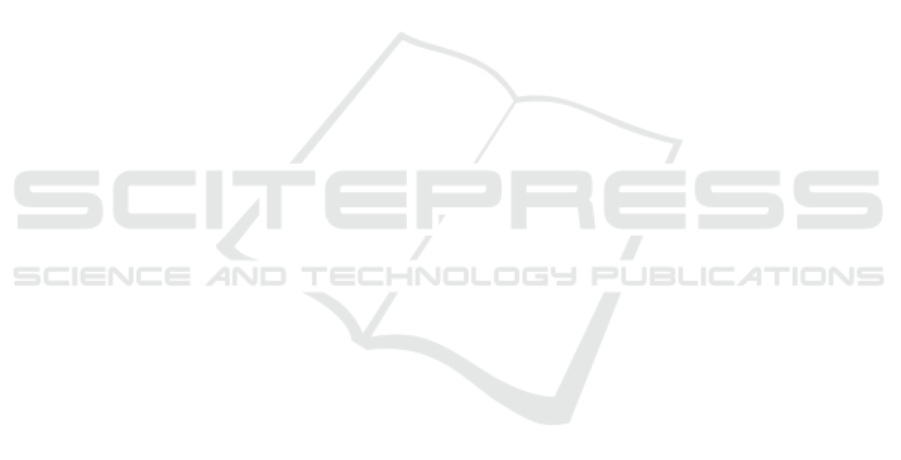
Benetos, E., Klapuri, A., and Dixon, S. (2012). Score-
informed transcription for automatic piano tutoring.
In 2012 Proceedings of the 20th European Signal Pro-
cessing Conference (EUSIPCO), pages 2153–2157.
Bernays, M. and Traube, C. (2013). Expressive produc-
tion of piano timbre: Touch and playing techniques
for timbre control in piano performance.
Br
´
ee, M. (1905). The groundwork of the Leschetizky
method. G. Schirmer.
Busse, W. G. (2002). Toward objective measurement and
evaluation of jazz piano performance via midi-based
groove quantize templates. Music Perception:An In-
terdisciplinary Journal, 19(3):443–461.
DeCheveigne, A., . K. H. (2002). Yin, a fundamental fre-
quency estimator for speech and music. The Journal of
the Acoustical Society of America, 111(4):1917–1930.
Dixon, S. (2001). Automatic extraction of tempo and beat
from expressive performances. Journal of New Music
Research, 30(1):39–58.
Emiya, V., Bertin, N., David, B., and Badeau, R. (2010).
MAPS - A piano database for multipitch estimation
and automatic transcription of music. Research report.
Eremenko V, Morsi A, N. J. S. X. (2020). Performance
assessment technologies for the support of musical in-
strument learning.
Gal, Y., Islam, R., and Ghahramani, Z. (2017). Deep
bayesian active learning with image data. In Interna-
tional Conference on Machine Learning, pages 1183–
1192. PMLR.
Goebl, W. (2001). Melody lead in piano performance: ex-
pressive device or artifact? The Journal of the Acous-
tical Society of America, 110(1):563–72.
Grachten, M. and Widmer, G. (2012). Linear basis mod-
els for prediction and analysis of musical expression.
Journal of New Music Research, 41(4):311–322.
Grosche, P. and M
¨
uller, M. (2010). What Makes
Beat Tracking Difficult? A Case Study on Chopin
Mazurkas. In in Proceedings of the 11th International
Society for Music Information Retrieval Conference,
2010, pages 649–654.
Hamond, LF; Welch, G. H. E. (2019). The pedagogical use
of visual feedback for enhancing dynamics in higher
education piano learning and performance. OPUS,
25(3):581–601.
Hattie, J. and Timperley, H. (2007). The power of feedback.
Review of Educational Research, 77(1):81–112.
Jianan, Y. (2021). Automatic evaluation system for piano
performance based on the internet of things technol-
ogy under the background of artificial intelligence.
