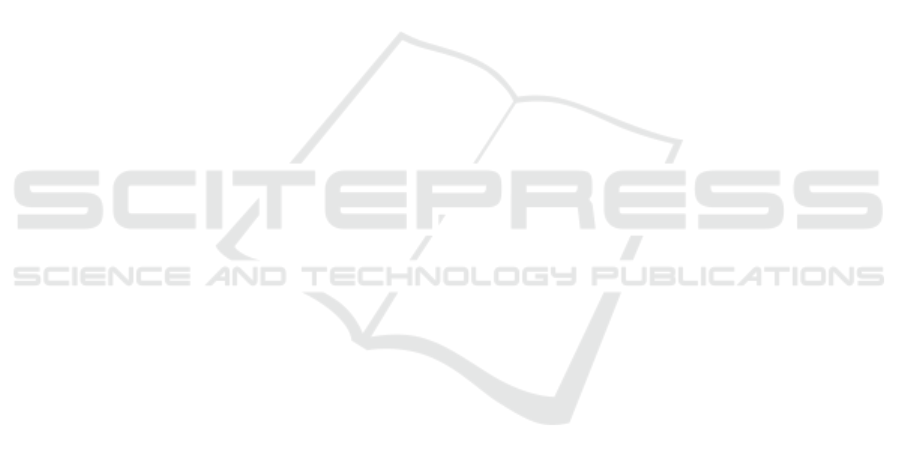
Further, new embedding types have been found
that behave well on specific dimensions, and further
study of these embeddings can be meaningful within
the framework of the semantic similarity problem.
REFERENCES
Arefyev, N., Ermolaev, P., and Panchenko, A. (2018).
How much does a word weigh? weighting word em-
beddings for word sense induction. arXiv preprint
arXiv:1805.09209.
Dhingra, B., Shallue, C. J., Norouzi, M., Dai, A. M., and
Dahl, G. E. (2018). Embedding text in hyperbolic
spaces. arXiv preprint arXiv:1806.04313.
Harris, Z. (1954). Distributional hypothesis. Word,
10(23):146–162.
Huang, G., Quo, C., Kusner, M. J., Sun, Y., Weinberger,
K. Q., and Sha, F. (2016). Supervised word mover’s
distance. In Proceedings of the 30th International
Conference on Neural Information Processing Sys-
tems, pages 4869–4877.
Kusner, M., Sun, Y., Kolkin, N., and Weinberger, K. (2015).
From word embeddings to document distances. In
International conference on machine learning, pages
957–966. PMLR.
Mikolov, T., Sutskever, I., Chen, K., Corrado, G. S., and
Dean, J. (2013). Distributed representations of words
and phrases and their compositionality. In Advances in
neural information processing systems, pages 3111–
3119.
Nickel, M. and Kiela, D. (2017). Poincar
´
e embeddings
for learning hierarchical representations. Advances
in neural information processing systems, 30:6338–
6347.
Papineni, K., Roukos, S., Ward, T., and Zhu, W.-J. (2002).
Bleu: a method for automatic evaluation of machine
translation. In Proceedings of the 40th annual meet-
ing of the Association for Computational Linguistics,
pages 311–318.
Pennington, J., Socher, R., and Manning, C. D. (2014).
Glove: Global vectors for word representation. In
Proceedings of the 2014 conference on empirical
methods in natural language processing (EMNLP),
pages 1532–1543.
Popovi
´
c, M. (2015). chrf: character n-gram f-score for au-
tomatic mt evaluation. In Proceedings of the Tenth
Workshop on Statistical Machine Translation, pages
392–395.
Sato, R., Yamada, M., and Kashima, H. (2021). Re-
evaluating word mover’s distance. arXiv preprint
arXiv:2105.14403.
Solomon, S., Cohn, A., Rosenblum, H., Hershkovitz, C.,
and Yamshchikov, I. P. (2021). Rethinking crowd
sourcing for semantic similarity. arXiv preprint
arXiv:2109.11969.
Sun, C., Ng, K. T. J., Henville, P., and Marchant, R. (2018).
Hierarchical word mover distance for collaboration
recommender system. In Australasian Conference on
Data Mining, pages 289–302. Springer.
Tifrea, A., Becigneul, G., and Ganea, O.-E. (2018).
Poincare glove: Hyperbolic word embeddings. In In-
ternational Conference on Learning Representations.
Volpi, R. and Malag
`
o, L. (2021). Natural alpha embeddings.
Information Geometry, pages 1–27.
Wang, Z., Zhou, D., Yang, M., Zhang, Y., Rao, C., and
Wu, H. (2020). Robust document distance with
wasserstein-fisher-rao metric. In Asian Conference on
Machine Learning, pages 721–736. PMLR.
Yamshchikov, I. P., Shibaev, V., Khlebnikov, N., and
Tikhonov, A. (2020). Style-transfer and paraphrase:
Looking for a sensible semantic similarity metric.
arXiv preprint arXiv:2004.05001.
Yokoi, S., Takahashi, R., Akama, R., Suzuki, J., and Inui,
K. (2020). Word rotator’s distance. arXiv preprint
arXiv:2004.15003.
Zhang, T., Kishore, V., Wu, F., Weinberger, K. Q., and
Artzi, Y. (2019). Bertscore: Evaluating text genera-
tion with bert. arXiv preprint arXiv:1904.09675.
Moving Other Way: Exploring Word Mover Distance Extensions
97