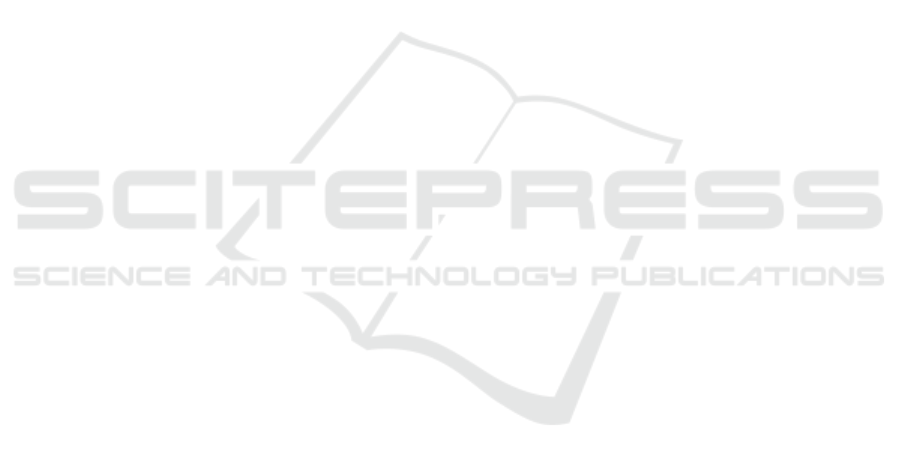
different nature of data, such as the time-series.
ACKNOWLEDGEMENTS
This research is partially funded and supported by:
project “Studio per l’adeguamento di aree portale
per tematismo - BRIC INAIL 2019 - FENU” CUP
F24G20000100001”; “PON R&I 2014-2020 Action
IV.6 - CUP F25F21002270003”.
REFERENCES
Carta, S., Fenu, G., Ferreira, A., Recupero, D. R., and
Saia, R. (2019). A two-step feature space transform-
ing method to improve credit scoring performance. In
International Joint Conference on Knowledge Discov-
ery, Knowledge Engineering, and Knowledge Man-
agement, pages 134–157. Springer.
Carta, S., Ferreira, A., Recupero, D. R., Saia, M., and Saia,
R. (2020). A combined entropy-based approach for a
proactive credit scoring. Engineering Applications of
Artificial Intelligence, 87:103292.
Carta, S., Ferreira, A., Recupero, D. R., and Saia, R. (2021).
Credit scoring by leveraging an ensemble stochastic
criterion in a transformed feature space. Progress in
Artificial Intelligence, pages 1–16.
Dumitrescu, E., Hue, S., Hurlin, C., and Tokpavi, S.
(2022). Machine learning for credit scoring: Improv-
ing logistic regression with non-linear decision-tree
effects. European Journal of Operational Research,
297(3):1178–1192.
Green, D. M. and Swets, J. A. (1966). Signal Detection
Theory and Psychophysics. Wiley, New York.
Hawkins, D. M. (2004). The problem of overfitting. Jour-
nal of chemical information and computer sciences,
44(1):1–12.
Khemais, Z., Nesrine, D., Mohamed, M., et al. (2016).
Credit scoring and default risk prediction: A compar-
ative study between discriminant analysis & logistic
regression. International Journal of Economics and
Finance, 8(4):39.
Leevy, J. L., Khoshgoftaar, T. M., Bauder, R. A., and Seliya,
N. (2018). A survey on addressing high-class imbal-
ance in big data. Journal of Big Data, 5(1):42.
Lei, K., Xie, Y., Zhong, S., Dai, J., Yang, M., and Shen,
Y. (2019). Generative adversarial fusion network for
class imbalance credit scoring. Neural Computing and
Applications, pages 1–12.
Liu, C., Huang, H., and Lu, S. (2019). Research on personal
credit scoring model based on artificial intelligence. In
International Conference on Application of Intelligent
Systems in Multi-modal Information Analytics, pages
466–473. Springer.
Liu, W., Fan, H., and Xia, M. (2022). Credit scoring based
on tree-enhanced gradient boosting decision trees. Ex-
pert Systems with Applications, 189:116034.
Park, S. and Park, H. (2021). Combined oversampling
and undersampling method based on slow-start al-
gorithm for imbalanced network traffic. Computing,
103(3):401–424.
Roy, A. G. and Urolagin, S. (2019). Credit risk assess-
ment using decision tree and support vector machine
based data analytics. In Creative Business and So-
cial Innovations for a Sustainable Future, pages 79–
84. Springer.
Saia, R. and Carta, S. (2016a). An entropy based algorithm
for credit scoring. In International Conference on Re-
search and Practical Issues of Enterprise Information
Systems, pages 263–276. Springer.
Saia, R. and Carta, S. (2016b). Introducing a vector space
model to perform a proactive credit scoring. In In-
ternational Joint Conference on Knowledge Discov-
ery, Knowledge Engineering, and Knowledge Man-
agement, pages 125–148. Springer.
Saia, R. and Carta, S. (2016c). A linear-dependence-based
approach to design proactive credit scoring models. In
KDIR, pages 111–120.
Saia, R. and Carta, S. (2017a). Evaluating credit card trans-
actions in the frequency domain for a proactive fraud
detection approach. In SECRYPT, pages 335–342.
Saia, R. and Carta, S. (2017b). A fourier spectral pattern
analysis to design credit scoring models. In Proceed-
ings of the 1st International Conference on Internet of
Things and Machine Learning, page 18. ACM.
Saia, R., Carta, S., and Fenu, G. (2018). A wavelet-based
data analysis to credit scoring. In Proceedings of the
2nd International Conference on Digital Signal Pro-
cessing, pages 176–180. ACM.
Saia, R., Carta, S., Recupero, D. R., Fenu, G., and Stan-
ciu, M. (2019). A discretized extended feature space
(defs) model to improve the anomaly detection per-
formance in network intrusion detection systems. In
KDIR, pages 322–329.
Saia., R., Giuliani., A., Pompianu., L., and Carta., S. (2021).
From payment services directive 2 (psd2) to credit
scoring: A case study on an italian banking institution.
In Proceedings of the 13th International Joint Confer-
ence on Knowledge Discovery, Knowledge Engineer-
ing and Knowledge Management - KDIR,, pages 164–
171. INSTICC, SciTePress.
Shen, F., Zhao, X., Kou, G., and Alsaadi, F. E. (2021).
A new deep learning ensemble credit risk evaluation
model with an improved synthetic minority oversam-
pling technique. Applied Soft Computing, 98:106852.
Sloan, R. H. and Warner, R. (2018). When is an algorithm
transparent? predictive analytics, privacy, and public
policy. IEEE Security & Privacy, 16(3):18–25.
Weiss, G. M. (2004). Mining with rarity: A unifying frame-
work. SIGKDD Explor. Newsl., 6(1):7–19.
Zhang, W., He, H., and Zhang, S. (2019). A novel multi-
stage hybrid model with enhanced multi-population
niche genetic algorithm: An application in credit scor-
ing. Expert Systems with Applications, 121:221–232.
SECRYPT 2022 - 19th International Conference on Security and Cryptography
282