Mathematical Problems in Engineering, 2021:14.
Johnson, D., Damian, D., and Tzanetakis, G. (2020). De-
tecting hand posture in piano playing using depth data.
Computer Music Journal, 43(1):59–78.
Kim, S., P. J. M. R. S. N. J. . L. K. (2021). Quantitative
analysis of piano performance proficiency focusing on
difference between hands. PloS one, 16(5).
Kim, S., Park, J. M., Rhyu, S., Nam, J., and Lee, K.
(2021). Quantitative analysis of piano performance
proficiency focusing on difference between hands.
PloS one, 16(5):e0250299.
Kosta, K., O. F. B. E. C. (2016). Outliers in performed
loudness transitions: An analysis of chopin mazurka
recordings. In International Conference for Music
Perception and Cognition (ICMPC), pages 601–604,
California, USA.
Lerch, A., A. C. P. A. and Gururani, S. (2020). An in-
terdisciplinary review of music performance analysis.
Transactions of the International Society for Music In-
formation Retrieval, 3(1):221–245.
Masaki, M., Hechler, P., Gadbois, S., and Waddell, G.
(2011). Piano performance assessment: video feed-
back and the quality assessment in music performance
inventory (qampi). In International Symposium on
Performance Science 2011, pages 24–27, Canada.
Mauch, M. and Dixon, S. (2014). Pyin: A fundamental
frequency estimator using probabilistic threshold dis-
tributions. In 2014 IEEE International Conference on
Acoustics, Speech and Signal Processing (ICASSP),
pages 659–663.
M
¨
uller, M. (2007). Information Retrieval for Music and
Motion, chapter Dynamic Time Warping. Springer
Berlin Heidelberg, Berlin, Heidelberg.
Nakamura, E., Ono, N., and Sagayama, S. (2014). Merged-
output hmm for piano fingering of both hands. In
Proceedings of the 13th International Society for Mu-
sic Information Retrieval Conference, ISMIR 2014,
pages 531–536, Taipei.
Nakamura, E., Saito, Y., and Yoshii, K. (2020). Statistical
learning and estimation of piano fingering. Informa-
tion Sciences, 517:68–85.
Neuhaus, H. (2008). The art of piano playing. Kahn and
Averill.
Nieto, A. (1988). La digitaci
´
on pian
´
ıstica. Fundaci
´
on
Banco Exterior.
Pan, J., Li, M., Song, Z., Li, X., Liu, X., Yi, H., and Zhu,
M. (2017). An Audio Based Piano Performance Eval-
uation Method Using Deep Neural Network Based
Acoustic Modeling. In Proc. Interspeech 2017, pages
3088–3092.
Parmar, P., Reddy, J., and Morris, B. (2021). Piano skills
assessment.
Parncutt, R., Sloboda, J. A., Clarke, E. F., Raekallio, M.,
and Desain, P. (1997). An Ergonomie Model of
Keyboard Fingering for Melodic Fragments Sibelius
Academy of Music , Helsinski. Music perception: An
Interdisciplinary Journal, 14(4):341–382.
Payeur, P., Nascimento, G. M. G., Beacon, J., Comeau, G.,
Cretu, A.-M., D’Aoust, V., and Charpentier, M.-A.
(2014). Human gesture quantification: An evaluation
tool for somatic training and piano performance. In
2014 IEEE International Symposium on Haptic, Au-
dio and Visual Environments and Games (HAVE) Pro-
ceedings, pages 100–105. IEEE.
Phanichraksaphong, V. and Tsai, W.-H. (2021). Automatic
evaluation of piano performances for steam education.
Applied Sciences, 11(24).
Ramoneda, P., Tamer, N. C., Eremenko, V., Miron, M., and
Serra, X. (2022). Score difficulty analysis for piano
performance education. In ICASSP 2022 IEEE Inter-
An Overview of Automatic Piano Performance Assessment within the Music Education Context
473