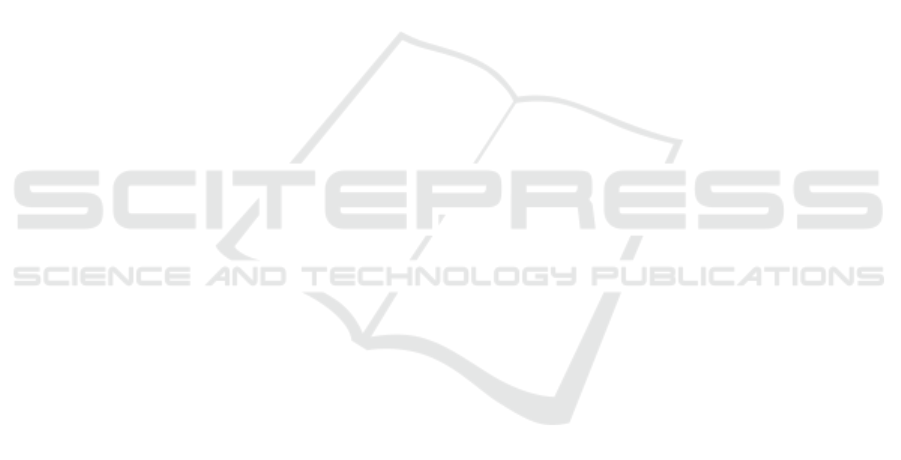
ACKNOWLEDGEMENTS
The authors thank Dr. Sridhar Kundur for many fruit-
ful discussion and suggestions that led to meaningful
improvements of this manuscript.
REFERENCES
Ache, J. M., Polsky, J., Alghailani, S., Parekh, R., Breads,
P., Peek, M. Y., Bock, D. D., von Reyn, C. R., and
Card, G. M. (2019). Neural basis for looming size and
velocity encoding in the drosophila giant fiber escape
pathway. Current Biology, 29(6):1073–1081.
Albus, J. S. and Hong, T. H. (1990). Motion, depth, and im-
age flow. In Proceedings., IEEE International Confer-
ence on Robotics and Automation, pages 1161–1170.
IEEE.
Aloimonos, Y. (1992). Is visual reconstruction necessary?
obstacle avoidance without passive ranging. Journal
of Robotic Systems, 9(6):843–858.
Evans, D. A., Stempel, A. V., Vale, R., Ruehle, S., Lefler,
Y., and Branco, T. (2018). A synaptic threshold
mechanism for computing escape decisions. Nature,
558(7711):590–594.
Geiger, A., Lenz, P., Stiller, C., and Urtasun, R. (2013).
Vision meets robotics: The kitti dataset. The Inter-
national Journal of Robotics Research, 32(11):1231–
1237.
Grigorescu, S., Trasnea, B., Cocias, T., and Macesanu,
G. (2020). A survey of deep learning techniques
for autonomous driving. Journal of Field Robotics,
37(3):362–386.
Kundur, S. R. and Raviv, D. (1999). Novel active vision-
based visual threat cue for autonomous navigation
tasks. Computer Vision and Image Understanding,
73(2):169–182.
Mujumdar, A. and Padhi, R. (2011). Evolving philoso-
phies on autonomous obstacle/collision avoidance of
unmanned aerial vehicles. Journal of Aerospace Com-
puting, Information, and Communication, 8(2):17–41.
Raviv, D. (1992). A quantitative approach to looming. US
Department of Commerce, National Institute of Stan-
dards and Technology.
Raviv, D. and Joarder, K. (2000). The visual looming nav-
igation cue: A unified approach. Comput. Vis. Image
Underst., 79:331–363.
Ridwan, I. (2018). Looming object detection with event-
based cameras. University of Lethbridge (Canada).
Roriz, R., Cabral, J., and Gomes, T. (2021). Automotive
lidar technology: A survey. IEEE Transactions on In-
telligent Transportation Systems.
Sharma, O., Sahoo, N. C., and Puhan, N. (2021). Recent
advances in motion and behavior planning techniques
for software architecture of autonomous vehicles: A
state-of-the-art survey. Engineering applications of
artificial intelligence, 101:104211.
Wang, M., Voos, H., and Su, D. (2018). Robust online ob-
stacle detection and tracking for collision-free navi-
gation of multirotor uavs in complex environments.
In 2018 15th International Conference on Control,
Automation, Robotics and Vision (ICARCV), pages
1228–1234. IEEE.
Yasin, J. N., Mohamed, S. A., Haghbayan, M.-H., Heikko-
nen, J., Tenhunen, H., and Plosila, J. (2020). Un-
manned aerial vehicles (uavs): Collision avoidance
systems and approaches. IEEE access, 8:105139–
105155.
Zhang, J. and Singh, S. (2014). Loam: Lidar odometry
and mapping in real-time. In Robotics: Science and
Systems, volume 2, pages 1–9. Berkeley, CA.
VEHITS 2022 - 8th International Conference on Vehicle Technology and Intelligent Transport Systems
462