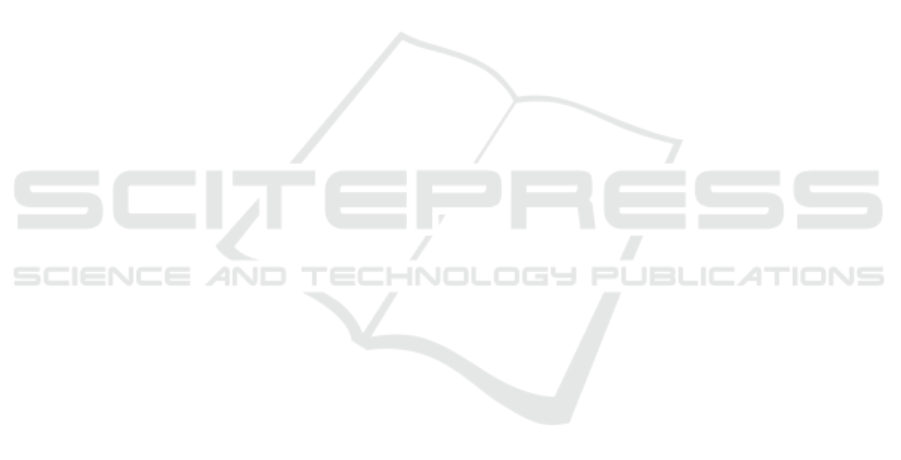
et al., 2012). In the review presented, we excluded
studies not written in English and this poses a lim-
itation on generalizing our results since in our data
retrieval and data analysis we found studies written in
other languages (i.e Russian). However, it is worth
noting that these studies written in Russian did not
meet the other inclusion/exclusion criterion as they
were analysed by the first author who is native a Rus-
sian language speaker.
6.4 Conclusion Validity
Similar to what is known as interpretive validity, con-
clusion validity is concerned with the formulation
of inaccurate conclusions from the given data. This
could be the small set of primary studies we have
collected. To mitigate the risk of excluding primary
studies we did the following: (i) the search strings
are defined by considering the concepts and their
acronyms, (ii) the related works of all the primary
studies are carefully analyzed, (iii) Duplicates were
removed based on the title, author, publisher, and year
of publication. The full text of selected studies was
carefully read through to mitigate misinterpretations
and extract accurate data.
7 CONCLUSIONS AND FURTHER
WORK
The goal of this study was to find the relationship be-
tween observed stress levels while programming and
while painting a picture. The relationship was aimed
to be recognised at the mental state level with the
help of EEG. To achieve the aforementioned goals,
we conducted a systematic literature review using
guidelines proposed by Kitchenham (Kitchenham and
Charters, 2007). We formulated four research ques-
tions and based on inclusion/exclusion criteria and
quality assessment we found 14 studies that were used
to answer the research questions. The review shows
that EEG devices can be used to accurately measure
stress. Secondly, the review revealed that there exists
a research gap and there is a need to conduct more ex-
periments for analysis of the stress level pattern when
a programmer is writing the code.
For future works, we plan on conducting experi-
ments whereby we will focus on the comparison be-
tween specific situations or activities that are done
while programming and done while painting accord-
ing to the pattern of the level of stress. In addition, we
aim at analysing a specific pattern of stress levels of
programmers during code writing in the experiments.
REFERENCES
Aljuaid, A. M. (2019). Theoretical design of eeg-based
neuroergonomics integrated portable system, apply-
ing direct psychophysiological indicators. In 2019 In-
dustrial & Systems Engineering Conference (ISEC),
pages 1–6. IEEE.
Amores, J., Richer, R., Zhao, N., Maes, P., and Eskofier,
B. M. (2018). Promoting relaxation using virtual re-
ality, olfactory interfaces and wearable eeg. In 2018
IEEE 15th international conference on wearable and
implantable body sensor networks (BSN), pages 98–
101. IEEE.
Andersson, M., Avamini, A., Colosimo, A., D’Amico, A.,
Davide, F., Di Natale, C., Ganci, S., Gutknecht, M.,
Holmberg, M., Mazzone, E., et al. (2001). Angelo
evaluation: application of a multisensor system for
psycho-physiological stress detection in working en-
vironments. In ICECS 2001. 8th IEEE International
Conference on Electronics, Circuits and Systems (Cat.
No. 01EX483), volume 3, pages 1509–1512. IEEE.
Chrousos (1998). Stressors, stress, and neuroendocrine in-
tegration of the adaptive response. the 1997 hans selye
memorial lecture. Annals of the New York Academy of
Sciences, 851:311–335.
Desai, A. R. (2017). Eeg-based evaluation of cognitive and
emotional arousal when coding in different program-
ming languages. University of Oulu.
Duraisingam, A., Palaniappan, R., and Andrews, S. (2017).
Cognitive task difficulty analysis using eeg and data
mining. In 2017 Conference on Emerging Devices and
Smart Systems (ICEDSS), pages 52–57. IEEE.
Hassan, I., Weyers, P., Maschuw, K., Dick, B., Gerdes,
B., Rothmund, M., and Zielke, A. (2006). Nega-
tive stress-coping strategies among novices in surgery
correlate with poor virtual laparoscopic performance.
Journal of British Surgery, 93(12):1554–1559.
Hetland, H., Sandal, G. M., and Johnsen, T. B. (2007).
Burnout in the information technology sector: Does
leadership matter? European Journal of Work and
Organizational Psychology, 16(1):58–75.
Hosseini, S. A. and Khalilzadeh, M. A. (2010). Emo-
tional stress recognition system using eeg and psy-
chophysiological signals: Using new labelling process
of eeg signals in emotional stress state. In 2010 Inter-
national Conference on Biomedical Engineering and
Computer Science, pages 1–6.
Hou, X., Liu, Y., Sourina, O., Tan, Y. R. E., Wang, L., and
Mueller-Wittig, W. (2015). Eeg based stress monitor-
ing. In 2015 IEEE International Conference on Sys-
tems, Man, and Cybernetics, pages 3110–3115.
Imperatori, C., Brunetti, R., Farina, B., Speranza,
A. M., Losurdo, A., Testani, E., Contardi, A.,
and Della Marca, G. (2014). Modification of eeg
power spectra and eeg connectivity in autobiograph-
ical memory: a sloreta study. Cognitive Processing,
15(3):351–361.
Imperatori, C., Farina, B., Adenzato, M., Valenti, E. M.,
Murgia, C., Marca, G. D., Brunetti, R., Fontana, E.,
and Ardito, R. B. (2019). Default mode network alter-
ations in individuals with high-trait-anxiety: An eeg
Review: Use of EEG on Measuring Stress Levels When Painting and Programming
379