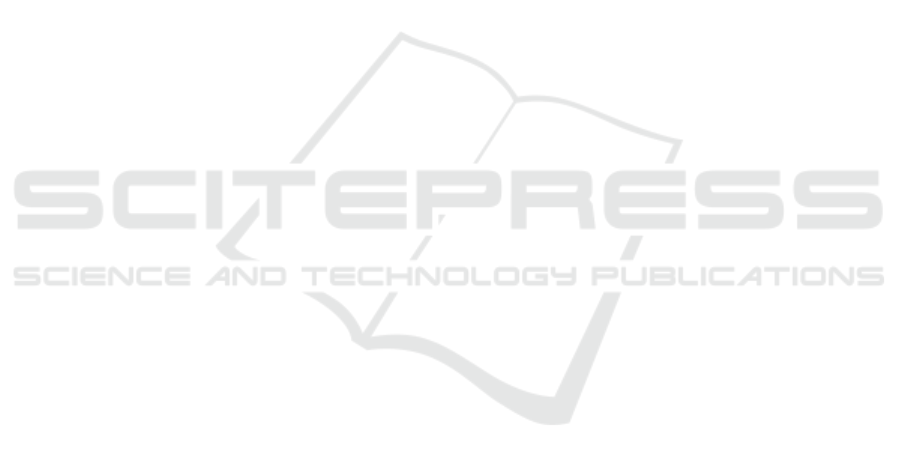
REFERENCES
Bang, S. H., Ak, R., Narayanan, A., Lee, Y. T., and Cho,
H. (2019). A survey on knowledge transfer for man-
ufacturing data analytics. Computers in Industry,
104:116–130.
Bifet, A. and Gavald
`
a, R. (2007). Learning from time-
changing data with adaptive windowing. In Proceed-
ings of the 2007 SDM.
Boiko Ferreira, L. E., Murilo Gomes, H., Bifet, A., and
Oliveira, L. S. (2019). Adaptive random forests with
resampling for imbalanced data streams. In 2019
IJCNN, pages 1–6.
Bordes, A., Ertekin, S., Weston, J., and Bottou, L. (2005).
Fast kernel classifiers with online and active learning.
Journal of Machine Learning Research, 6:1579–1619.
Breiman, L. (1996). Bagging predictors. Machine Learn-
ing, 24(2):123–140.
Breiman, L. (2001). Random forests. Machine Learning,
45(1):5–32.
Cortes, C. and Vapnik, V. (1995). Support-vector networks.
Machine learning, 20(3):273–297.
Degenhardt, F., Seifert, S., and Szymczak, S. (2017). Evalu-
ation of variable selection methods for random forests
and omics data sets. Briefings in bioinformatics, 20.
Dharani Y., G., Nair, N. G., Satpathy, P., and Christopher,
J. (2019). Covariate shift: A review and analysis on
classifiers. In 2019 GCAT, pages 1–6.
Ditzler, G., Roveri, M., Alippi, C., and Polikar, R. (2015).
Learning in nonstationary environments: A survey.
IEEE CIM, 10(4):12–25.
Domingos, P. and Hulten, G. (2002). Mining high-speed
data streams. Proceeding of the Sixth ACM SIGKDD.
Ducange, P., Marcelloni, F., and Pecori, R. (2021). Fuzzy
hoeffding decision tree for data stream classification.
Int. Journal of Comput. Intell. Systems, 14(1):946.
Elwell, R. and Polikar, R. (2011). Incremental learning
of concept drift in nonstationary environments. IEEE
Transactions on Neural Networks, 22(10):1517–1531.
Gomes, H. M., Bifet, A., Read, J., Barddal, J. P., En-
embreck, F., Pfharinger, B., Holmes, G., and Ab-
dessalem, T. (2017). Adaptive random forests for
evolving data stream classification. Machine Learn-
ing, 106(9-10):1469–1495.
Gunduz, N. and Fokoue, E. (2015). Robust classifica-
tion of high dimension low sample size data. arXiv:
1501.00592.
Hasanin, T., Khoshgoftaar, T. M., Leevy, J., and Seliya,
N. (2019). Investigating random undersampling and
feature selection on bioinformatics big data. In 2019
IEEE BigDataService, pages 346–356.
