on Education and New Learning Technolo-
gies: Palma, Spain. 1-3 July, 2019, pages 2910–2920.
IATED Academy.
Huang, R., Spector, J. M., and Yang, J. (2019). Educational
technology: a primer for the 21st century. Springer.
Kennedy, J. and Eberhart, R. (1995). Particle swarm opti-
mization. In Proceedings of ICNN’95 - International
Conference on Neural Networks, volume 4, pages
1942–1948 vol.4.
Kennedy, J. and Eberhart, R. C. (1997). A discrete bi-
nary version of the particle swarm algorithm. In 1997
IEEE International conference on systems, man, and
cybernetics. Computational cybernetics and simula-
tion, volume 5, pages 4104–4108. IEEE.
Kennedy, J. F., Eberhart, R. C., and Shi, Y. (2001). Swarm
intelligence. The Morgan Kaufmann series in evo-
Sequencing and Recommending Pedagogical Activities from Bloom’s Taxonomy using RASI and Multi-objective PSO
115