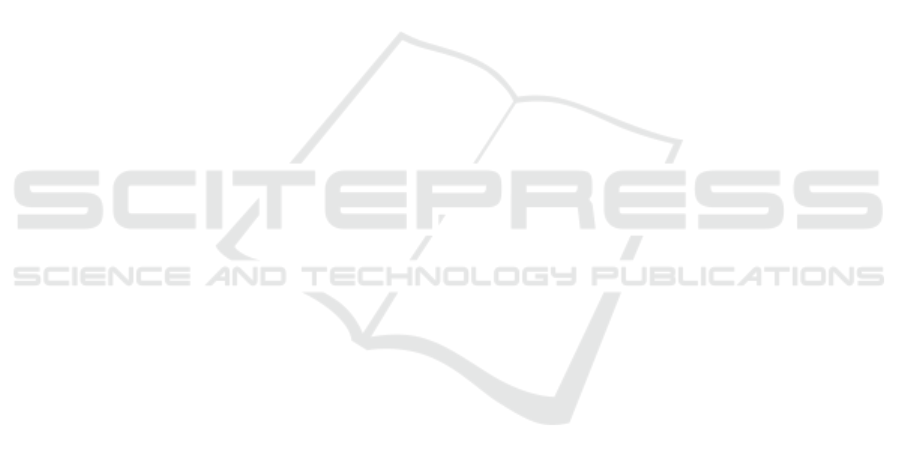
Kim Geok, T., Zar Aung, K., Sandar Aung, M., Thu Soe,
M., Abdaziz, A., Pao Liew, C., Hossain, F., Tso, C. P.,
and Yong, W. H. (2021). Review of indoor position-
ing: Radio wave technology. Appl. Sci., 11(1):279.
K
´
oczy, L. and Hirota, K. (1993). Approximate reasoning by
linear rule interpolation and general approximation.
Int. J. Approximate Reasoning, 9(3):197–225.
Kodinariya, T. M. and Makwana, P. R. (2013). Review on
determining number of cluster in k-means clustering.
Int. J. Adv. Res. Comput. Sci. Manage. Stud., 1(6):90–
95.
LeCun, Y., Bengio, Y., and Hinton, G. (2015). Deep learn-
ing. nature, 521(7553):436–444.
L
´
eger, J.-C. (1999). Menger curvature and rectifiability.
Ann. Math., 149:831–869.
Li, J., Qu, Y., Shum, H. P. H., and Yang, L. (2017). Tsk
inference with sparse rule bases. In Proc. Springer
Adv. Comput. Intell. Syst., pages 107–123.
Li, J., Shum, H. P., Fu, X., Sexton, G., and Yang, L. (2016).
Experience-based rule base generation and adaptation
for fuzzy interpolation. In Proc. IEEE Int. Conf. Fuzzy
Syst., pages 102–109.
Li, J., Yang, L., Fu, X., Chao, F., and Qu, Y. (2018a). Inter-
val type-2 tsk+ fuzzy inference system. In Proc. IEEE
Int. Conf. Fuzzy Syst., pages 1–8.
Li, J., Yang, L., Qu, Y., and Sexton, G. (2018b). An ex-
tended takagi–sugeno–kang inference system (tsk+)
with fuzzy interpolation and its rule base generation.
Soft Comput., 22(10):3155–3170.
Maduraga, M. W. P. and Abeysekara, R. (2021). Compar-
ison of supervised learning-based indoor localization
techniques for smart building applications. In Proc.
IEEE Int. Res. Conf. Smart Comput. Syst. Eng., vol-
ume 4, pages 145–148.
Mamdani, E. H. (1977). Application of fuzzy logic to ap-
proximate reasoning using linguistic synthesis. IEEE
Trans. Comput., 26(12):1182–1191.
Nessa, A., Adhikari, B., Hussain, F., and Fernando, X. N.
(2020). A survey of machine learning for indoor posi-
tioning. IEEE Access, 8:214945–214965.
Sthapit, P., Gang, H.-S., and Pyun, J.-Y. (2018). Bluetooth
based indoor positioning using machine learning al-
gorithms. In Proc. IEEE Int. Conf. Consum. Electron.
- Asia, pages 206–212.
Suroso, D. J., Rudianto, A. S., Arifin, M., and Hawibowo,
S. (2021). Random forest and interpolation techniques
for fingerprint-based indoor positioning system in un-
ideal environment. Int. J. Comput. Digital Syst.
Takagi, T. and Sugeno, M. (1985). Fuzzy identification of
systems and its applications to modeling and control.
” IEEE Trans. Syst., Man, and Cybern., 15(1):116–
132.
Yang, L., Zuo, Z., Chao, F., and Qu, Y. (2017). Fuzzy inter-
polation systems and applications. In Ramakrishnan,
S., editor, Modern Fuzzy Control Systems and Its Ap-
plications. IntechOpen, Rijeka.
Yang, W., Yuan, Y., Ren, W., Liu, J., Scheirer, W. J., Wang,
Z., Zhang, T., et al. (2020). Advancing image un-
derstanding in poor visibility environments: A collec-
tive benchmark study. IEEE Trans. Image Process.,
29:5737–5752.
Zhang, D. and Tan, C. (2021). Application of indoor po-
sitioning technology in smart home management sys-
tem. In Proc. IEEE Int. Conf. Big Data Artif. Intell.
Internet Things Eng., pages 627–631.
Zsolt T
´
oth, J. T. (2016). Miskolc iis hybrid ips: Dataset for
hybrid indoor positioning. In Proc. IEEE Int. Conf.
Radioelektronika, pages 408–412.
Zuo, Z., Chen, X., Xu, H., Li, J., Liao, W., Yang, Z.-X.,
and Wang, S. (2022). Idea-net: Adaptive dual self-
attention network for single image denoising. In Proc.
IEEE/CVF Winter Conf. Appl. of Comput. Vis. Work.,
pages 739–748.
Zuo, Z., Li, J., Anderson, P., Yang, L., and Naik, N. (2018).
Grooming detection using fuzzy-rough feature selec-
tion and text classification. In Proc. IEEE Int. Conf.
Fuzzy Syst., pages 1–8.
Zuo, Z., Li, J., Wei, B., Yang, L., Chao, F., and Naik, N.
(2019). Adaptive activation function generation for
artificial neural networks through fuzzy inference with
application in grooming text categorisation. In Proc.
IEEE Int. Conf. Fuzzy Syst., pages 1–6.
Zuo, Z., Li, J., Xu, H., and Moubayed, N. A. (2021).
Curvature-based feature selection with application in
classifying electronic health records. Elsevier Tech-
nol. Forecasting Social Change, 173:121–127.
Zuo, Z., Yang, L., Liu, Y., Chao, F., Song, R., and Qu, Y.
(2020). Histogram of fuzzy local spatio-temporal de-
scriptors for video action recognition. IEEE Trans.
Ind. Inf., 16(6):4059–4067.
IoTBDS 2022 - 7th International Conference on Internet of Things, Big Data and Security
288