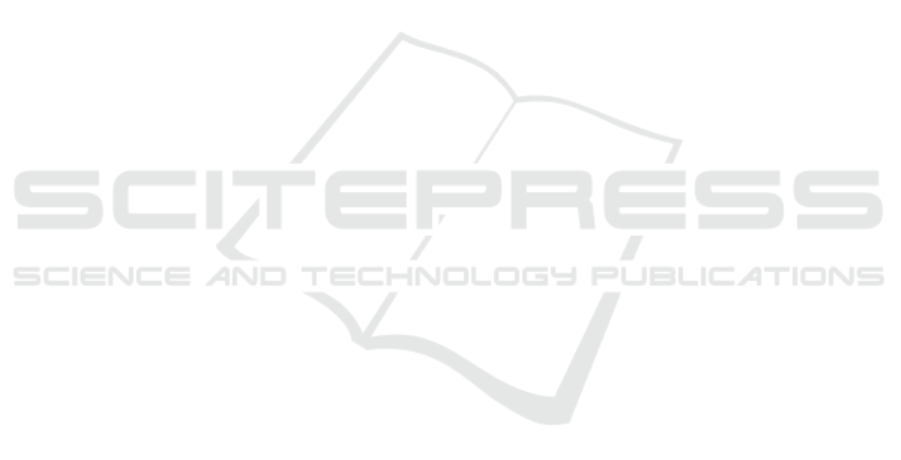
ference on Computer Vision and Pattern Recognition
(CVPR), pages 770–778. HugoFerreira.
Ioffe, S. and Szegedy, C. (2015). Batch normalization:
Accelerating deep network training by reducing in-
ternal covariate shift. In Bach, F. and Blei, D., ed-
itors, Proceedings of the 32nd International Confer-
ence on Machine Learning, volume 37 of Proceedings
of Machine Learning Research, pages 448–456, Lille,
France. PMLR.
Jack, C. R., Knopman, D. S., Jagust, W. J., Shaw, L. M.,
Aisen, P. S., Weiner, M. W., Petersen, R. C., and
Trojanowski, J. Q. (2010). Hypothetical model of
dynamic biomarkers of the Alzheimer’s pathological
cascade. The Lancet. Neurology, 9(1):119–28.
Jha, D., Smedsrud, P. H., Riegler, M. A., Johansen, D.,
Lange, T. d., Halvorsen, P., and Johansen, H. D.
(2019). ResUNet++: An advanced architecture for
medical image segmentation. In Proceedings - 2019
IEEE International Symposium on Multimedia, ISM
2019, volume 00, pages 225–230. Institute of Electri-
cal and Electronics Engineers Inc.
Kingma, D. P. and Ba, J. (2015). Adam: A method for
stochastic optimization. In Bengio, Y. and LeCun,
Y., editors, 3rd International Conference on Learn-
ing Representations, ICLR 2015, San Diego, CA, USA,
May 7-9, 2015, Conference Track Proceedings.
Kitazawa, M., Medeiros, R., and LaFerla, F. M. (2012).
Transgenic mouse models of Alzheimer disease: De-
veloping a better model as a tool for therapeu-
tic interventions. Current Pharmaceutical Design,
18(8):1131–1147.
Koozekanani, D., Boyer, K., and Roberts, C. (2021). Reti-
nal thickness measurements from optical coherence
tomography using a Markov boundary model. IEEE
Transactions on Medical Imaging, 20(9):900–916.
Krantic, S. and Torriglia, A. (2014). Retina: source of the
earliest biomarkers for Alzheimer’s disease? Journal
of Alzheimer’s disease : JAD, 40(2):237–43.
London, A., Benhar, I., and Schwartz, M. (2013). The retina
as a window to the brain–from eye research to CNS
disorders. Nature Reviews Neurology, 9(1):44–53.
Morales, S., Colomer, A., Mossi, J. M., Amor, R. d., Wold-
bye, D., Klemp, K., Larsen, M., and Naranjo, V.
(2021). Retinal layer segmentation in rodent OCT im-
ages: Local intensity profiles & fully convolutional
neural networks. Computer Methods and Programs in
Biomedicine, 198:105788.
Morgia, C. L., Ross-Cisneros, F. N., Koronyo, Y., Hanni-
bal, J., Gallassi, R., Cantalupo, G., Sambati, L., Pan,
B. X., Tozer, K. R., Barboni, P., Provini, F., Avanzini,
P., Carbonelli, M., Pelosi, A., Chui, H., Liguori, R.,
Baruzzi, A., Koronyo-Hamaoui, M., Sadun, A. A.,
and Carelli, V. (2016). Melanopsin retinal ganglion
cell loss in Alzheimer disease. Annals of Neurology,
79(1):90–109.