Hirsch, V., Reimann, P., and Mitschang, B. (2019). Data-
driven fault diagnosis in end-of-line testing of com-
plex products. In 2019 IEEE DSAA, pages 492–503.
Homayoun, S. and Ahmadzadeh, M. (2016). A review on
data stream classification approaches. Journal of Ad-
vanced Computer Science & Technology, 5(1):8.
Iwashita, A. S. and Papa, J. P. (2019). An overview on con-
cept drift learning. IEEE Access, 7:1532–1547.
John, G. H., Kohavi, R., and Pfleger, K. (1994). Irrelevant
features and the subset selection problem. In Cohen,
W. W. and Hirsh, H., editors, Machine Learning Pro-
ceedings 1994, pages 121–129. Morgan Kaufmann,
San Francisco (CA).
Khan, A. and Usman, M. (2015). Early diagnosis of
alzheimer’s disease using machine learning tech-
niques: A review paper. In 2015 IC3K, volume 01,
pages 380–387.
Khoshkangini, R., Pashami, S., and Nowaczyk, S. (2019).
Warranty claim rate prediction using logged vehicle
data. In Moura Oliveira, P., Novais, P., and Reis, L. P.,
editors, Progress in AI, pages 663–674.
Kull, M. and Flach, P. (2014). Patterns of dataset shift.
First International Workshop on Learning over Mul-
tiple Contexts (LMCE) at ECML-PKDD.
Losing, V., Hammer, B., and Wersing, H. (2018). Incremen-
tal on-line learning: A review and comparison of state
of the art algorithms. Neurocomputing, 275:1261–
1274.
Lu, J., Liu, A., Dong, F., Gu, F., Gama, J., and Zhang,
G. (2019). Learning under concept drift: A review.
IEEE Transactions on Knowledge and Data Engineer-
ing, 31(12):2346–2363.
Mait
´
ın, A. M., Garc
´
ıa-Tejedor, A. J., and Mu
˜
noz, J. P. R.
(2020). Machine learning approaches for detecting
parkinson’s disease from eeg analysis: A systematic
review. Applied Sciences, 10(23).
Marron, J., Todd, M., and Ahn, J. (2007). Distance-
weighted discrimination. Journal of the American Sta-
tistical Association, 102:1267–1271.
Moreno-Torres, J. G., Raeder, T., Alaiz-Rodr
´
ıguez, R.,
Chawla, N. V., and Herrera, F. (2012). A unifying
view on dataset shift in classification. Pattern Recog-
nition, 45(1):521–530.
Nalbach, O., Linn, C., Derouet, M., and Werth, D. (2018).
Predictive quality: Towards a new understanding of
quality assurance using machine learning tools. In
Business Information Systems, pages 30–42. Springer
International Publishing.
Pearson, K. (1901). LIII. on lines and planes of closest fit to
systems of points in space. The London, Edinburgh,
and Dublin Philosophical Magazine and Journal of
Science, 2(11):559–572.
Prytz, R., Nowaczyk, S., R
¨
ognvaldsson, T., and Byttner, S.
(2015). Predicting the need for vehicle compressor
repairs using maintenance records and logged vehi-
cle data. Engineering Applications of Artificial Intel-
ligence, 41:139–150.
Qui
˜
nonero-Candela, J., Sugiyama, M., Schwaighofer, A.,
and Lawrence, N. D., editors (2008). Dataset Shift in
Machine Learning. The MIT Press.
Turki, T. and Wei, Z. (2016). A greedy-based oversam-
pling approach to improve the prediction of mortality
in mers patients. In 2016 Annual IEEE Systems Con-
ference (SysCon), pages 1–5.
Utgoff, P. E. (1989). Incremental induction of decision
trees. Machine Learning, 4(2):161–186.
Wu, S. (2013). A review on coarse warranty data and anal-
ysis. Reliability Eng. & System Safety, 114:1–11.
Analysis of Incremental Learning and Windowing to Handle Combined Dataset Shifts on Binary Classification for Product Failure
Prediction
405