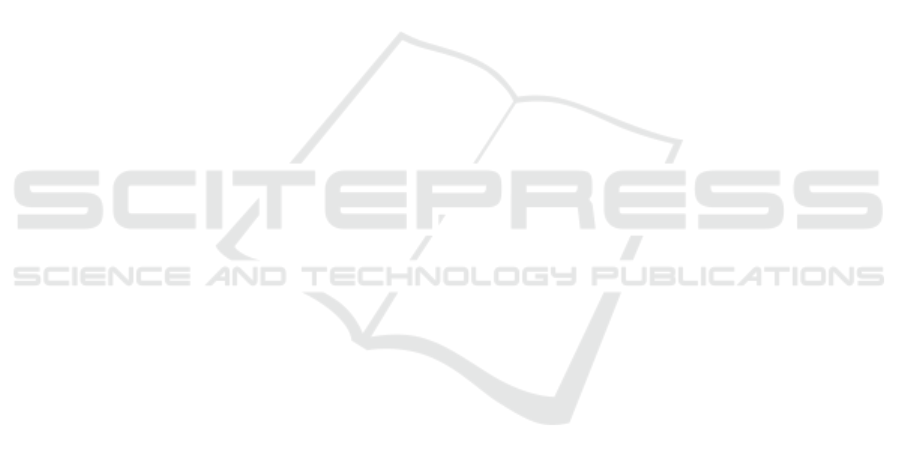
a sparsely populated area. An important aspect of this
project was to figure out the benefits of micro pub-
lic transport, where commuters share a bus with other
commuters to overcome the first mile to a train sta-
tion. Another focus was to find out to what extent
commuters’ travel times change using micro public
transport, which has a strong influence on user accep-
tance. The base goals of the project can be divided
into following sub-goals:
• Mathematical Modelling of the Optimization
Problem: A sub-goal of the project was the real-
istic mathematical modelling of the optimization
problem for the control of a ride service. The
problem is to pick up customers from any loca-
tion and bring them to a given destination, which
is a train station. The optimization task consists
in falling below a travel time that is acceptable for
the user under very dynamic conditions. These
conditions include different traffic situations and a
varying number of customers with different pick-
up points who are to be served by the system. The
departure times of the desired train line play a par-
ticularly important role, as these times are deci-
sive for when the commuters should arrive at the
station.
• Route Control: With the help of mathematical
modelling of the problem, an algorithm was de-
veloped that dynamically controls a vehicle fleet.
This vehicle fleet consists of several buses with
predefined capacities that are intended to satisfy
the various pick-up requests. The task of the al-
gorithm is to choose optimal routes and departure
times of the buses so that the optimization criteria
are met as good as possible.
• Traffic Simulation: Microscopic traffic simula-
tions are used to validate the developed control
algorithm. The focus is on determining the poten-
tial for saving carbon dioxide emissions and com-
paring travel times between individual transport
and a micro public transport system for different
scenarios.
Through the above-mentioned sub-goals, a system
was created with the goal of simulating an intelligent
micro public transport system. With the help of mi-
croscopic traffic simulations, scenarios were exam-
ined in which commuters get to their workplaces in-
dividually with their own vehicle and with the micro
public transport system. The mathematical modelling
of the optimization problem ensures that the routes of
the pick-up buses are optimally chosen so that their
total travel time is minimized and commuters’ indi-
vidual time constraints are satisfied. This has the ef-
fect of increasing commuter acceptance of a micro
public transport system. When designing the simu-
lations, the focus was placed on one train line per sce-
nario. This means that only those commuters who
want to catch the same train will be considered. It
is not possible for a pick-up bus to serve commuters
with different preferred train lines, as the different
train lines are usually quite far apart in relation to
the departure times and the commuters’ travel times
would therefore suffer as a result.
3 RELATED WORK
The ideal chemical reaction of fuel combustion cham-
ber produces carbon dioxide and water and, as a result
of the transient combustion process, additional com-
bustion products. Consequently, fuel design holds a
big potential for further improvements in reduction of
unwanted combustion products (
¨
Uberall et al., 2015).
However, as long as one is not willing to completely
change the method of transportation using internal
combustion engines, carbon dioxide (CO
2
) emission
is always unavoidable. As a consequence CO
2
takes
about 65% of the total greenhouse gas emissions,
where the transportation sector currently contributes
20 -25% of global CO
2
emissions with its global share
estimated to rise to 30-50% by 2050 (Yang et al.,
2019).
At first sight one might think that alternative fuel
vehicles such as all-electric and fuel cell vehicles will
be the best solution to reduce CO
2
emissions. These
fall into the category of cleaner vehicle strategies re-
ducing emission rates per vehicle-kilometre. How-
ever, according to (Litman, 2017), efficient and alter-
native fuel vehicles only provide a few benefits, and
by increasing total vehicle travel tend to exacerbate
problems such as congestion, accidents and sprawl.
Moreover one should be aware of the fact that for such
vehicles one has always to take into account the full
life time cycle (manufacturing, use of the vehicle, end
of life, and recycling) (Mar
´
ın and De Miguel Perales,
2021). Mobility management, i.e. strategies which
reduce total vehicle travel, provides far more benefits.
Eco-driving, a term used for driving assistance
techniques that support the driver in optimizing route
choice and driving behavior, is a cleaner vehicle strat-
egy which is suggested in (Engelmann et al., 2020). In
their study they incorporate a vehicle dynamic based
CO
2
emission model and a Pareto-optimal based rout-
ing approach and discuss the benefit trade-off be-
tween travel time and emission in a simulation study.
Similarly (Engelmann et al., 2020) discusses emission
optimized routes in terms of NO
x
using the Graph-
Hopper API and OpenStreetMap. In all evaluated
VEHITS 2022 - 8th International Conference on Vehicle Technology and Intelligent Transport Systems
144