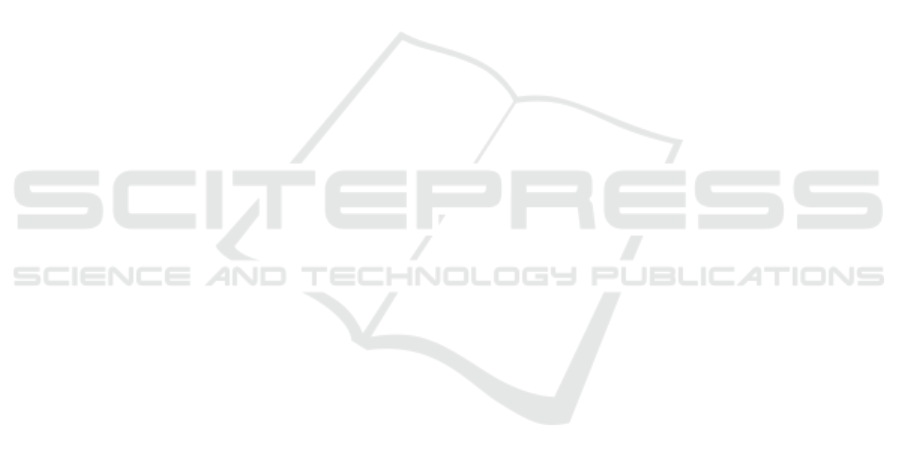
timate the Z-translation. In comparison to the state-
of-the-art, the proposed method achieves comparable
results while not using any artificial calibration tar-
gets. This makes the method versatile and applicable
in search and rescue scenarios.
In the future, one goal will be the reduction of
erroneous plane extractions which add inconclusive
constraints to the optimization process. Reducing
such mismatches directly improves the parameter es-
timation and also has a positive effect on the repeat-
able accuracy. Additional error modeling of the plane
extraction process will also improve uncertainty esti-
mation. Furthermore, using bins not only for azimuth
and elevation but also for the distance of the plane-
line-matches could be beneficial for the optimization
result.
ACKNOWLEDGEMENTS
This work has partly been funded by the German Fed-
eral Ministry of Education and Research (BMBF) un-
der the project number 13N15550 (UAV-Rescue).
REFERENCES
Domhof, J., Kooij, J. F., and Gavrila, D. M. (2021). A
Joint Extrinsic Calibration Tool for Radar, Camera
and Lidar. IEEE Transactions on Intelligent Vehicles,
6(3):571–582.
Domhof, J., Kooij, J. F. P., and Gavrila, D. M. (2019). A
multi-sensor extrinsic calibration tool for lidar , cam-
era and radar. IEEE International Conference on
Robotics and Automation (ICRA), pages 1–7.
El Natour, G., Ait-Aider, O., Rouveure, R., Berry, F., and
Faure, P. (2015). Toward 3D reconstruction of outdoor
scenes using an MMW radar and a monocular vision
sensor. Sensors (Switzerland), 15(10):25937–25967.
Fan, H., Bennetts, V. H., Schaffernicht, E., and Lilienthal,
A. J. (2019). Towards gas discrimination and mapping
in emergency response scenarios using a mobile robot
with an electronic nose. Sensors (Switzerland), 19(3).
Fischler, M. a. and Bolles, R. C. (1981). Random Sample
Consensus: A Paradigm for Model Fitting with Ap-
plications to Image Analysis and Automated Cartog-
raphy. Communications of the ACM, 24(6):381–395.
Fritsche, P., Kueppers, S., Briese, G., and Wagner, B.
(2016). Radar and LiDAR Sensorfusion in Low Vis-
ibility Environments. In Proceedings of the 13th In-
ternational Conference on Informatics in Control, Au-
tomation and Robotics (ICINCO), volume 2, pages
30–36. SciTePress.
Fritsche, P., Zeise, B., Hemme, P., and Wagner, B. (2017).
Fusion of radar, LiDAR and thermal information for
hazard detection in low visibility environments. SSRR
2017 - 15th IEEE International Symposium on Safety,
Security and Rescue Robotics, Conference, pages 96–
101.
Heng, L. (2020). Automatic targetless extrinsic calibration
of multiple 3D LiDARs and radars. In IEEE Interna-
tional Conference on Intelligent Robots and Systems.
Keel, B. M. (2010). Constant False Alarm Rate Detectors.
In Richards, M. A., Scheer, J. A., and Holm, W. A.,
editors, Principles of Modern Radar: Basic princi-
ples, chapter 16, pages 589–621. Institution of Engi-
neering and Technology, Edison, NJ.
Kim, J. H., Starr, J. W., and Lattimer, B. Y. (2015). Fire-
fighting Robot Stereo Infrared Vision and Radar Sen-
sor Fusion for Imaging through Smoke. Fire Technol-
ogy, 51(4):823–845.
Kummerle, R., Grisetti, G., Strasdat, H., Konolige, K.,
and Burgard, W. (2011). G2o: A general framework
for graph optimization. In 2011 IEEE International
Conference on Robotics and Automation, pages 3607–
3613. IEEE.
Per
ˇ
si
´
c, J., Markovi
´
c, I., and Petrovi
´
c, I. (2017). Extrinsic
6DoF calibration of 3D LiDAR and radar. 2017 Euro-
pean Conference on Mobile Robots, ECMR 2017.
Per
ˇ
si
´
c, J., Markovi
´
c, I., and Petrovi
´
c, I. (2019). Extrinsic
6DoF calibration of a radar–LiDAR–camera system
enhanced by radar cross section estimates evaluation.
Robotics and Autonomous Systems, 114:217–230.
Per
ˇ
si
´
c, J., Petrovi
´
c, L., Markovi
´
c, I., and Petrovi
´
c, I. (2021).
Online multi-sensor calibration based on moving ob-
ject tracking. Advanced Robotics, 35(3-4):130–140.
Scholler, C., Schnettler, M., Krammer, A., Hinz, G.,
Bakovic, M., Guzet, M., and Knoll, A. (2019). Target-
less Rotational Auto-Calibration of Radar and Cam-
era for Intelligent Transportation Systems. In 2019
IEEE Intelligent Transportation Systems Conference
(ITSC), pages 3934–3941. IEEE.
Sugimoto, S., Tateda, H., Takahashi, H., and Okutomi,
M. (2004). Obstacle detection using millimeter-
wave radar and its visualization on image sequence.
Proceedings - International Conference on Pattern
Recognition, 3(May):342–345.
Wang, T., Zheng, N., Xin, J., and Ma, Z. (2011). Integrating
millimeter wave radar with a monocular vision sensor
for on-road obstacle detection applications. Sensors,
11(9):8992–9008.
Wise, E., Persic, J., Grebe, C., Petrovic, I., and Kelly, J.
(2021). A Continuous-Time Approach for 3D Radar-
to-Camera Extrinsic Calibration. In 2021 IEEE In-
ternational Conference on Robotics and Automation
(ICRA), pages 13164–13170. IEEE.
ICINCO 2022 - 19th International Conference on Informatics in Control, Automation and Robotics
384