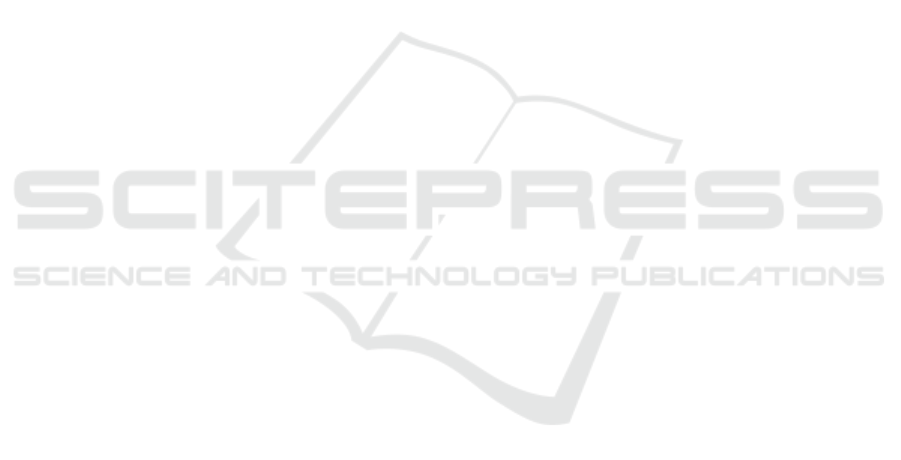
the method of content-based filtering to a given work-
list and the event log and present the result, a work-
list, ordered according to potential user preferences,
to the active user of the PAIS. An advantage of this
approach is that existing data and information like the
process model or the event log can be used to cal-
culate the user’s preferences. Furthermore, activities
of the process model can be enhanced by other tags
for more accurate predictions. We evaluated our work
prototypically with our project partners. The idea
received a lot of positive feedback, and the project
partners confirmed that the presented idea leads to an
improvement in user satisfaction. Initial prototypical
implementations and evaluations showed the success
of the approach. Manual calculations of recommen-
dations provided promising results demonstrating the
need for implementation.
In future work, we will extend our approach by
alternate activity rating approaches as well as more
approaches to generating activity tags. Furthermore,
other methods known from recommender systems can
be considered to tackle drawbacks, like cold-start
problem for new users, or recommendation of appar-
ent items, of content-based filtering method, e.g., col-
laborative filtering or knowledge-based filtering. Fi-
nally, we plan to implement a prototype and to include
a real-world evaluation of our approach.
REFERENCES
van der Aalst, W. M. P. (2016). Process Mining: Data Sci-
ence in Action. Springer, 2 edition.
van der Aalst, W. M. P., Pesic, M., and Song, M. (2010).
Beyond process mining: From the past to present and
future. In Proc. of the 22nd International Confer-
ence on Advanced Information Systems Engineering
(CAiSE ’10), volume 6051.
Aggarwal, C. C. (2016). Recommender Systems - The Text-
book. Springer.
Barba, I., Weber, B., and Valle, C. D. (2012). Supporting
the optimized execution of business processes through
recommendations. In Proc. BPI, volume 99, pages
135–140.
Bellet, C. S., Neve, J.-E. D., and Ward, G. (2019). Does
employee happiness have an impact on productivity?
Sa
¨
ıd Business School WP.
Bidar, R., ter Hofstede, A. H. M., Sindhgatta, R., and
Ouyang, C. (2019). Preference-based resource and
task allocation in business process automation. In
OTM 2019, volume 11877, pages 404–421.
Bryson, A., Forth, J., and Stokes, L. (2015). Does worker
wellbeing affect workplace performance? Technical
report, Department for Business, Innovation & Skills.
Cabanillas, C., Garc
´
ıa, J. M., Resinas, M., Ruiz, D.,
Mendling, J., and Cort
´
es, A. R. (2013). Priority-based
human resource allocation in business processes. In
ICSOC 2013, volume 8274, pages 374–388.
Chomboon, K., Chujai, P., Teerarassammee, P., Kerdpra-
sop, K., and Kerdprasop, N. (2015). An empirical
study of distance metrics for k-nearest neighbor algo-
rithm. In Proc. of the 3rd International Conference on
Industrial Application Engineering, pages 280–285.
Conforti, R., de Leoni, M., Rosa, M. L., van der Aalst, W.
M. P., and ter Hofstede, A. H. M. (2015). A recom-
mendation system for predicting risks across multi-
ple business process instances. Decis. Support Syst.,
69:1–19.
Dumas, M., Rosa, M. L., Mendling, J., and Reijers, H. A.
(2018). Fundamentals of Business Process Manage-
ment. Springer, 2nd edition.
Garc
´
ıa, J. M., Ruiz, D., and Ruiz-Cort
´
es, A. (2010). A
model of user preferences for semantic services dis-
covery and ranking. In The Semantic Web: Research
and Applications, volume 6089, pages 1–14.
Goldsmith, J. and Junker, U. (2008). Preference handling
for artificial intelligence. AI Magazine, 29:9–12.
Guo, Z., Zeng, W., Wang, H., and Shen, Y. (2019). An
enhanced group recommender system by exploiting
preference relation. IEEE Access, PP:1–1.
Haisjackl, C. and Weber, B. (2010). User assistance during
process execution - an experimental evaluation of rec-
ommendation strategies. In Proc. of BPI Workshop,
volume 66, pages 134–145.
Huber, S., Fietta, M., and Hof, S. (2015). Next step recom-
mendation and prediction based on process mining in
adaptive case management. In 7th International Con-
ference on Subject-Oriented Business Process Man-
agement.
Jablonski, S. and Bussler, C. (1996). Workflow Manage-
ment: Modeling Concepts, Architecture, and Imple-
mentation.
Koulopolous, T. M. (1995). The Workflow Imperative:
Building Real World Business Solutions.
Lawrence, P. (1997). Workflow Handbook.
Manouselis, N. and Costopoulou, C. (2007). Analysis and
classification of multi-criteria recommender systems.
World Wide Web, 10(4):415–441.
Marin-Castro, H. M. and Tello-Leal, E. (2021). Event log
preprocessing for process mining: A review. Applied
Sciences, 11.
Nguyen, H. and Haddawy, P. (1998). Diva: Applying de-
cision theory to collaborative filtering. In Proc. of the
AAAI Workshop on Recommender Systems.
Novak, J. M. (1999). Choice theory: A new psychology
of personal freedom. Brock Education: a Journal of
Educational Research and Practice, 9.
OMG (2011). Business Process Model and Notation
(BPMN), Version 2.0.
Pflug, J. and Rinderle-Ma, S. (2015). Analyzing the effects
of reordering work list items for selected control flow
patterns. In IEEE 19th International Enterprise Dis-
tributed Object Computing Workshop, pages 14–23.
Pichler, H. and Edre, J. (2019). Towards look-ahead strate-
gies for work item selection. 2019 IEEE Jordan Inter-
national Joint Conference on Electrical Engineering
and Information Technology (JEEIT), pages 752–757.
Content-based Filtering for Worklist Reordering to improve User Satisfaction: A Position Paper
595