Ngo, L., Cha, J., and Han, J.-H. (2020). Deep neural net-
work regression for automated retinal layer segmenta-
tion in optical coherence tomography images. IEEE
Transactions on Image Processing, 29:303–312.
Nunes, A., Silva, G., Duque, C., Janu
´
ario, C., Santana, I.,
Ambr
´
osio, A. F., Castelo-Branco, M., and Bernardes,
R. (2019). Retinal texture biomarkers may help to
discriminate between Alzheimer’s, Parkinson’s, and
healthy controls. PLOS ONE, 14(6):e0218826.
Oddo, S., Caccamo, A., Shepherd, J. D., Murphy, M.,
Golde, T. E., Kayed, R., Metherate, R., Mattson,
M. P., Akbari, Y., and LaFerla, F. M. (2003). Triple-
transgenic model of Alzheimer’s disease with plaques
and tangles intracellular aβ and synaptic dysfunction.
Neuron, 39(3):409–421.
Rathke, F., Schmidt, S., and Schn
¨
orr, C. (2014). Probabilis-
tic intra-retinal layer segmentation in 3-D OCT im-
ages using global shape regularization. Medical Image
Analysis, 18(5):781–794.
Ronneberger, O., Fischer, P., and Brox, T. (2015). U-
Net: Convolutional networks for biomedical image
segmentation. In Lecture Notes in Computer Science
(including subseries Lecture Notes in Artificial Intel-
ligence and Lecture Notes in Bioinformatics), volume
9351, pages 234–241. Springer Verlag.
Roy, A. G., Conjeti, S., Karri, S. P. K., Sheet, D., Katouzian,
A., Wachinger, C., and Navab, N. (2017). ReLayNet:
retinal layer and fluid segmentation of macular optical
coherence tomography using fully convolutional net-
works. Biomedical Optics Express, 8(8):3627.
Sarhan, M. H., Nasseri, M. A., Zapp, D., Maier, M.,
Lohmann, C. P., Navab, N., and Eslami, A. (2020).
Machine learning techniques for ophthalmic data pro-
cessing: A review. IEEE Journal of Biomedical and
Health Informatics, 24(12):3338–3350.
Sch
¨
on, C., Hoffmann, N. A., Ochs, S. M., Burgold, S.,
Filser, S., Steinbach, S., Seeliger, M. W., Arzberger,
T., Goedert, M., Kretzschmar, H. A., Schmidt, B., and
Herms, J. (2012). Long-term in vivo imaging of fib-
rillar tau in the retina of P301S transgenic mice. PLoS
ONE, 7(12):e53547.
Soh, L.-K. and Tsatsoulis, C. (1999). Texture analysis of
SAR sea ice imagery using gray level co-occurrence
matrices. IEEE Transactions on Geoscience and Re-
mote Sensing, 37(2):780–795.
Soukup, P., Maloca, P., Altmann, B., Festag, M., Atzpodien,
E.-A., and Pot, S. (2019). Interspecies variation of
outer retina and choriocapillaris imaged with optical
coherence tomography. Investigative Opthalmology &
Visual Science, 60(10):3332.
Srinivasan, P. P., Heflin, S. J., Izatt, J. A., Arshavsky, V. Y.,
and Farsiu, S. (2014). Automatic segmentation of
up to ten layer boundaries in SD-OCT images of the
mouse retina with and without missing layers due to
pathology. Biomedical Optics Express, 5(2):348.
Venhuizen, F. G., Ginneken, B. v., Liefers, B., Grinsven,
M. J. J. P. v., Fauser, S., Hoyng, C., Theelen, T., and
S
´
anchez, C. I. (2017). Robust total retina thickness
segmentation in optical coherence tomography images
using convolutional neural networks. Biomedical Op-
tics Express, 8(7):3292.
Yazdanpanah, A., Hamarneh, G., Smith, B. R., and Sarunic,
M. V. (2011). Segmentation of intra-retinal layers
from optical coherence tomography images using an
active contour approach. IEEE Transactions on Med-
ical Imaging, 30(2):484–496.
Shedding Light on Early Central Nervous System Changes for Alzheimer’s Disease through the Retina: An Animal Study
257